The road to perfection in medical image segmentation process
By: Graylight Imaging MedTeam
In our previous posts, we covered the topic of the use of 3D modelling and medical image segmentation in cardiovascular imaging. We believe that showing our experience with these processes, presenting examples of the application of 3D modelling and 3D printing in the cardiovascular field and a brief historical outline of medical image processing have helped to illustrate the enormous possibilities hidden within those processes.
Medical image segmentation – recap
We have been segmenting anatomical structures in the field of cardiology for over three years. In our experience, segmentation of the coronary arteries is the most labor-intensive.
These difficulties arise from: complicated shape of vessels; variability of vessels in patients – especially when anomalies are present; small vessel diameter in relation to spatial resolution of the scan; blurring and noise of the image resulting mainly from heart movements or technical parameters of the scan used during image acquisition by the centers from which the data are obtained.
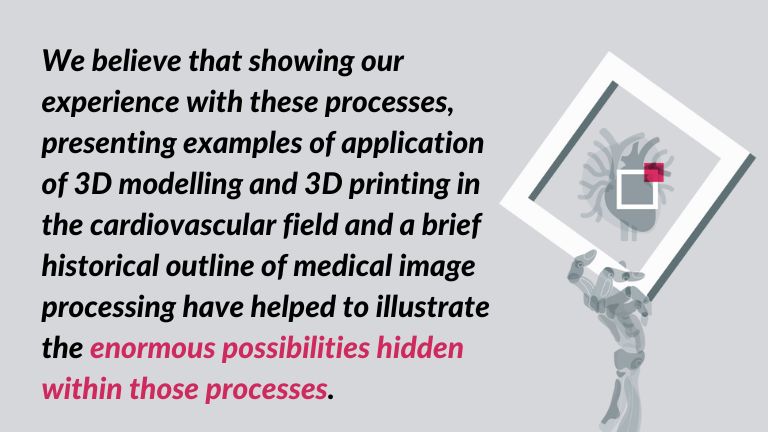
Practice makes perfect
Over the course of several years of working with the issue, we have been able to develop measures in the process that have had a positive impact on reducing the time spent on the task and increasing the quality of the segmentation itself.
Team competence
It is said that a competent team is the key to success. This universal truth also applies to such a basic and repeatable process as medical image segmentation. An employee with a background in medicine and technology will find it easier to identify the right anatomical areas and will be more proficient in using 2D and 3D segmentation tools – compared to someone with a different background. A person with domain-specific education will also seek to optimize the process. And that’s by drawing on previous experience. Our team includes specialists in biomedical engineering and electro-radiology, which works out great for the project.
Anatomy is for the people
Let’s face it, there is no fooling oneself that someone without a medical degree will be familiar with the anatomical details of all human organs. In our experience, it is well worth taking the time to familiarize yourself with the anatomy of a structure and its representation in medical images. Figuratively speaking – when the brain knows what it is looking for, it will find it more easily. It may also be a good idea to ask for help from a medical expert, such as a radiologist. He/she will tell you what to pay attention to and how not to fall into the anatomical traps in the case of anomalies.
Select and learn the tools
When there are a few thousand segmentations to make, it is advisable, at the very beginning, to think about the optimization of work. Before choosing a tool, dedicate some time to reviewing the software available on the market. And test the one that will allow us to work most efficiently. Perhaps the software available on the market will work well. Or maybe, it’s better to create one’s own tool for medical image segmentation tailored to one’s needs – as it was in our case.
It’s not just software that is important. Hardware also plays an essential role. Therefore, it is worth equipping yourself with an efficient computer, a monitor (or two) with appropriate parameters, as well as a comfortable mouse or even a 3D manipulator or a touchscreen graphic tablet. Before starting work, it is also worth getting acquainted with all the possibilities of software or hardware. We know from our own experience that a tool discovered by accident can make work much easier. When investing in a solution, let’s get to know it to the bone.
Assumptions in the medical image segmentation process
Consistency of models will be important, especially when we are creating segmentations that will provide input to machine learning. It is not always easy to determine where a given structure begins and where it ends or what level of detail we want to obtain. That’s why it’s a good idea to define assumptions that will keep the results consistent in the medical image segmentation process. Even if they are only qualitative assumptions and not quantitative ones.
2D versus 3D
Working on masks does not mean that we have to move only in two dimensions. There are now tools allowing you to work on both cross-sections of DICOM images and their 3D reconstructions [1]. Some features of the model are easier to see or correct in 3D. Details, on the other hand, sometimes need to be refined in individual image layers.
Rome wasn’t built in a day
We may find ourselves in a situation where we are not sure whether what we are looking at is our region of interest. Especially when working on noisy/grainy or blurred images. Therefore, it is worth organizing your work in such a way that you start with a good quality study, without anatomical anomalies, and gradually increase the difficulty level. This approach will avoid initial frustration with difficult data.
Cross-validation
Medical image segmentation is an iterative and time-consuming process. Cross-validation has worked well in our case. Team members check the results of their work with each other, which has three benefits. Mutual learning from each other, catching minor errors thanks to the fresh look of another person, as well as a certain diversification of work – switching from the task of segmentation to verification.
Rethinking the medical image segmentation process
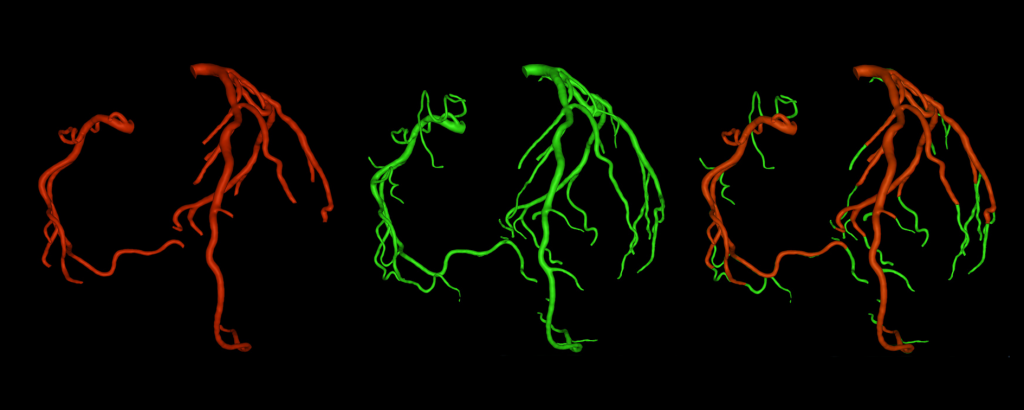
The path we have gone through in the process of medical image segmentation and the experience we have gained allows us to achieve the results that can be seen in the image of the segmented coronary arteries. The image presents: 3D model of the coronary arteries. 1.(from left) Model obtained during segmentation at the initial stage of the project, 2. Current 3D model obtained after refining the process, 3. Comparison of models.
By applying the above points, we were able to improve the quality of our segmentations. We have reconsidered the path it takes to go from models containing major branches to the quality of coronary artery segmentation achieved by medical market leaders. Our segmentations meet high standards, extremely important in developing medical programs at the intersection of AI and medicine.
Read our post: Why does the fight against heart disease need AI?