Post-Operative Delirium and Post-Operative Cognitive Dysfunction prediction model
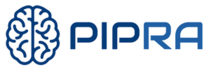
Challenge: Certified Predictive System as a Web App
PIPRA was a newly formed startup that had just received funding for its project as part of the EIT Health Wild Card program supported by the European Union. The company decided that it would not expand its internal structures for the purpose of software development, but would look for an external company to carry out such a project for them. The client partnered with Graylight Imaging because we specialize in Software as a Medical Device solutions.
The challenge was to implement the project in a comprehensive manner, from detailed discussion and verification of the client’s thesis, through the proposal of a solution based on machine learning, parallel production of documentation for the future certification of the system, to the delivery of the finished solution to the client.
The project involved the development of a predictive model on which to base a comprehensive, certified system in the form of a web-based application. There was a lot of time pressure. It began on 1 August 2020 and was expected to be completed in nine months. Graylight Imaging met the deadlines.
Work in progress: Workshop
To ensure project success, we held a pre-development workshop with the client. This session helped us gain a deep understanding of their idea, goals, and specific requirements. Additionally, the workshop allowed us to verify the technological feasibility of the project thesis, guaranteeing the chosen approach could be effectively implemented.
The workshop played an important role. All the necessary information was put together, the uncertainties were clarified, and the focus areas at the concept level that needed to be worked on and rethought were identified. The client was fully supported in this by the experience, competence and technological and scientific knowledge of the Graylight Imaging team.
Work in progress: Predictive Model
GLI software engineers suggested basing the application on machine learning solutions. They developed the model and engineered its features. The data to teach the model was provided by the client. They included several thousand anonymized numerical data presented in tabular form. Some of the data was used to teach the model and some was retained by the client to validate the correct function of the model. Thus, the threat of overfitting the model was eliminated.
The process of fitting the model was challenging because the data available to the GLI team came from different studies and varied in characteristics (age, sex, weight, height, etc.). Not all data were complete. Therefore, a separate predictive model was developed that predicted the missing characteristic for a given patient. The model filled in data gaps.
Work in progress: Web Application & Certification Documentation
According to the client’s requirements, the system was to work as a web application. In addition to machine learning specialists, engineers specializing in web solutions were also engaged to work on the project. The system architecture was based on Python and Django. The team also prepared mock-ups of the application and equipped it with functionalities that make it physician-friendly.
The system was to be certified. So our team working on the project was simultaneously producing documentation that would later be used in the certification (CE) process. Once the work on the predictive model was completed, it was ready for further certification.
Results of our work
The project resulted in a development of a predictive model for data analysis and a web application based on that model. With the unique technology, the physician is supported in the decision-making process of whether surgery should be performed.
Before deciding on the need for surgery, the physician examines the patient and takes their history. The information and test results, e.g., age, sex, past medical conditions, are entered by the physician into the application where the patient is already registered. The predictive model processes the entered data and informs the physician of the patient’s risk – high or low – of developing POD and POCD.
This provides the physician with additional information. The system’s data guides surgical decisions. If surgery appears riskier, physicians may opt for alternative approaches. Additionally, knowing a patient’s risk of complications (POD/POCD) allows for tailored precautions during surgery itself.