Medical image segmentation development process
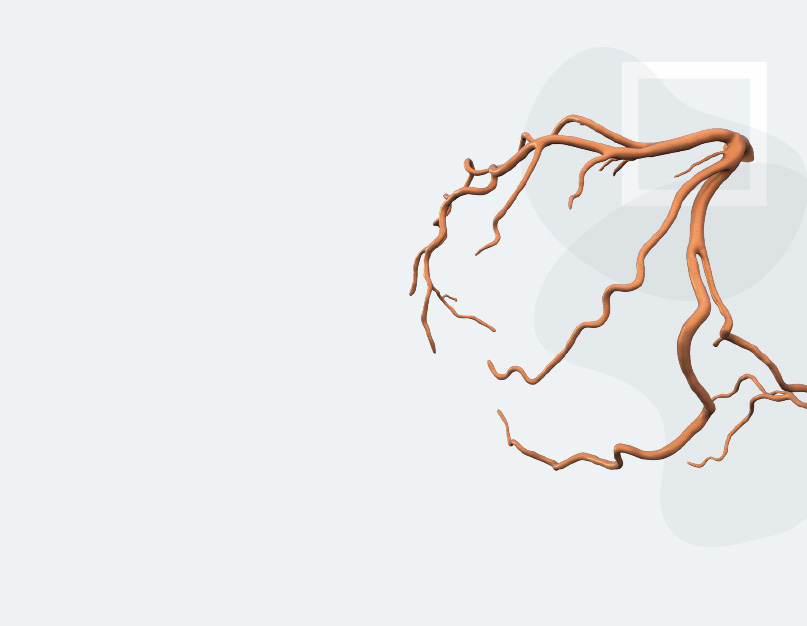
Challenge
Medical image segmentation plays an important role in everyday clinical practice. It enables more effective diagnosing and planning of treatment of neurological lesions of oncological origin, as well as those affecting the cardiovascular system, e.g. coronary artery disease.
Image segmentation, which is the process of dividing an image into specific areas, allows for in-depth analysis. This is due to the fact that it makes it possible to generate a selected anatomical structure in three dimensions and separate it from the adjacent tissues.
Despite the progress that has been made in this field over the past 20 years, many challenges remain. These challenges include obtaining precise and accurate results, as well as developing advanced machine learning algorithms that work not only in the case of normal organs, but also in the event of pathological changes.
Graylight Imaging has over 15 years of experience developing systems for medical imaging. We have developed a methodology and process that enabled us to achieve high-quality results.
What we did
Medical segmentation is only a seemingly easy process. A number of components affect the final effect. Over the past several years, Graylight Imaging specialists have been working to ensure that the models and algorithms created and developed by us are characterized by high quality and precision. They have been applied i.a. in oncology and cardiology. We work with images of different modalities: CT and MRI.
Effective segmentation methodology:
- Cooperation of specialists from various fields – interdisciplinary teams consisting of physicists, specialists in machine learning, electroradiology and biomedical engineering as well as programmers work on the development of segmentation models.
- A team of medical imaging specialists – a highly specialized group of experts is working on highly accurate 3D models of anatomical structures. The team consists of people with competences that allow them to understand both medical and technical issues. Constantly exploring the secrets of human anatomy, with competence to use 3D modeling software. This is where the difficult segmentation process begins, which is essential to building ML models.
- We work on a variety of data – data with different modalities and originating from devices made by different manufacturers.
- Anatomical diversity – we have perfected the imaging precision on anatomically diverse organs, e.g. coronary arteries, which are featured by high anatomical variability.
- Cross-consultation – this is an important element of the process that brings measurable benefits. Members of the team working on segmentations review each other’s results. This approach makes it easier to find minor errors made by a co-worker. But there is more to it. One of the benefits is also the opportunity to learn from each other.
- Our own segmentation software – we have developed an original solution that meets our high requirements and is adapted both to our needs and the requirements for medical devices.
Results
Years of work on medical imaging systems have brought tangible results. We started from high-quality examinations, examinations without anatomical anomalies, and then, as time went on and we gathered more and more experience, we increased the difficulty.
Today, projects involving the creation of segmentation models are handled by teams of highly specialized professionals combining competences from various fields.
Our experience is reflected by successes in both commercial and R&D fields. The models we develop automatically segment the coronary artery tree while maintaining the reconstruction accuracy of over 90% (Sørensen-Dice coefficient of about 0.9) and automatically segment the brain tumor along with its subregions (edema, tumor enhancement and necrosis). They also provide data on volume measurement and RANO (Response Assessment in Neuro-Oncology). These are just a few examples of our capabilities.