RECIST as a perfect biomarker of patient response?
Researchers use RECIST criteria to track solid tumor changes in trials (it’s an important part of trial medical image analysis). These assigns patients a response category (complete, partial, stable, or progressive disease) based on how much a set of target lesions shrinks or grows. It’s done by measuring the total change in the longest diameters of these tumors. However, are these criteria an infallible measure?
RECIST criteria’s historical records
In 1976 James Hanley and Charles Moertel published the first paper on thresholds to decide if a cancer treatment worked. The WHO issued guidelines for evaluating patient response in 1979. They went beyond simple tumor size. Guidelines included CT scans, X-rays, and adopted cut-offs from the mentioned work: -50% for response, +25% for progression. However, key details were missing. How many lesions to measure? Or what defines a measurable lesion [1]? Finally, in 2000, criteria now known as RECIST were published. These were developed based on the analysis of data from 14 clinical trials, involving a total of 4,600 patients. Importantly, RECIST got an update in 2009 (v1.1). It added rules for measuring small lymph nodes and cut the number of tracked lesions to 5 per patient. Again, it relied on data analysis, a literature review and simulations using a massive database of patients and lesions.
Are you supposed to say ‘check’?
Although it is not a chess tournament game, scientific development requires challenging established patterns. At the 2023 ASCO Annual Meeting a group of researchers did so. A team led by Megan Othus (a biostatistician at the SWOG Statistics and Data Management Center) presented a quite interesting poster, describing SWOG S1609 trial results [2]. The trial itself was dedicated to immunotherapy. Researchers were evaluating ipilimumab plus nivolumab across solid rare tumor subtypes. The analysis cohort was 720 patients. Interestingly, it was the first assessment of the correlation between patients receiving checkpoint treatment and changes in RECIST tumor burden. And the results were also extremely interesting.
Does RECIST translate to how well patients do?
RECIST reigns supreme for measuring solid tumor response. Of course, it has variations: mRECIST tackles liver cancer specifically or iRECIST (for immune-based therapeutics). Apparently, just looking at size changes can mislead us about how a patient is responding. In the research by Othus et al. During the mentioned trial a standard RECIST measurement was employed. Clinicians were reviewing follow-up scans and measuring targeted lesions. Changes in tumors’ diameters sum were then classified as complete response, partial response, stable disease, or progressive disease. However, past studies with chemo patients showed these categories might not be perfect. The same happened here. Tumor response seemed to be more like a gradual scale rather than clear-cut stages. This suggests the clear-cut response categories of RECIST might not perfectly match real-world patient outcomes.
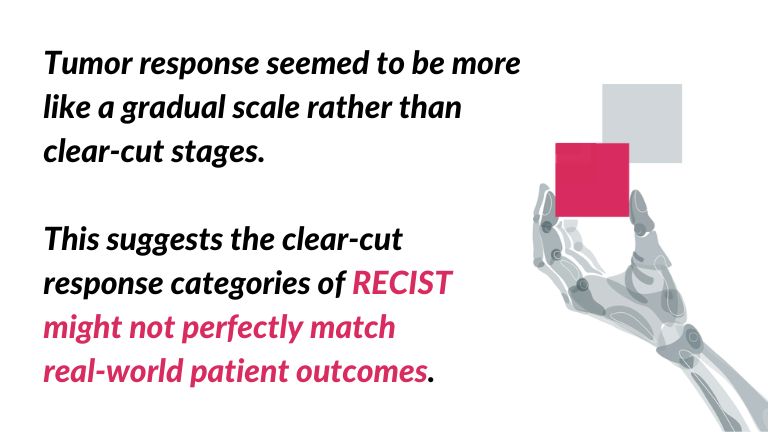
Where the shortcomings step in
But what does this finding actually mean? Let’s have a look at papers investigating the potential pitfalls of RECIST criteria. For the purposes of this discussion, let me leave out such obvious aspects as an interobserver and intraobserver variability. As I noted in one of the previous posts [3], there is a huge urge for this variability reduction. However, we must realize that in addition to the human factor, other factors also must be considered. Firstly, the role of different imaging modalities. Although the CT is well defined in RECIST v1.1, PET-CT and MRI remain limited. Secondly, RECIST relies heavily on size. Some tumors, like desmoids, can respond to treatment by becoming more fibrous, not necessarily shrinking. Therefore, response assessment that does not take morphology into account can be misleading [4].
Is this measurement prone to errors?
As noted by Brian Morse et al. [5], the proper selection of target and non-target lesions is a key. Because non-target lesions aren’t tracked over time, the treatment response becomes more open to subjectivity. RECIST v1.1 criteria suggest that progression by non-target lesions alone should be extremely rare. However, we must remember that once progression is noted, it may affect the trial results. This could cause costly repercussions for a trial sponsor and for a patient (discontinuation of therapy). Additionally, Morse’s study revealed a higher frequency of errors in evaluating non-target lesions compared to target lesions. The authors list the whole bunch of potential RECIST-related errors:
- Misgrouping or skipping non-target lesions
- Not accounting all sites of tumor
- Counting benign growths as malignant ones at the baseline examination
- Misjudging lymph node response based on a small decrease in size
- Indicating worsening based on size changes in non-target lesions
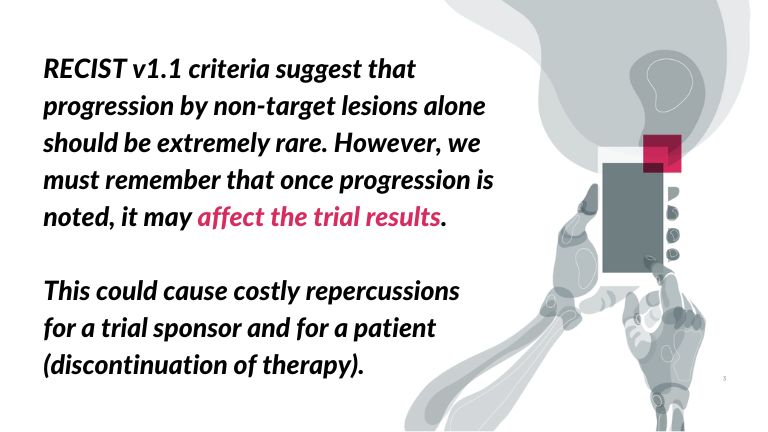
Enhancing measurement strategies
RECIST reproducibility might be disrupted. It is impacted by clinician experience, the decision about the lesions chosen for tracking and their characteristics. Finally, by the detection of new lesions and how they’re interpreted. Because of that some researchers suggest that in certain situations alternative imaging markers for tumor response may be used [6]. Moreover, they advocate for the automation of RECIST paradigm. 3D segmentation would offer several advantages, such as full volume of lesions and elimination of potential bias from reader interpretation in 2D analysis. It would allow for the additional shape metrics to be included. And that might be relevant for a more complete patient response assessment.
Automated RECIST by Graylight Imaging
Our medical imaging analysis experts have had the pleasure of collaborating on several projects in which we have developed algorithms to be use in clinical trials. In one of the projects related to Hepatocellular carcinoma (HCC), we created an automated RECIST algorithm with a great performance (DICE 0,77 for tumors, including primary tumors, secondary tumors and metastases, and 0,95 for liver segmentation). What’s more, it performs volume measurements of targeted tumors. Additionally, there is an option to extend it to mRECIST (read more: [7]). The model itself was developed based on portal venous CT scans. If you believe that such automation could be valuable for your project, we are open to discussing it further. We would also be happy to tell you about other possibilities related to advanced medical image analysis and image biomarkers extraction.
References:
[1] doi: 10.3389/fonc.2021.800547
[2] https://meetings.asco.org/abstracts-presentations/222691
[4] A. Rastogi et al., Tumor Response Criteria in Oncoimaging: RECIST Criteria and beyond — Part 1, Journal of Gastrointestinal and Abdominal Radiology ISGAR Vol. 2 No. 2/2019. https://www.thieme-connect.com/products/ejournals/pdf/10.1055/s-0039-1692021.pdf
[5] Morse B et al., Pearls and pitfalls of response evaluation criteria in solid tumors (RECIST) v1.1 non-target lesion assessment. Abdom Radiol (NY). 2019 Feb;44(2):766-774. doi: 10.1007/s00261-018-1752-4. PMID: 30196362; PMCID: PMC7771310.
[6] Fournier L, et al., Twenty Years On: RECIST as a Biomarker of Response in Solid Tumors an EORTC Imaging Group – ESOI Joint Paper. Front Oncol. 2022 Jan 10;11:800547. doi: 10.3389/fonc.2021.800547. PMID: 35083155; PMCID: PMC8784734.
[7] https://graylight-imaging.com/project/liver-and-liver-tumor-segmentation/