Radiomics: a great potential to impact the future landscape of digital healthcare
Following the three paper recommendations presented on Graylight Imaging’s LinkedIn profile, we would like to focus on the concept of the radiomics method in more detail. Firstly, we are going to introduce basic definitions and pipelines. Secondly, we would like to dive into the current challenges and opportunities of this promising field of research. We invite you to take part in the discussion about the potential risks and future prospects of radiomics.
Introduction to the radiomics method
The intense growth of modern technologies resulted in a constant increase in digitalized information collected during a clinical routine. That opened new opportunities for analysis, but the amount and complexity of data required novel methods of automated processing. Radiomics is an emerging data-driven field of science focusing on the development and analysis of biomarkers extracted from medical images. The introduction of advanced machine-learning techniques led to the rapid development of radiomics research. How? By providing not available earlier tools for the successful detection of patterns. Furthermore, patterns not obtainable to radiology specialists through manual examination. We may use automated extraction of large amounts of quantifiable features for disease diagnosis, survival prediction, the assessment of response to a treatment, and many more [1].
Radiomic features encode complementary biologic information from anatomical (e.g., MRI, CT) and functional (e.g., PET, fMRI) imaging. Features may be obtained by hand-crafted algorithms (figure 1) or through deep learning techniques which benefit from automated representation learning. We may split such hand-crafted and deep features into the following groups based on their characteristics [2]:
- shape-based features – descriptors of 2D or 3D binary segmentation masks
- first-order statistical features – describe the distribution of voxel intensities within the segmented image region, e.g., organ or tumor
- texture features – describe complex relationships between multiple voxels of the image
- other – features built upon transformed input images (e.g., by extraction of local binary patterns) or by novel custom algorithms

Figure 1: Liver Surface Nodularity Score as an example of hand-crafted feature for assessment of liver cirrhosis in CT image [3]
Radiomic pipeline
A typical radiomic pipeline consists of the following steps [4]:
- image data acquisition and reconstruction
- segmentation of the region of interest
- feature extraction and selection
- data analysis
- prognosis/prediction
Figure 2: Example of radiomics pipeline for survival prediction [5]
Radiomic workflows vary according to a particular application or desired clinical outcome. Figure 2 represents an example of radiomic pipeline that uses structural MR data to estimate survival prediction. Real-life multi-disciplinary radiomic pipelines incorporate radiomic features in many various ways: as data augmentation tool to feed a neural network with more training samples, to explain features produced by deep learning algorithms [6], or to combine deep learning and hand-crafted features in a radiomic-guided approach [7].
Opportunities offered by radiomics method
Radiomics has a great potential to impact the future landscape of digital healthcare.
Hand-crafted radiomic features are well-defined and easier to interpret by a trained specialist. They reflect important clinical properties of anatomical structures by transforming original image information. You may generate such features uniquely for the specific task by custom algorithms to obtain better and more reliable results.
Though used mostly in oncology, there are many non-oncologic research areas where radiomic analysis proved effective. For instance, research on Alzheimer’s disease [8], multiple sclerosis [9], and lung diseases [10]. A typical radiomic pipeline relies on ground-truth segmentations to extract features from the masked scans. It is not always the case though – the preparation of high-quality ROI segmentation is a time-consuming and laborious task. Not to mention, full 3D scans offer advantages over masked data in certain applications. We must remember that masked data might not capture the whole picture for some analyses.
It is a non-invasive method for screening and clinical assessment of anatomical structures as a whole. This approach is especially useful for the examination of tumors. Due to their heterogeneous nature, biopsy may not always hit the change and provide reliable results. Cancerous lesions may grow in sensitive hard-to-access areas, so we may employ radiomic analysis to assess the tissue and avoid invasive intervention. A great example of such an approach is the brain tumor. Brain biopsies are strenuous for the patient with risk of possible complications. There are several features serving as robust indicators of brain lesion types [11] or for response assessment to the therapy [12]. There are many constraints regarding such algorithms. You must precisely follow consistent acquisition protocols to ensure reliable results. This is the case especially for MR studies. The representation of voxel intensities in this modality isn’t covered by standardized units.
A great step towards personalized medicine?
You may consider radiomic-based applications as a support tool for clinical decision-making in immuno- and radiotherapy [12]. Therapy prognosis and survival prediction are some of the hardest tasks in clinical routine, burdened with great responsibility toward patient’s well-being. Radiomic approach is successfully used in clinical studies that require the assessment of response to the therapy [13].
Once used for the treatment assessment, why not take a step further by selecting in advance the optimal treatment that would work best for a particular patient? That way we could tailor therapy to fulfill needs of an individual and maximize the therapeutic effect [13]. Such set of biomarkers is referred to as radiomic signature of a patient [5] [14]. Researchers can use it as a first step toward personalized medicine.
Challenges to be addressed
Besides undeniable highlights, radiomics method faces many challenges. A radiomic pipeline consists of many processing steps. Moreover, all of them require standardization and transparency. Robust end-to-end evaluation procedures must be implemented along with the test-retest approach in clinical settings. This combination is necessary to provide the final evidence about radiomics efficacy. Researchers have already identified the following limitations [1] [4]:
- Retrospective studies – one of the basic problems pertains to the historical aspects of radiomics development. Obviously, there are various open-access datasets. However, plenty of them come from retrospective clinical studies. They didn’t follow standardized procedures. Many of them used some arbitrarily chosen cut-off values or parameters. Certainly, a solution would be to plan prospective studies while having the radiomic method in mind from the beginning.
- Heterogeneous cohorts – often precise information about tested cohorts is missing. Unquestionably, ensuring patient diversity in our data is crucial. This diversity helps create a robust prediction model that generalizes well to new data.
- Insufficient standardization – as I have said, the entire pipeline needs standarisation. Starting from data acquisition, through pre- and postprocessing, to extraction of well-defined features and evaluation using commonly-used metrics.
- Limited amount of data – in medical field the amount of extracted features is often much higher than the available patient data. We need to apply an extensive feature reduction/selection to avoid the curse of dimensionality.
- Interpretation of results – the lack of well-established prognostic and predictive references makes it impossible to evaluate results reliably and assess which features are truly relevant clinically. Furthermore, mistakes may result from incorrect interpretation of the results or flawed analysis of data.
- Technical integration – seamless integration with hospital workflow is necessary, so that the radiomic pipeline is fully incorporated into daily routine of radiologists. This is crucial to collect important feedback and to not lose the clinical perspective.
Strategies to get the most out of the radiomics method
One of the most critical issues is the standardization of every step of the processing. Different acquisition protocols used by different institutions result in different data obtained for the same patient. The scanning protocols have a great impact on generated data, especially inconsistent acquisition parameters of the scanners, such as: reconstruction kernel for CT, magnetic field settings and lack of standardized units for MR. Another story is time-dependent variables, such as: contrast dosage and timing – difference in timing between the injections of contrast agents affects voxel intensities and may result in generation of different images even for the same scanner. Figure 3 presents other burdensome parameters. To resolve that issue literature proposes several methods, such as: modeling the relationship and applying corrections accordingly [15], deep learning approach [16], post-reconstruction batch harmonization in order to harmonize radiomic feature sets by ComBat method [17].
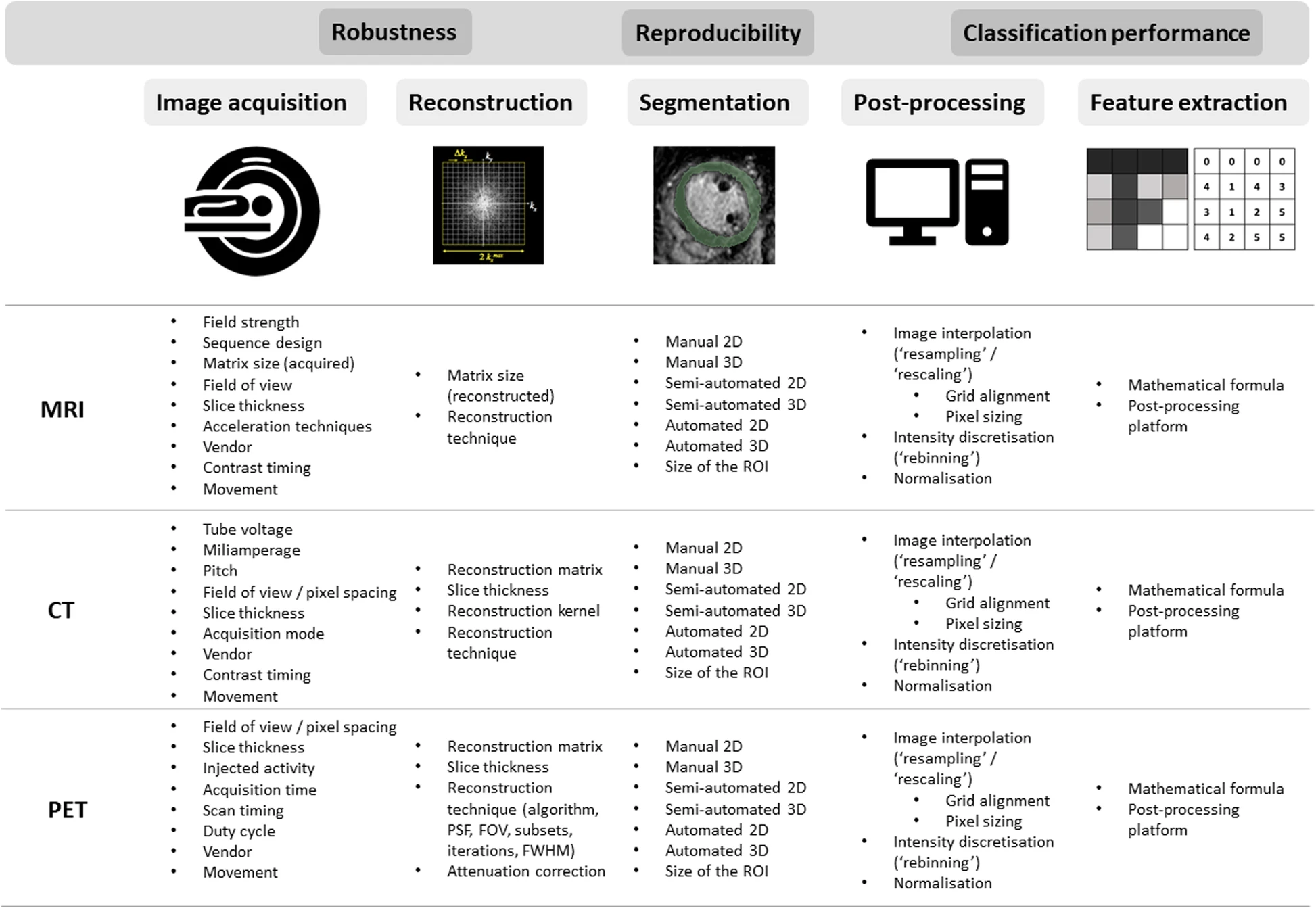
Figure 3: Technical factors impacting different steps of radiomic pipeline [4]
To ensure repeatability and consistency of radiomic features the following initiatives have been introduced:
- Image Biomarker Standardisation Initiative (IBSI) to standardize mathematical formulas of feature definitions, processing steps, and resampling techniques [18]: https://theibsi.github.io/
- Radiomics Quality Score (RQS) checklist: https://www.radiomics.world/rqs2
- Transparent Reporting of a multivariable prediction model for Individual Prognosis Or Diagnosis (TRIPOD) statement [19]: https://www.tripod-statement.org/
Another approach is to create datasets tailored solely for verification of protocol consistency. There are publicly available datasets like the RIDER dataset to help to assess the impact of varying factors in radiomics. The public phantom dataset, designed for radiomics reproducibility tests on CT, helps to assess the impact of acquisition settings in order to eliminate non-robust radiomic features [4].
Radiomics method – future perspectives
Radiomics method is an interesting and promising field of research, but there are still many unanswered questions regarding technical and regulatory aspects. It needs to be incorporated into prospective studies to prove its value in a real clinical environment. The implementation of robust validation protocols will be the key to reproducibility, transparency and standardization of the entire processing pipelines. In my opinion, the hand-crafted radiomic features should be used along with the deep learning techniques to enrich current applications and provide us with new exciting solutions in the future.
We have gained experience in quantitative image analysis through development of our own deep learning-powered system for processing of DCE-MRI studies. This application will be described on Graylight Imaging LinkedIN profile in one of the next posts, and we will have another opportunity to share our insights regarding radiomics method and quantitative image analysis.
References:
[1] https://pubmed.ncbi.nlm.nih.gov/32101448/
[2] https://pubmed.ncbi.nlm.nih.gov/29092951/
[3] https://pubmed.ncbi.nlm.nih.gov/27089026/
[4] https://insightsimaging.springeropen.com/articles/10.1186/s13244-020-00887-2
[5] https://www.oncotarget.com/article/20423/text/
[6] https://pubmed.ncbi.nlm.nih.gov/30854457/
[7] https://www.nature.com/articles/s42003-021-02814-7
[8] https://pubmed.ncbi.nlm.nih.gov/32124697/
[9] https://pubmed.ncbi.nlm.nih.gov/34531195/
[10] https://pubmed.ncbi.nlm.nih.gov/34202096/
[11] https://www.frontiersin.org/journals/oncology/articles/10.3389/fonc.2021.732196/full
[12] https://pubmed.ncbi.nlm.nih.gov/32504782/
[13] https://pubmed.ncbi.nlm.nih.gov/31448366/
[14] https://pubmed.ncbi.nlm.nih.gov/32357923/
[15] https://pubmed.ncbi.nlm.nih.gov/31240330/
[16] https://pubmed.ncbi.nlm.nih.gov/31210613/
[17] https://pubmed.ncbi.nlm.nih.gov/29301932/
See the previous post: The Beauty of Seeing Beyond the Visible: Automating the DCE-MRI Analysis of Brain Tumors