The Beauty of Seeing Beyond the Visible: Automating the DCE-MRI Analysis of Brain Tumors
Magnetic resonance imaging (MRI) plays a key role in modern cancer care. It allows us to non-invasively diagnose a patient. Clinicians can determine the cancer stage, monitor the treatment, assess and quantify its results. What’s more, understand its potential side effects in virtually all organs. For instance, you may exploit MRI to better understand both structural and functional characteristics of the tissue thanks to imaging biomarkers. Such detailed and clinically-relevant analysis of an imaged tumor can help design more personalized treatment pathways, and ultimately lead to a better patient care. Additionally, MRI does not use ionizing radiation. We can use it to acquire images in different planes and orientations. MRI (with and without contrast) is the investigative tool of choice for neurological cancers. For brain tumors, we commonly acquire multi-modal MRI, including T1-weighted (contrast and non-contrast), T-weighted, Fluid Attenuation Inversion Recovery (FLAIR) sequences, alongside diffusion and perfusion images [1].
Why to automate the analysis process?
Analyzing an input MRI study requires the critical steps of detecting and segmenting brain lesions. Firstly, they significantly influence the further steps of the lesion analysis, e.g., extracting its quantifiable characteristics, such as bidimensional or volumetric measurements. Incorrect delineation may easily lead to improper interpretation of the captured scan and adversely affect the treatment pathway [2]. A tremendous amount of MRI data generated every day drives the development of machine learning brain lesion segmentation systems. However, such data is extremely imbalanced (only the minority of all pixels or voxels in 3D present a tumorous tissue), it is very large and heterogeneous. Obviously, this heterogeneity may be present not only due to different scanners and/or protocols utilized to capture the image.
Inter- and intra-observer variability effect
It may also result from various tumor characteristics and intrinsic features captured in the image. Undeniably, manual delineation of MRI scans is time-consuming and tedious. Manually segmenting lesions at high quality for supervised training presents a significant challenge. Additionally, readers may disagree on how to delineate the same scan, leading to discrepancies. What’s more, even the same reader can produce different segmentations of the same scan at different times. And – in general – the quality of manual segmentations significantly vary [3]. This questionable quality of manually-generated ground-truth information is an important problem in the case of supervised methods, because they directly affect the quality of a trained model.
Fully-automated extraction of imaging biomarkers
Fully-automated medical-image segmentation pipelines, e.g., exploiting image analysis and machine learning, are thus of great interest, as they can accelerate diagnosis, ensure reproducibility, and make comparisons much easier (e.g., comparing the manual delineations performed by two readers at two different oncology centers alongside the extracted brain-tumor numerical features is extremely challenging if they did not follow the same segmentation protocol). Also, we are aimed at decreasing the overall analysis time. Finally, we want to reduce false negatives, being the tumorous pixels/voxels that were incorrectly classified as healthy by a machine learning model.
The beauty of seeing in four dimensions
In dynamic contrast-enhanced MRI (DCE-MRI), once the contrast bolus is injected, it captures the voxel intensity changes within a volume of interest (e.g., a brain tumor), and it allows for quantifying the dynamic processes within a tissue based on such temporal changes of voxel intensities (hence, our fourth dimension is time). Imaging biomarkers extracted from DCE-MRI can be used in patient prognosis, risk assessment and quantification of tumor characteristics and stage [4]. In DCE-MRI, a contrast agent flows through the vascular system to the analyzed volume which is reflected in the observed signal. The analysis process of such imaging involves manual (or semi-automated) delineation of a volume of interest, alongside the vascular input region that is used to calculate the vascular input function (VIF). Although it is possible to determine the perfusion parameters without calculating the contrast concentration in VIF, it may result in inaccurate perfusion parameters.
The beauty of seeing beyond visible
Do we have to suffer from all drawbacks of the manual analysis though?
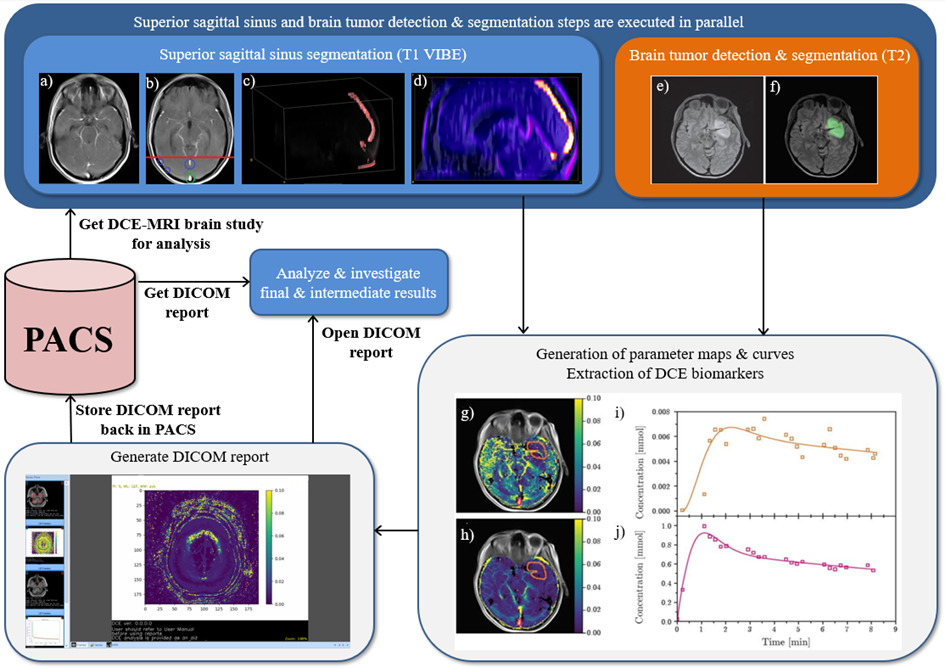
Figure 1: Automated Dynamic Contrast-Enhanced Magnetic Resonance Imagining analysis
Superior sagittal sinus segmentation (SSS) (T1 VIBE): a) Original T1 VIBE image, b) candidate SSS regions, c) 3D rendering of SSS after pruning false-candidates, d) 3D rendering of SSS segmented using our approach. Brain tumor detection & segmentation (T2): e) Original T2 image. f) Original T2 image with our segmentation – green area represents agreement with a reader, red area represents false negatives, while blue area represents false positives (DICE=0.954). Generation of parameter maps, curves, DCE imaging biomarkers extraction: g) Values of Ktrans and h) Ve are the results of fits of the Tofts’ model (pixelwise), superimposed onto the original image – orange contour represents the tumor, pink – superior sagittal sinus (SSS). i) Orange points represent an average contrast concentration in the area of the tumor, the orange curve represents the fit of the model. j) Pink points represent an average contrast concentration within SSS, the pink curve is the fit of an Artery Input Function. The pharmacokinetic parameters obtained from the fits are Ktrans = 0.0135 [min-1] and ve = 0.0084. Note that the SSS segmentation and brain tumor detection & segmentation steps are executed in parallel to speed up processing (we rendered a step which exploits deep learning & GPU processing in orange).
Imaging biomarkers - to accelerate analysis
To accelerate the analysis process, and to make it fully reproducible, we proposed an automated deep learning-powered system for extracting imaging biomarkers from DCE-MRI [5]. For each pixel (voxel), we analyze its temporal contrast-flow characteristics. Additionally, we generate the parametric maps that visualize the perfusion parameters in the compartment model (see the example artefacts gathered in Figure 1). Here, we extracted Ktrans and ve, being the influx volume transfer constant (or the permeability surface area product per unit volume of tissue between plasma and extravascular extracellular space, EES), and the volume of EES per unit volume of tissue, respectively.
The multi-faceted experimental analysis
The system consists of two algorithms. An algorithm for determining the vascular input region which benefits from fast image-processing techniques (in both 2D and 3D). The second one was an algorithm for segmenting brain tumors (from one or more MR sequences). In the latter case, we exploited U-Net-based architectures, in which an ensemble of such models was trained over non-overlapping training sets. Also, we introduced a new VIF model, being an extension of the linear model – it was used in pharmacokinetic modeling. Furthermore, we verified all the algorithms against benchmark and clinical data for brain tumor segmentation. The multi-faceted experimental analysis included the mean opinion score experiment (for more details see the post [6]). 12 experienced radiologists, 3 to 11 years of experience, were quantitatively evaluating the segmentations. They reviewed both ground-truth and elaborated using the proposed technique.
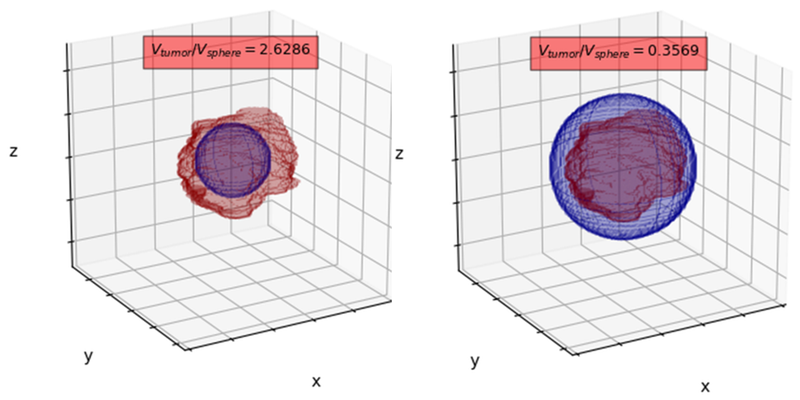
Does segmentation affect imaging biomarkers’ extraction?
Also, we verified the influence of the segmentation accuracy on the extracted perfusion parameters of the analyzed tumor. Here, an obvious question may pop up: does segmentation accuracy affect extraction of DCE-MRI imaging biomarkers? To verify this impact, we simulated inscribed (left panel in Figure 2) and circumscribed (right panel in Figure 2) spheres which approximate manual segmentation and statistically compared the Ktrans and ve values. Note that the volume of the corresponding spheres is significantly different from the volume of the annotated BT (we report the volume ratios above the spheres). Interestingly, Bland-Altman analysis showed high level of agreement between perfusion parameters (Ktrans & ve) for GT, circumscribed and inscribed spheres approximating GT (Figure 3).
Experimental results versus reality
The experimental results were fundamental in the clinical validation report of the product, Sens.AI (https://sensai.eu/en/). A clinical validation report is a document that is pivotal in the process of applying for the CE mark for medical devices. The component for detecting and segmenting brain tumors from MRI (FLAIR) has been CE-certified as medical device. Such thorough and evidence-based verification and validation is always the key in medical image analysis. We need to precisely understand what does work and what does not (and why).
Concluding remarks on imaging biomarkers
DCE-MI imaging plays an important role in the diagnosis and grading of brain tumors. Quantitative DCE imaging biomarkers provide information on tumor prognosis & characteristics. However, their manual extraction is time-consuming and prone to human errors. In this study, we introduced an automated approach to extract DCE-MRI from brain-tumor MRI without user intervention. Obviously, we also verified all of its critical components over benchmark and clinical data.
Process step by step
For vascular input region determination, we used 3D (connected-component) analysis of thresholded T1 sequences, whereas brain tumors are detected and segmented using a voting ensemble of U-Net-based deep neural networks. Besides, we employed piecewise continuous regression to estimate bolus-arrival time and utilized a new cubic model for pharmacokinetic modeling. To this end, we fully automated the end-to-end DCE-MRI process. It is faster than pouring a cup of coffee, as it takes less than 3 minutes for an input MRI scan. Additionally, it is independent of human errors and bias.
Can the process be further enhanced? Sure it can – how about extracting radiomic features from the original MRI scan, and perhaps from the parametric maps to get the full picture?
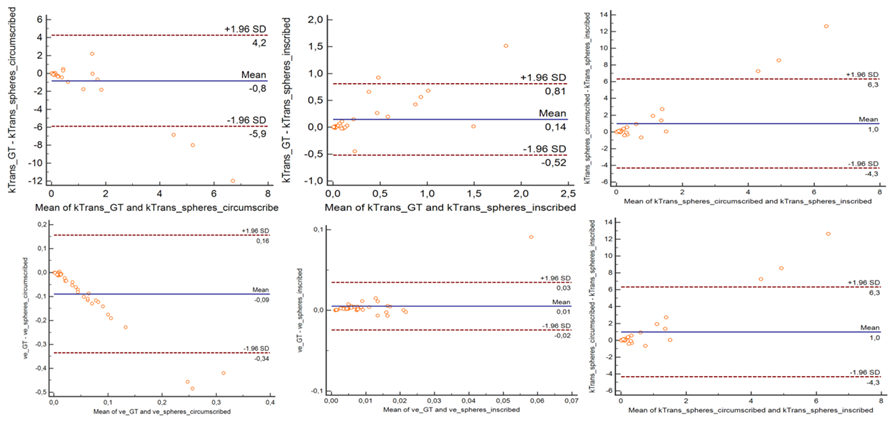
Figure 3: Bland-Altman analysis showed high level of agreement between perfusion parameters (Ktrans & ve) for GT, circumscribed and inscribed spheres approximating GT.
References:
[1] J. E. Villanueva-Meyer, M. C. Mabray, and S. Cha, Current Clinical Brain Tumor Imaging, Neurosurgery, vol. 81, no. 3, pp. 397 – 415, 05 2017.
[2] R. Meier, U. Knecht, T. Loosli, S. Bauer, J. Slotboom, R. Wiest, and M. Reyes, Clinical evaluation of a fully-automatic segmentation method for longitudinal brain tumor volumetry, Scientific Reports, vol. 6, no. 1, pp. 23376, 2016. https://pubmed.ncbi.nlm.nih.gov/27001047/
[3] M. Visser, D. Müller, R. [van Duijn], M. Smits, N. Verburg, E. Hendriks, R. Nabuurs, J. Bot, R. Eijgelaar, M. Witte, M. [van Herk], F. Barkhof, P. [de Witt Hamer], and J. [de Munck], Inter-rater agreement in glioma segmentations on longitudinal MRI, NeuroImage: Clinical, vol. 22, pp. 101727, 2019.
[4] C. Cuenod and D. Balvay, Perfusion and vascular permeability: Basic concepts and measurement in DCE-CT and DCE-MRI, Diagnostic and Interventional Imaging, vol. 94, no. 12, pp. 1187 – 1204, 2013.
[5] J. Nalepa, P. Ribalta Lorenzo, M. Marcinkiewicz, B. Bobek-Billewicz, P. Wawrzyniak, M. Walczak, M. Kawulok, W. Dudzik, K. Kotowski, I. Burda, B. Machura, G. Mrukwa, P. Ulrych, M. P. Hayball, Fully-automated deep learning-powered system for DCE-MRI analysis of brain tumors, Artificial Intelligence in Medicine, vol. 102, pp. 101769, 2020. doi: 10.1016/j.artmed.2019.101769