Generative Adversarial Networks (GANs) in healthcare
By: Jarosław Gośliński
Today, we would like to discuss Generative Adversarial Networks, or GANs, which can be used for various healthcare and medical imaging applications like e.g., modality translation.
While this may look like a scene from a science-fiction movie, deep learning networks such as GANs and their sister network, Cycle-GAN, have proven to be capable of such tasks with relative ease. One of the advantages of GANs is their ability to learn in an unsupervised manner without requiring paired samples, making it possible to convert images from one modality to another or low-quality images into high-quality ones.
The ability of GAN in the medical imaging area to synthesize realistic images offers the potential to the medical image analysis area. If your project involves medical imaging analysis or deep learning, GANs could be a valuable tool to explore.
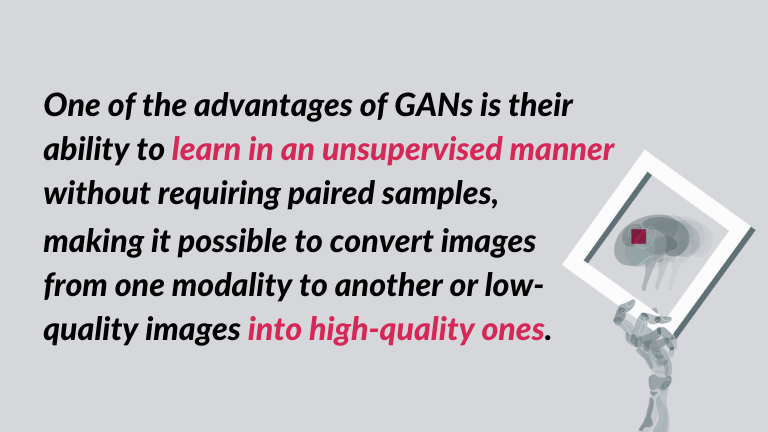
The role of GAN for medical image analysis
Recent studies have demonstrated the potential of Cycle-GANs in medical imaging [1], such as the translation of PET images into CT images or generating artificial CT images of COVID-19 human chest [2] These applications have become increasingly important due to the scarcity of training medical data for deep learning networks, and the ability of Cycle-GANs to generate more labeled images in an unsupervised manner.
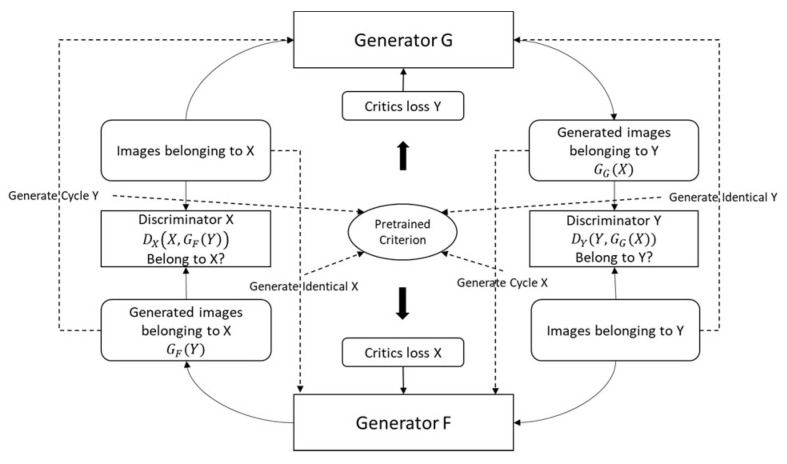
Figure 1. Ad CycleGAN Architecture. Source: Liang Z, Huang JX, Antani S. Image Translation by Ad CycleGAN for COVID-19 X-Ray Images: A New Approach for Controllable GAN. Sensors (Basel). 2022 Dec 8;22(24):9628. doi: 10.3390/s22249628. PMID: 36559994; PMCID: PMC9785652.
Application of GAN in various medical areas
As noted by Koshino et al., cycle-GANs can be applied in several areas of medical imaging, such as:
- image synthesis,
- modality conversion,
- de-noising,
- image reconstruction,
- high-resolution image generation,
- and domain adaptation [3].
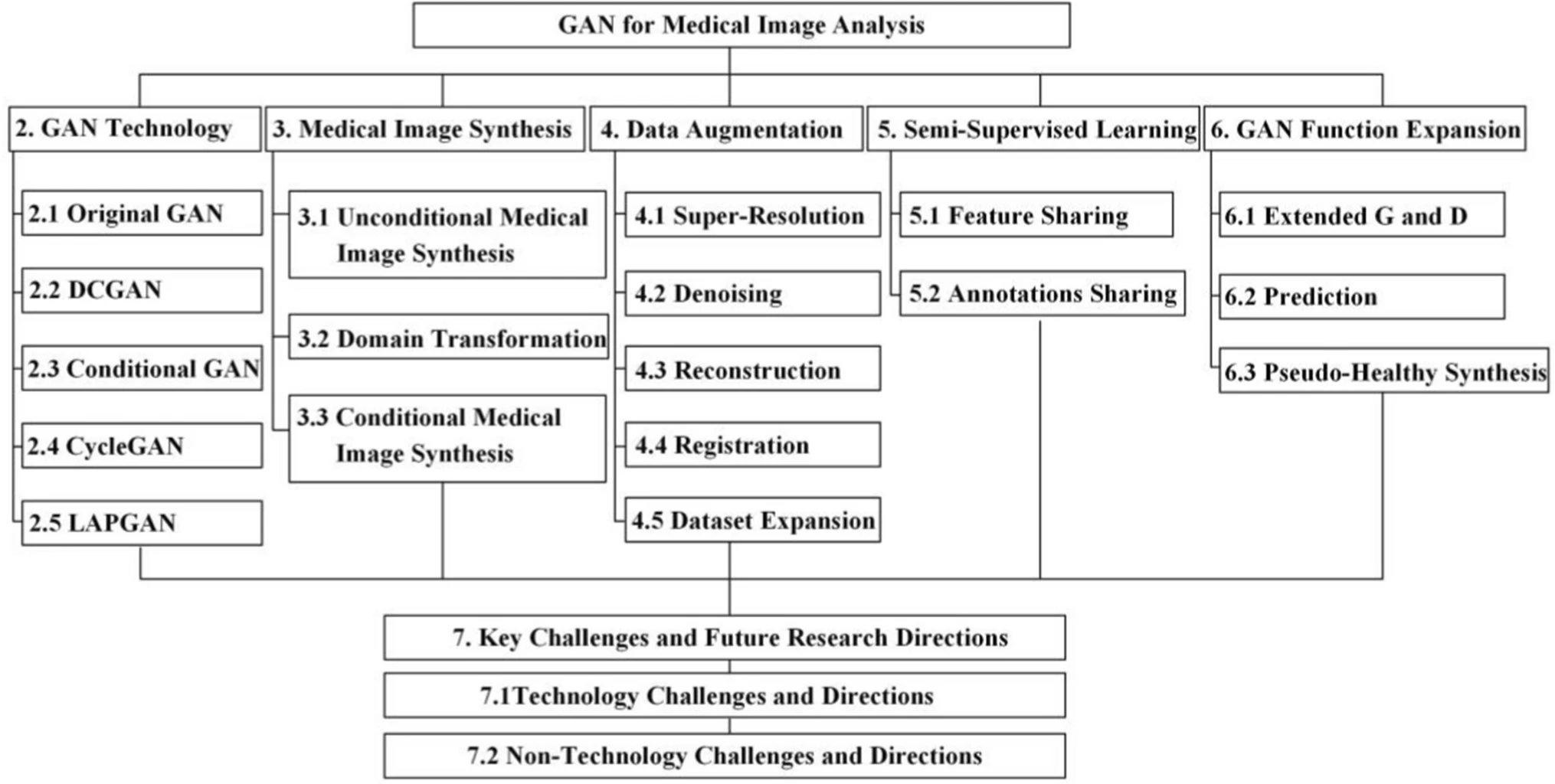
Figure 2. Source: Li, X., Jiang, Y., Rodriguez-Andina, J.J. et al. When medical images meet generative adversarial network: recent development and research opportunities. Discov Artif Intell 1, 5 (2021). https://doi.org/10.1007/s44163-021-00006-0
However, the use of synthesized images for lesion segmentation and detection is still controversial, and proper usage is crucial to avoid potential biases and inaccuracies [4].
Cycle-GANs in medical imaging
Despite these concerns, recent studies [5] have shown the potential of GANs in various subfields of medical imaging, and their reliability has been confirmed with proper application. Therefore, it is essential to carefully evaluate the reliability of GAN-generated images and use them appropriately in medical imaging applications.
Our team has been experimenting with Generative Adversarial Networks (GAN in medical imaging) not since yesterday. We would be excited to talk about whether they could be applicable to your project.
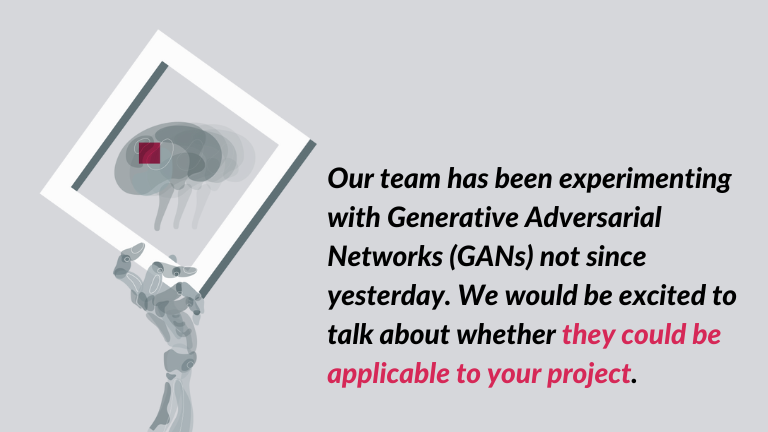
Read the previous post about our expertise in cutting-edge technology: Generating Smooth Connections in 3D Mesh Models of Brain Arteries