Automated RANO and automated RECIST algorithms at your service?
The evaluation of treatment response is of utmost importance for determining the effectiveness of therapeutic interventions and guiding patient management. Over the years, various criteria, such as the Response Assessment in Neuro-Oncology Criteria (RANO) and the Response Evaluation Criteria in Solid Tumors (RECIST), have been developed to standardize the measurement of treatment response and medical image analysis. Yet, the irregular and complex shapes of tumors, e.g. gliomas pose a challenge to conventional bidimensional measurements, raising the need for automation.
At Graylight Imaging we have developed and applied to real world data our fully automated algorithms. They provide precise and comprehensive patient response measurements adhering to RANO and RECIST criteria (automated RANO and automated RECIST). What’s interesting – our technology can do much more than what the guidelines indicate.
RANO and RECIST: limitations and inter-observer variability
Our research project focused on developing automated algorithm for precise and comprehensive patient response measurements as well as volumetric data. The study involved a substantial number of pre-operative and post-operative adult patients with glioma.
The automated RANO algorithm demonstrated its potential to offer a more precise assessment of tumor burden than manual RANO measurements. While various assessment systems share similarities, the devil lies in the details, and even minor differences in criteria can significantly impact the interpretation of results and subsequent treatment decisions. Whats more: manual measurements may not be easily reproducible by other radiologists or in follow-up examinations. Summing up, this can make it difficult to monitor the progression of a disease or assess treatment outcomes consistently.
Additional measurements for clinical research
In clinical practice, providing additional measurements, especially for tumors smaller than those with a bidirectional diameter of ≥ 10 mm, can be extremely challenging. Similarly, accurately measuring the specific response of a tumor in subsequent follow-ups for a patient, regardless of percentage growth or regression, poses significant difficulties.
For smaller tumors, manual measurements become more prone to errors and inconsistencies due to the limited size and visibility of the lesions. When monitoring the response of a tumor over time, using manual measurements alone may not provide a comprehensive and precise assessment. During treatment, tumors undergo complex changes in size, shape, and appearance. These changes make it challenging to accurately measure a tumor’s response using only manual methods.
We strongly believe that the automation aspect brings added efficiency and reliability to the process, which is needed for e.g. in clinical trials.
RANO criteria versus 3D volumetric measurements in neuro-oncology
The particular significance of the algorithm developed by our team lies in the segmentation of the tumor into subregions and the calculation of their volumes.
For many years, a debate has been ongoing within the medical community regarding whether bidimensional (2D) measurements should be enriched or even replaced by 3D measurements, however we’re still facing a lack of definitive resolution on this matter.
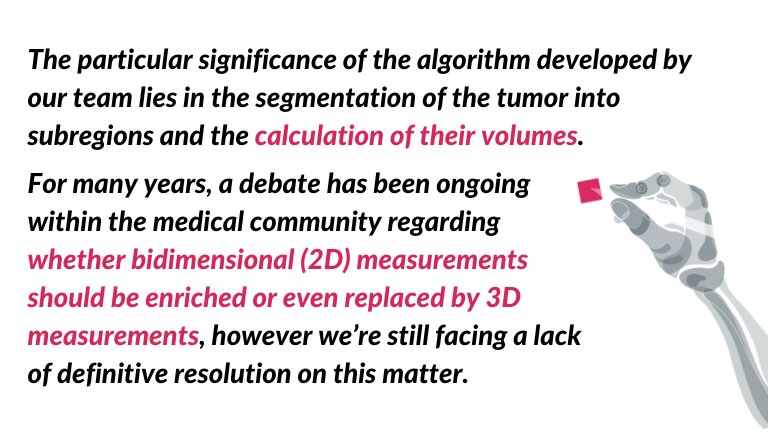
Volumetric measurements potential
Various studies have explored the correlation between RANO measurements and tumor volume measurements in the context of medical imaging and response assessment. Interestingly, certain research has shown remarkable consistency between the two measurement methods, while other studies present conflicting findings, indicating a discrepancy between RANO measurements and the volumetric one. As Diana Bardo et al. showed, in the chosen group of patients, the 3D measurements showed considerable variation and did not align with RANO measurements. This discrepancy indicated that some patients exhibited minimal changes in tumor size. Others experienced significant tumor growth during the same time frame. Of course, as the authors emphasize, – to validate the reliability and practicality of 3D volumetric assessment for measuring treatment response in upcoming clinical trials involving low-grade astrocytoma, larger-scale studies are necessary. [1]
Although this discussion has been ongoing for many years, there is an increasing consensus that response assessment in neuro-oncology, particularly in clinical trials, requires… The improvement to minimize the so-called “human error” and maximize the accuracy in determining the true response to specific therapies. [2] Volumetric measurements have the potential to provide a more precise assessment of tumor burden, however they are too laborious to be performed manually.
Automated RANO measurement enriched by volumetric data in glioma clinical trial
The study we performed was conducted over 760 pre-operative and 504 post-operative cohorts of adult patients with glioma. To the best of our knowledge, it is the first algorithm of its type. It is capable of identifying and segmenting multiple tumor areas in glioma patients both before and after surgery.
This yielded three significant contributions:
- A completely automated algorithm that accurately computes two-dimensional measurements in post-contrast T1 sequences. It works strictly following current RANO criteria.
- Moreover, it provides measurable volumetric characteristics for subregions: necrosis, edema and enhancing tumor.
- A more robust alternative to the automated 2D RANO. The solution optimizes the product of diameters instead of the maximum diameter. Importantly, this approach proves to be less affected by minor changes in the lesion’s contour. [3]
As has been noted, the validation indicates that our automated RANO algorithm offers great precision. You receive a more precise assessment of tumor burden compared to manual RANO measurements. In addition of course to the benefit of full automation.
Benefits of Automated RANO and Automated RECIST measurements within your reach
Our automated RANO algorithm brings forth several benefits, primarily in the segmentation of tumors into subregions and the calculation of their volumes. By automating these processes, we can achieve increased efficiency and reduced time consumption compared to manual measurements. Additionally, automation minimizes the influence of human subjectivity, ensuring more consistent and standardized results across different radiologists or follow-up examinations. Manual methods can struggle with measuring smaller tumors accurately. Computer-assisted tools and AI algorithms offer a solution, enabling us to handle these measurements with improved accuracy.
Study | Whole tumor vol. mm3 | Enhancing tumor vol. mm3 | Non-enhancing tumor/necroris vol. mm3 | Edema vol. mm3 | Lesion 1# RANO | Lesion 1# volume mm3 |
---|---|---|---|---|---|---|
05-06-2004-NA Baseline segmentation | 8190.98 | 3995.99 | 866.99 | 3327.99 | 398.84 | 3995.99 |
07-08-2004-NA-Control visit | 11835.00 | 2.00 | 1135.00 | 10698.00 | 0.0 | 2.00 |
08-16-2004-NA-Control visit | 9484.72 | 1.23 | 20.04 | 9463.44 | 0.0 | 0.35 |
10-04-2004-NA-Control visit | 131.49 | 0.0 | 131.49 | 0.0 | 0.0 | 0.0 |
12-23-2004-NA-Control visit | 13251.99 | 791.24 | 135.99 | 12324.74 | 116.31 | 791.24 |
03-30-2005-NA-Control visit | 24794.97 | 184.49 | 274.99 | 24335.47 | 43.01 | 184.49 |
12-08-2005-NA-Control visit | 46902.49 | 0.0 | 0.0 | 46902.49 | 0.0 | 0.0 |
03-02-2006-NA-Control visit | 55983.99 | 0.0 | 48.49 | 55935.49 | 0.0 | 0.0 |
Data source: https://wiki.cancerimagingarchive.net/pages/viewpage.action?pageId=133073470
Citation: Zolotova, S. V., Golanov, A. V., Pronin, I. N., Dalechina, A. V., Nikolaeva, A. A., Belyashova, A. S., Usachev, D. Y., Kondrateva, E. A., Druzhinina, P. V., Shirokikh, B. N., Saparov, T. N., Belyaev, M. G., & Kurmukov, A. I. (2023). Burdenko’s Glioblastoma Progression Dataset (Burdenko-GBM-Progression) (Version 1) [Data set]. The Cancer Imaging Archive. https://doi.org/10.7937/E1QP-D183
TCIA Citation: Clark, K., Vendt, B., Smith, K., Freymann, J., Kirby, J., Koppel, P., Moore, S., Phillips, S., Maffitt, D., Pringle, M., Tarbox, L., & Prior, F. (2013). The Cancer Imaging Archive (TCIA): Maintaining and Operating a Public Information Repository. In Journal of Digital Imaging (Vol. 26, Issue 6, pp. 1045–1057). Springer Science and Business Media LLC. https://doi.org/10.1007/s10278-013-9622-7
Final steps?
The algorithm described above is not our first step in this very area. It is more a continuation in studies focusing on precise and automated patient response assessment. Some time ago, we delved into the automated measurement of RECIST. Our solution conducts automated tumor volume and automated RECIST measurements, and if appropriate training data is accessible, it can be extended to mRECIST measurements as well. [4]
References:
[1] Dianna Bardo et al., radi-16. Rano criteria and volumetric tumor measurement produce significantly incongruous results in non-radiated low grade astrocytomas which may lead to misinterpretation of therapy response, https://doi.org/10.1093/neuonc/noy059.656
[2] Benjamin M. Ellingson et al., the Jumpstarting Brain Tumor Drug Development Coalition Imaging Standardization Steering Committee, Consensus recommendations for a standardized Brain Tumor Imaging Protocol in clinical trials
[3] Jakub Nalepa et al., Deep learning automates bidimensional and volumetric tumor burden measurement from MRI in pre- and post-operative glioblastoma patients
[4] Read more: Liver and liver tumor segmentation and analysis