Artificial Radiologist – the difference between machine learning and artificial intelligence in healthcare
AI in radiology: is science fiction happening now?
The difference between machine learning (ML) and artificial intelligence in healthcare (AI) is frequently misunderstood by many people. Especially, when it comes to AI in radiology. The vision of AI promoted in science fiction and marketing slogans inflates the expectations towards current ML and medical algorithms development. We hear about AI beating humans in complicated games like Go [1] or StarCraft [2]. Moreover, about AI driving autonomous vehicles; about artistic AI composing music [3] and poems [4]; or recently about humanoid AI-driven Tesla Bots [5]. All these applications are great achievements, but they are nowhere near being “intelligent”. Just like ML doesn’t deserve to be called AI. I’d like to present a hypothetical story of “artificial radiologist” DrEddy and human radiologist Adam working in the same hospital in 2022. The story is based on several years of my cooperation with machine learning and radiology experts in Graylight Imaging projects.
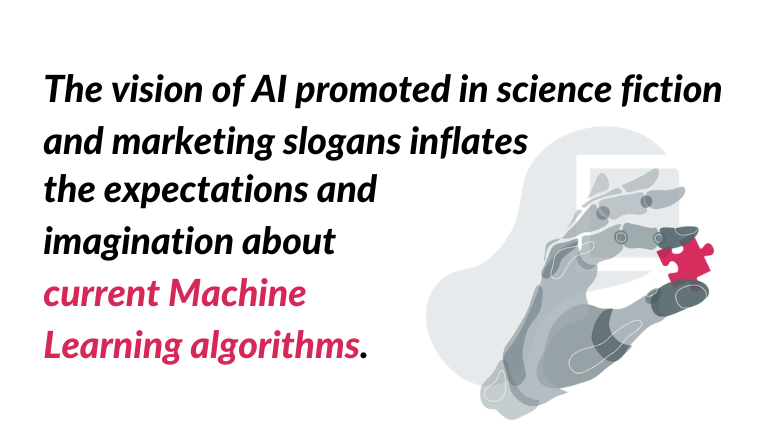
AI in radiology: measurement accuracy vs human perspective
Undeniably, we’re already technically capable of creating DrEddy using state-of-the-art ML algorithms. In ML technique a computer analyzes a massive amount of data (i.e., thousands of medical reports). It finds patterns connected with specific tasks during a process called training. At this point, DrEddy resembles a young resident radiologist gaining experience by being guided by a senior radiologist. However, for DrEddy it takes days instead of years to master competencies. Additionally, we have evidence that DrEddy provides similar accuracy in diagnosis [6] or even outperforms Adam in tasks like tumor segmentation [7][8], lung cancer screening [9], or predicting patient outcomes [10]. DrEddy is CE and FDA certified, works on a 24/7 shift, gives faster and more repeatable diagnoses than Adam. DrEddy has a powerful language module GPT-3 [11]. It can analyze documentation or write precise screening reports. Some people in the hospital management question the need to employ Adam.
The true understanding of AI in healthcare
Fortunately, Adam knows the difference between AI and ML and gives them a convincing list of 4 reasons. Let’s dig deeper into each of them to better understand the benefits and drawbacks of AI in radiology.
The first, and maybe the most crucial limitation of DrEddy is that it does not understand the essence of the disease it is diagnosing. It is trained on a big but limited number of imaging samples from a specific group of patients. It makes DrEddy helpless when diagnosing rare or atypical cases. Moreover, the complexities of human anatomy are infinite, and there will be always cases that do not fit into DrEddy’s patterns. The human radiologist has a true understanding of the living organism behind the images while machine learning (or artificial intelligence in healthcare) is a mindless pattern comparator.
AI in radiology: intuition is key
There are cases in radiology that are not well-defined. Sometimes it is necessary to perform multiple different imaging procedures to find the source of the problem, and there is no algorithm to follow. Lastly, it is not feasible nor safe for the patient to perform all possible scans. Therefore, the radiologist has to follow the intuition which is a concept unreachable for DrEddy.
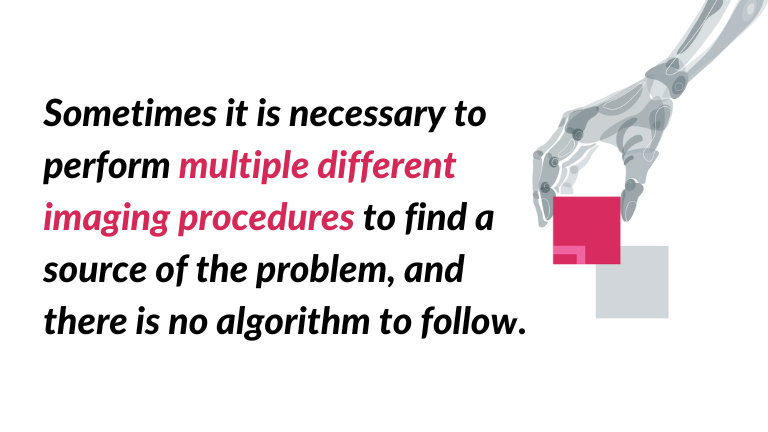
Empathy and patient-centered radiology
Different patients have different levels of emotional resilience and different mental preparation for medical treatment. A good human radiologist may assess it during the interview, and adjust the diagnostic procedures and the way he informs the patient about the results of the examination to improve the psychological comfort of the patient. DrEddy is not able to tell if someone has claustrophobia, autism, depression, or is just scared of the procedure without understanding the emotions of the patient. AI in radiology excels at automatically recognizing complex patterns but falls short in empathy.
AI in radiology requires responsibility and self-awareness
Finally, we need to consider the legal and ethical aspects of DrEddy and artificial intelligence in healthcare work. We verify the results of our models with a group of experienced radiologists in the Mean Opinion Score procedure (for more click here). There are always some cases where experts do not agree about the diagnosis. Therefore, there is a high chance that DrEddy also won’t give a perfect diagnosis every time. Who would be responsible for any medical errors caused by DrEddy? The programmers? It may be very hard to resolve. What if it turns out that the error was caused in the data by the “teacher” of DrEddy? No matter the answer, DrEddy itself cannot be penalized in any way besides turning it off. It is not aware of the consequences of its actions. Should we even allow DrEddy to decide about the health and life of the patient?
Cooperation between Adam and DrEddy
This list was enough for the hospital to keep Adam. The management and Adam agreed that the cooperation between Adam and DrEddy is crucial. This symbiosis joins the advantages of both human and ML, …at least until Artificial General Intelligence (AGI). AGI is a term for a “strong” AI (assuming ML as a “weak” AI) which describes the ability of a machine to simulate consciousness, feelings, and self-awareness. The AGI module would allow DrEddy to think, feel, and create a list of reasons for firing Adam. However, over 40 institutions are researching AGI [12], but it will stay a sci-fi domain for at least a couple of years. Fortunately, we should say, because scientists such as Stephen Hawking warned that AGI is probably the greatest danger for humanity. Thus, organizations like OpenAI launched to promote responsible AI development. When it comes to AI in radiology this responsibility is crucial.
References:
[1] D. Silver et al., “Mastering the game of Go with deep neural networks and tree search,” doi: 10.1038/nature16961.
[2] O. Vinyals et al., “Grandmaster level in StarCraft II using multi-agent reinforcement learning,”doi: 10.1038/s41586-019-1724-z.
[3] “Musicians Are Using AI to Create Otherwise Impossible New Songs,” Time. https://time.com/5774723/ai-music/ (accessed Sep. 26, 2021).
[4] “This AI Poet Mastered Rhythm, Rhyme, and Natural Language to Write Like Shakespeare,” https://spectrum.ieee.org/this-ai-poet-mastered-rhythm-rhyme-and-natural-language-to-write-like-shakespeare (accessed Sep. 26, 2021).
[5] B. Gomez, “Elon Musk warned of a ’Terminator’-like AI apocalypse — now he’s building a Tesla robot,” https://www.cnbc.com/2021/08/24/elon-musk-warned-of-ai-apocalypsenow-hes-building-a-tesla-robot.html (accessed Sep. 26, 2021).
[6] T. S. Cook, “Human versus machine in medicine: can scientific literature answer the question?,” doi: 10.1016/S2589-7500(19)30124-4.
[7] J. Nalepa et al., “Fully-automated deep learning-powered system for DCE-MRI analysis of brain tumors,” doi: 10.1016/j.artmed.2019.101769.
[8] J. R. Mitchell et al., “Deep neural network to locate and segment brain tumors outperformed the expert technicians who created the training data,” doi: 10.1117/1.JMI.7.5.055501.
[9] D. Ardila et al., “End-to-end lung cancer screening with three-dimensional deep learning on low-dose chest computed tomography,” doi: 10.1038/s41591-019-0447-x.
[10] J. Lee, “Is Artificial Intelligence Better Than Human Clinicians in Predicting Patient Outcomes?,” doi: 10.2196/19918.
[11] T. B. Brown et al., “Language Models are Few-Shot Learners,” ArXiv200514165 Cs, Jul. 2020, Accessed: Sep. 26, 2021. [Online].
[12] S. Baum, “A Survey of Artificial General Intelligence Projects for Ethics, Risk, and Policy,” doi: 10.2139/ssrn.3070741.