Segmenting pediatric optic pathway gliomas from MRI using deep learning – our contribution
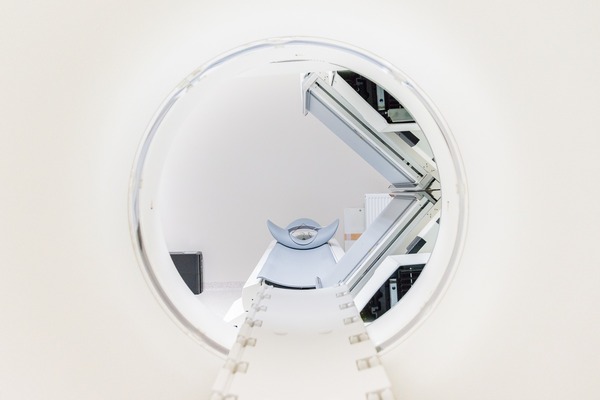
One of our historically most important areas of technological interest is brain imaging. Many of our research publications (link) have been in this area. In the last issue of ‘Computers in Biology and Medicine’[1] we published an article in which together with physicians from Children’s Memorial Health Institute in Warsaw and Division of Diagnostic Imaging, Department of Radiology and Nuclear Medicine, Faculty of Medical Sciences in Katowice (Medical University of Silesia in Katowice) we benefit from our experience in MRI analysis of adult brain gliomas (LGG/HGG) and developed medical algorithm to segment pediatric optic pathway gliomas from MRI.
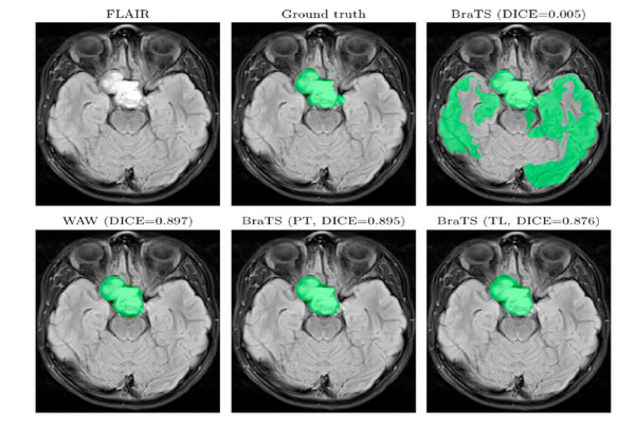
This figure comes from: J. Nalepa, S. Adamski, K. Kotowski, S. Chelstowska, M. Machnikowska-Sokolowska, O. Bozek, A. Wisz, E. Jurkiewicz: Segmenting pediatric optic pathway gliomas from MRI using deep learning. Computers in Biology and Medicine, Volume 142, 2022, 105237, ISSN 0010-4825, https://doi.org/10.1016/j.compbiomed.2022.105237.
Pediatric optic pathway gliomas
Optic pathway gliomas (OPG) or optic nerve gliomas account for 3–5% of all pediatric central nervous system tumors. Although optic pathway gliomas are low-grade tumors, their behavior can be aggressive and lead to loss of vision[2]. Diagnosis is suspected on MRI scan, however yet it is a ‘challenging task due to the difficult and error-prone process of manual segmentation of such lesions, as they can easily manifest different location and appearance characteristics’[3].
Technology that assists radiologists in analyzing imaging studies could significantly impact the therapy and diagnostic process, but this area is not currently being explored intensively.
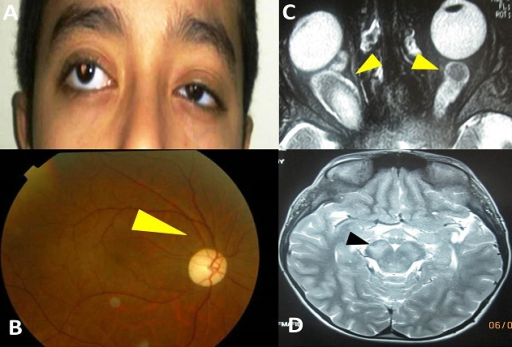
Handor H., Laghmari M., Hafidi Z., Daoudi R.: Optic nerve glioma revealed a divergent strabismus, The Pan African medical journal, 2014, A: strabisme divergent et exophtalmie droite; B) atroph | Open-i (nih.gov).
Segmenting pediatric optic pathway gliomas from MRI using deep learning – our contribution
In the published paper, the authors propose a deep learning algorithm, together with an array of its training strategies, to segment OPGs from MRI scans. Importantly – one of the emerging barriers is of course the very limited amount of data. Despite this factor, they show how to maintain high generalization capabilities.
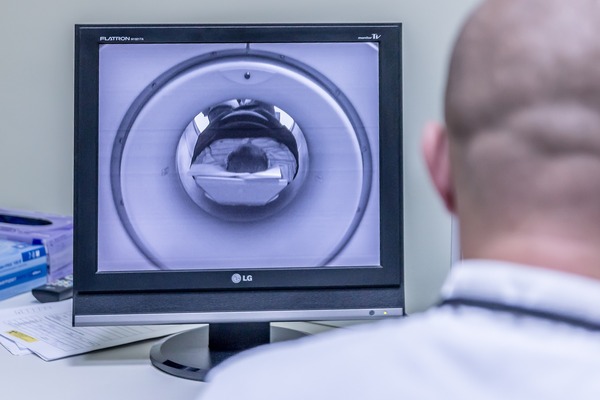
The work of our deep learning researchers and the experiment conducted represents our contribution to trying to build a technology that would answer the diagnostic problems associated with this type of glioma in children.
First, to the best of our knowledge, this is the first publication investing qualitatively and quantitatively the cross-task (OPG vs. LGG/HGG segmentation) generalization capabilities of such deep learning techniques.
Secondly, all models presented in this paper are built on the publicly available nnU-Net framework (https://github.com/MIC-DKFZ/nnUNet), which we believe has a positive impact on democratizing access to knowledge and techniques in deep learning development. Additionally, this framework is an industry-recognized reference in the context of adult LGG/HGG dedicated models – the nnU-Net segmentation engine took first place in the BraTS 2020 competition [4].
Third and finally, the authors performed rigorous multi-fold validation of the proposed techniques – which was backed up with thorough statistical testing – in order to fully understand their advantages (and potential shortcomings).
Research, development and outcome
Finally, in addition to encouraging you to read the paper presented here, we would like to tempt you to summarize this experiment. It is obvious that developmental research often focuses on the verification of a hypothesis. Our researchers, in addition to such verification, managed to present concrete, tangible results in their experiment.
Outcomes:
- authors investigated 13 nnU-Net models trained (or fine-tuned) with 4 different training samples,
- the proposed training strategies help elaborate well-generalizing deep models even in the case of limited ground-truth data,
- all algorithms’ average end-to-end analysis time (of a single MRI) amounted to less than 10 s for each model per patient,
- proposed techniques offer real-time operation,
- proposed models are able to accurately delineate OPGs that manifest various appearance and location characteristics,
- authors’ technique can not only effectively delineate optic pathway gliomas, but can also be applied for detecting other brain tumors such as low- and high-grade gliomas in adults.
Research and its follow-up
The experiment does not conclude the topic, of course. The processing chain still requires clinical validation over more heterogeneous MRI data. In our view, however, introducing an automated OPG delineation procedure into the OPG management and treatment process would allow us to design more objective treatment pathways for such patients.
References:
[1.] J. Nalepa, S. Adamski, K. Kotowski, S. Chelstowska, M. Machnikowska-Sokolowska, O. Bozek, A. Wisz, E. Jurkiewicz: Segmenting pediatric optic pathway gliomas from MRI using deep learning. Computers in Biology and Medicine, Volume 142, 2022, 105237, ISSN 0010-4825, https://doi.org/10.1016/j.compbiomed.2022.105237.
[2] I. Fried, U. Tabori, T. Tihan, A. Reginald, E. Bouffet, Optic pathway gliomas: a review, CNS oncology 2 (2) (2013) 143–159, https://www.ncbi.nlm.nih.gov/pmc/articles/PMC6169473.
[3] J. Nalepa, S. Adamski, K. Kotowski, S. Chelstowska, M. Machnikowska-Sokolowska, O. Bozek, A. Wisz, E. Jurkiewicz: Segmenting pediatric optic pathway gliomas from MRI using deep learning. Computers in Biology and Medicine, Volume 142, 2022, 105237, ISSN 0010-4825, https://doi.org/10.1016/j.compbiomed.2022.105237.
[4] F. Isensee, P.F. J¨ager, P.M. Full, P. Vollmuth, K.H. Maier-Hein, nnU-Net for brain tumor segmentation, in: A. Crimi, S. Bakas (Eds.), Brainlesion: Glioma, Multiple Sclerosis, Stroke and Traumatic Brain Injuries, Springer International Publishing, Cham, 2021, pp. 118–132.
See the previous post by Anna Choma: Graylight Imaging Data Scientists top ranked in RSNA-ASNR-MICCAI 2021 Brain Tumor Segmentation (BraTS) Challenge