Radiology AI Landscape in numbers (and money)
Artificial Intelligence finds utility across various stages of medical treatment, with its principal applications encompassing clinical image acquisition and processing, the implementation of machine learning techniques, and the development of AI algorithms for the prediction and classification of diseases and disorders [1]. Likely, as a result of this, radiology is emerging at the forefront when it comes to the use of AI in medicine. But do the numbers truly confirm this? Recently, several interesting studies have been published on this topic, and this post aims to briefly discuss them.
How do radiologists use AI in clinical practice? Surveys
In 2020 the ACR Data Science Institute conducted its first annual survey of ACR members to learn how radiologists use AI in their clinical practice. It found that approximately 30% of radiologists were using AI as part of their practice, and of practices not using AI, 20% planned to purchase AI tools in the next 1 to 5 years [2]. But what does it look like in 2023?
When discussing the number of AI solutions currently used in radiology, a recent publication by Radboud University Medical Center researchers comes to mind. [3] During this research, the authors observed the practical application of commercially accessible AI software in radiology units within the Netherlands, spanning a duration of three years, from 2020 to 2022. Conclusions? The number of health departments utilizing artificial intelligence has steadily increased, with at least one-third of the centers utilizing AI in clinical practice in the Netherlands by 2022. However, interestingly, seven implementations were discontinued in 2022.
Research mentioned above found that AI is predominantly utilized to support the analysis of
- chest CT scans – for tasks such as nodule detection, pulmonary embolism identification, and assessing COVID-19 severity,
- neuro CT scans – including the detection of large vessel occlusions, intracranial hemorrhages, Alberta Stroke Program Early CT Score (ASPECTS) evaluation, and CT perfusion (CTP) analysis,
- and musculoskeletal radiographs – encompassing tasks such as bone age estimation, fracture detection, and automated extremity measurements.
In conclusion, there has been a consistent upward trajectory in the adoption of artificial intelligence within healthcare departments, with the presence of AI in clinical practice observed in at least 1/3 of the healthcare centers across the Netherlands. There is a possible bias in this research, nevertheless, the presented trend highlights the growing integration of AI as a valuable tool in modern healthcare settings.
The dilemma of AI adoption in radiology
Even with the increasing number of implementations in the clinical environment, it is worthwhile to pause and consider the obstacles in utilizing AI.
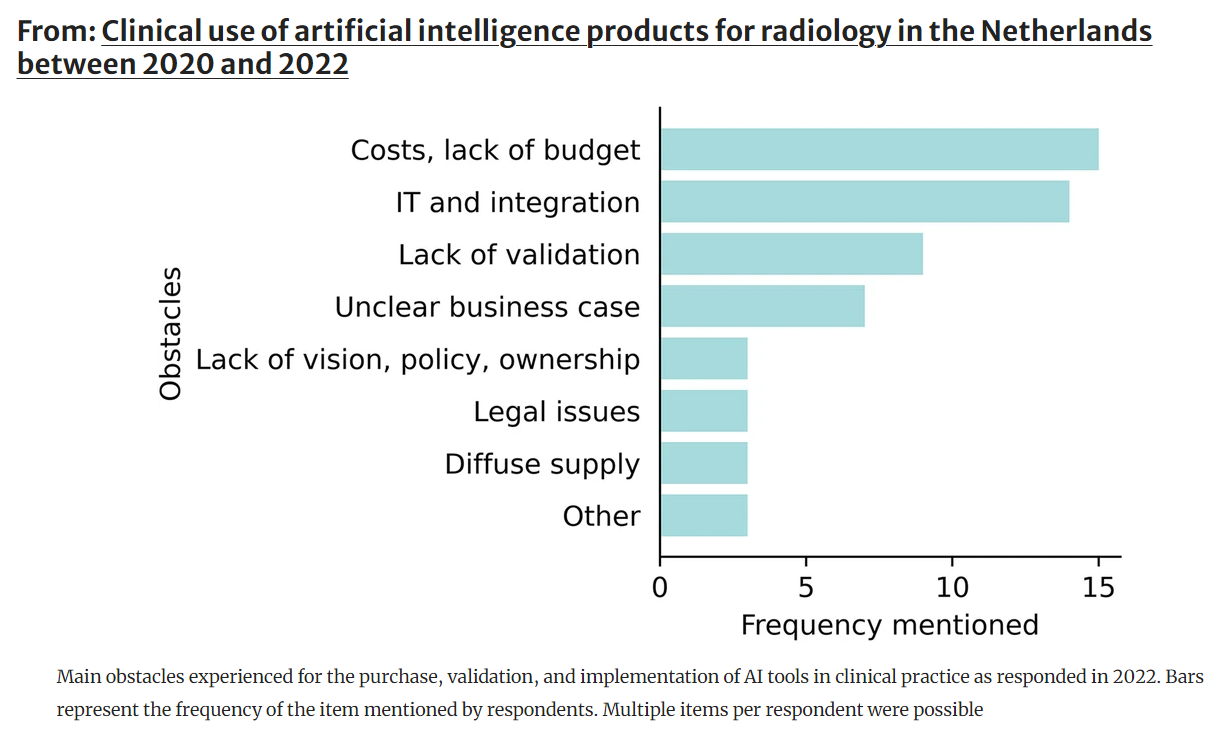
Source: van Leeuwen, K.G., de Rooij, M., Schalekamp, S. et al. Clinical use of artificial intelligence products for radiology in the Netherlands between 2020 and 2022. Eur Radiol (2023). https://doi.org/10.1007/s00330-023-09991-5
Interestingly, lots of respondents focused (except for the money issue, of course) on validation. It seems that the primary challenge associated with integrating AI into healthcare lies in the lack of confidence among users (and maybe payers?). This trust deficit [4] may arise because the AI’s performance in medical tasks and its information processing methods remain hidden from these stakeholders. Furthermore, many AI systems possess self-learning capabilities and the ability to adapt their working algorithms. As a result, the entire process may not be entirely transparent or understandable. And you don’t want to pay for something that you don’t even understand.
To pay or not to pay for AI in radiology?
Therefore, let’s circle back to money. The respondents cited budget constraints as the primary barrier to adopting AI in clinical practice. Will high purchase and implementation costs remain the major obstacles holding back AI adoption? As Franziska Lobig et al. aptly noted, while the payment structures for radiology AI applications are still emerging, some radiology AI applications are already subject to separate payments in the United States (US).
Similarly, the National Health Service (NHS) in the United Kingdom (UK) and the National Health Insurance system of Japan also have separate money arrangements for radiology software applications provided as Software-as-a-Service (SaaS) [5]. The article advocates, that it is critical for payers to develop a clear set of criteria for determining which AI applications for radiology should be paid for separately and be considered as an independent expense when compared to the cost of medical imaging studies by themselves. In their research, Lobig et al. proposed a framework to determine this.
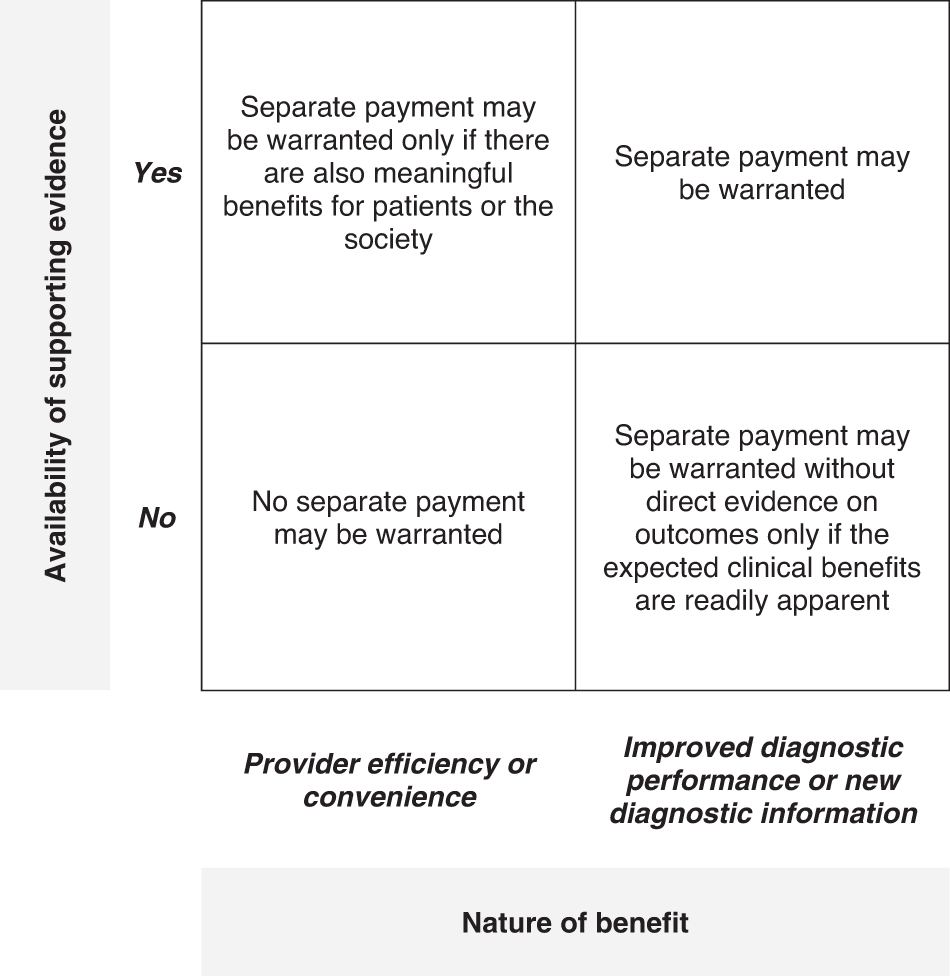
Source: Lobig, F., Subramanian, D., Blankenburg, M. et al. To pay or not to pay for artificial intelligence applications in radiology. npj Digit. Med. 6, 117 (2023). https://doi.org/10.1038/s41746-023-00861-4
This also underscores the importance of reimbursement policies. Clinical guidelines and reimbursement policies play a pivotal role in shaping the adoption of AI in healthcare by directly influencing financial incentives for healthcare providers. Furthermore, well-designed reimbursement policies can encourage innovation and the development of AI solutions tailored to address specific healthcare challenges, ultimately enhancing patient care and outcomes.
Since reimbursement can be a critical determinant of AI adoption in healthcare, we all must engage in discussions on evidence requirements for these applications, streamlining processes to democratize access to proven state-of-the-art innovations for all.
References:
[1] Bitkina OV, Park J, Kim HK. Application of artificial intelligence in medical technologies: A systematic review of main trends. Digit Health. 2023 Jul 18;9:20552076231189331. doi: 10.1177/20552076231189331. PMID: 37485326; PMCID: PMC10359663.
[2] Allen B, Agarwal S, Coombs L, Dreyer K, Wald C (2021) 2020 ACR Data Science Institute Artificial Intelligence Survey. J Am Coll Radiol 18(8):1153–1159. https://doi.org/10.1016/j.jacr.2021.04.002
[3] van Leeuwen, K.G., de Rooij, M., Schalekamp, S. et al. Clinical use of artificial intelligence products for radiology in the Netherlands between 2020 and 2022. Eur Radiol (2023). https://doi.org/10.1007/s00330-023-09991-5
[4] Check our post on how the lack of conclusive evidence is also reflected in public opinion (six out of ten American adults say they would be concerned if their healthcare provider used AI): https://site.graylight-imaging.com/ai-in-healthcare-news-picked-by-gli-02/
[5] Lobig, F., Subramanian, D., Blankenburg, M. et al. To pay or not to pay for artificial intelligence applications in radiology. npj Digit. Med. 6, 117 (2023). https://doi.org/10.1038/s41746-023-00861-4