Research: Pelvic bones fracture examination based on CT scans
Pelvic fractures: beyond the break
Around 8-9% of blunt force injuries involve the pelvis. This type of injury is common in blunt trauma cases, such as car and motorcycle accidents, bicycle crashes, and pedestrian collisions with vehicles.
This percentage may seem relatively small. However, pelvis injuries are of key interest in treatment. The fractures in the pelvic region may lead to life-long disability. Importantly, this is not the only factor that needs to be considered.
Because of its structure, if one part of the pelvis breaks, it’s probable that another part will break as well. The force of the impact that caused the first break is likely to travel through the bone. Eventually, it may cause a second fracture in a nearby area. [1].
How can technology help in pelvic fractures cases?
As you can see, the level of complexity of pelvic injuries is extremely high. Knowing this, clinicians always carefully examine a patient with a pelvic fracture. Moreover, the pelvis is made up of several sections. The type of treatment a patient needs will depend on which specific section is injured.
In one of our projects, we had to develop an algorithm for pelvis bone classification and segmentation. We believe that the automation of CT scan assessment can improve diagnostic sensitivity. The use of AI algorithms for the medical image analysis has yielded excellent results in many therapeutic areas. Our team hoped that this would also be the case in this instance.
Interactive graphics – rotate, zoom in, zoom out.
Breaking through complexity: pelvic fractures AI project
Firstly, we had to properly address the problem of pelvic bone classification and segmentation (sacrum, left pelvic bone, right pelvic bone, and lumbar spine) in trauma patients. We collected 103 CT scans and segmented bones in those cases. Using U-net-like architecture, we distinguished the pelvic bones and pelvic fractures. As the fractures are mostly due to communication accidents, they are classified as complete with comminuted breakage. In CT, we can see many small bones being displaced near pelvic bones. The most challenging part lies in the correct classification of tiny bones in the close vicinity of displacements of different bones.
From CT scans to solutions
From the dataset 21 samples were selected for the test set. We equipped the final model with dedicated postprocessing. We wanted to improve its discriminating ability towards the left and right pelvic bones. This was crucial as part, because with rich augmentation in network training, some small pieces of bones might be undistinguishable for the model (loss of symmetry or ambiguous parts of ilium).
How did we assess AI performance?
To assess the model performance we used DICE, recall, and precision metrics, respectively.
- Recall is a metric that enables us to answer a very important question. How good a model is at finding all positive cases? It’s the true positives divided by all actual positives.
- Precision measures how often an ML model is correct. It allows for the assessment of the accuracy of positive predictions.
- Lastly, Dice score compares the similarity between two datasets (masks, image segmentations).
For each metric, we got at least 0.9 an average score with almost every case having a few fractures.
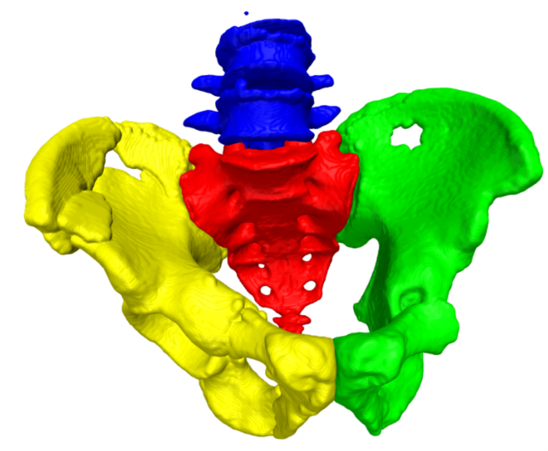
Figure 1: Properly classified and segmented pelvic bones. Yellow – right pelvic bone, green – left pelvic bone, red – sacrum, blue – lumbar spine. In the picture, we can see a number of fractures in the ilium part of the pelvic bones.
References: