PET/CT in lung cancer analysis assisted with radiomic-based features
Lung cancer analysis: the global killer
Lung cancer constitutes 12.7% of the global cancer incidence rate. The staging and diagnosis process heavily relies on PET/CT. By offering tumor details, powerful quantitative approaches like CT texture analysis can improve the diagnostic value of PET/CT. However, in busy clinics where analysis is still laborious and prone to human mistakes, such procedures are impractical.
To quantify the characteristics of lung cancer
Improving lung cancer diagnosis from medical image analysis is a key factor in the screening process or monitoring of the disease. Choosing the right imaging modality (PET vs. CT scan or others) depends on several factors. Positron emission tomography (PET) measures cellular function. It shows how active different tissues are by detecting the level of a radioactive tracer absorbed by cells. On the other hand, computer tomography (CT) creates detailed images of anatomy. Moreover, there is also a PET/CT scan combining both technologies into one exam. It provides information on both structure (CT) and function (PET) in a single session.
Lung cancer analysis: choosing PET/CT
Although structural tumor information can be observed in structural imaging, such as computed tomography (CT)… Understanding the physiological activity in functional imaging may be pivotal to perform malignancy of the tumor differentiation. As an example, positron emission tomography (PET) allows us to measure the glucose uptake. It indicates the metabolism of the tissue and may help us identify active lesions (unfortunately, PET images are of poor spatial resolution, and they do not reveal much of the anatomical details). CT scans are used to verify the hot spots based on the human body atlas. However, they also can serve as an exciting source of “invisible” information about the lesion. We wanted to quantify the characteristics of the tumor (e.g., its volume), or extract additional information which may not be visible to the naked eye.
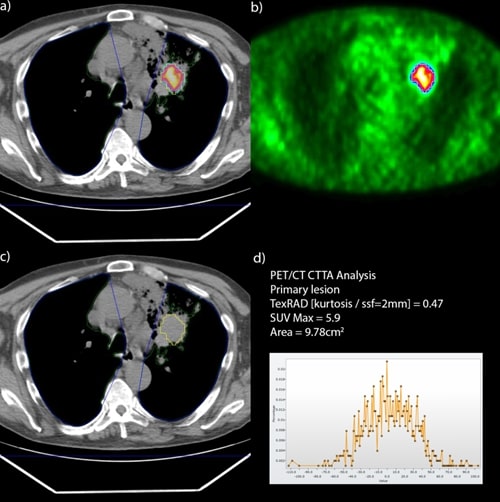
Figure 1: Extracting radiomic-based features from high-uptake lesions requires their accurate delineation – they are clearly visible in b) PET, and once they are segmented there, they may be easily transferred to the a)-c) co-registered CT to extract d) “invisible” textural information from CT.
Our approach, referred to as LUNGCX, required no user intervention and was 100% repeatable – starting from the segmentation of lungs in CT [1], delineating lesions in the lung areas in PET through fairly simple thresholding, and extracting biomarkers from CT using texture analysis.
The preliminary study: what we did?
In our study, we wanted to assess the performance of an automated PET/CT analysis in lung cancer and compare it against experienced imaging reporting.
We conducted a study using radiomic features elaborated from the image which could correlate with the patient’s clinical parameters. We have shown that textural parameters can be used to predict the survival of the patient (p=0.028) – the filtration-histogram technique [2] was utilized to reveal such image features.
Results
We presented the results at RSNA 2016 [3] in an Informatics poster discussion. You may find the publication online: Jakub Nalepa et al: “PET/CT in Lung Cancer: An Automated Imaging Tool for Decision Support”, Proc. Radiological Society of North America Meeting (RSNA), Chicago, USA, 2016.
References
[1] Our segmentation approach was described in more detail in the article: Jakub Nalepa, Michal Czardybon, Maksym Walczak: „ Real-time lung segmentation from whole-body CT scans using Adaptive Vision Studio: a visual programming software suite”, Proc. SPIE Real-Time Image and Video Processing 2018 10670, 93-103, 2018.
[2] TexRAD Ltd, www.texrad.com, part of Feedback Plc, Cambridge, UK