POC: Limit of Detection in PCR
Challenge: a new approach to PCR test’s measurement
The polymerase chain reaction (PCR) [1] technique is widely used to rapidly make millions to billions of copies of a specific RNA/DNA sample. The procedure takes place in a PCR chamber and, in many cases, relies on thermal cycling. By attaching fluorophores to the samples, we can monitor the outcome by using a digital camera. The global image intensity indicates the reaction progress over a short period of time. However, sometimes one might face the issue of the limit of detection PCR. In some cases, the global signal changes are below the detection limit, especially when the process is started with a small number of DNA molecules. In this case, one can search for a location within the chamber and measure the growth process based on the local signal.
The experimental project: limit of detection PCR
At Graylight Imaging we specialize in medical image analysis. In one of our proof of concept projects we automatized the detection of such places using signal processing techniques. Observing their signatures in 3d time-space suggests a clear pattern for further application of robust machine learning methods.
Case 1 – signal is above level of detection
PCR chamber with large number of DNA molecules gives a strong and easy to detect signal which can be observed using illumination of the fluorescent dye. In the example below the incubation process involved approximately 100 DNA molecules. Their proliferation is clearly visible with the naked eye and the time series of average value of an image provides a good measure of the kinetics of the process.
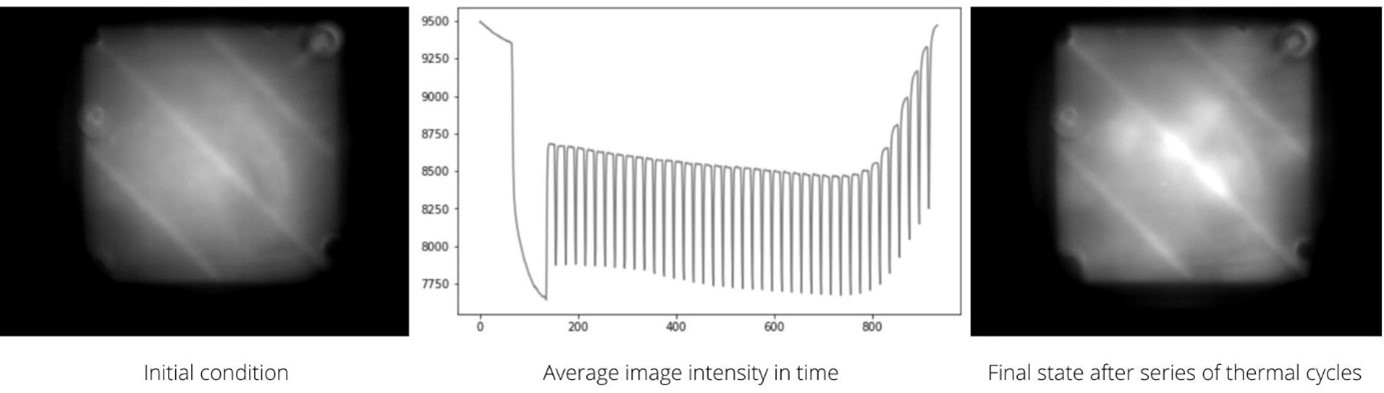
Case 2 – the signal is below the level of detection
If the initial number of DNA molecules is smaller, the signal might become very difficult to observe. In the example below the final state differs by very little detail from the initial one. The time series of the average luminosity of the whole chamber does not exhibit any growth process.
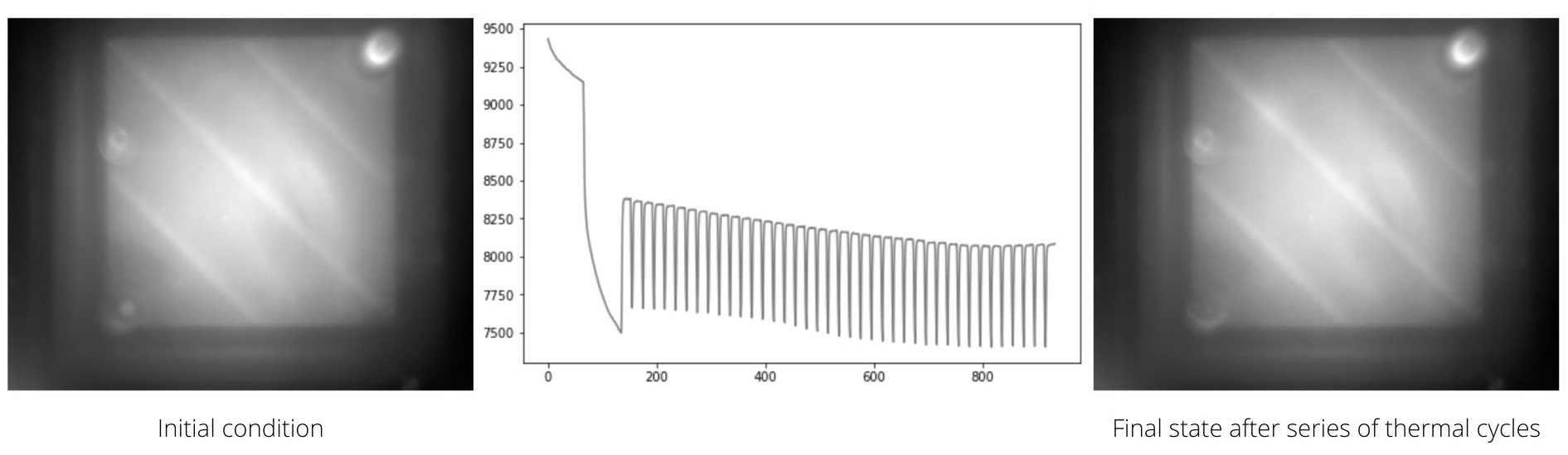
Can we obtain valid measurement in case 2?
We used signal analysis to detect an area where a signal grows locally. The average signal in this area indeed exhibits clear growth.
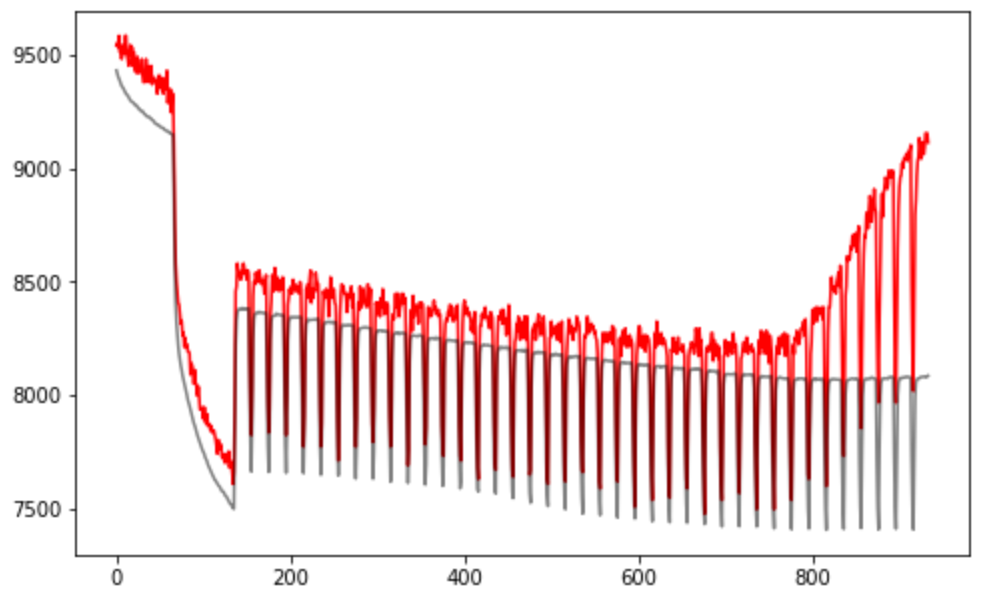
Measurement of local changes in the luminosity of PCR chamber as a function of time. Despite of lack of an amplification in a global signal – the local average exhibits clear growth.
How to automatically detect regions of interest which can lead to correct measurement?
If we treat time as a third dimension, the time series of image frames compose a 3d volume. For improved analysis it is better to take into the account a difference between subsequent local maxima of the 2d image frames. In 3d space in case 2 we have following picture:
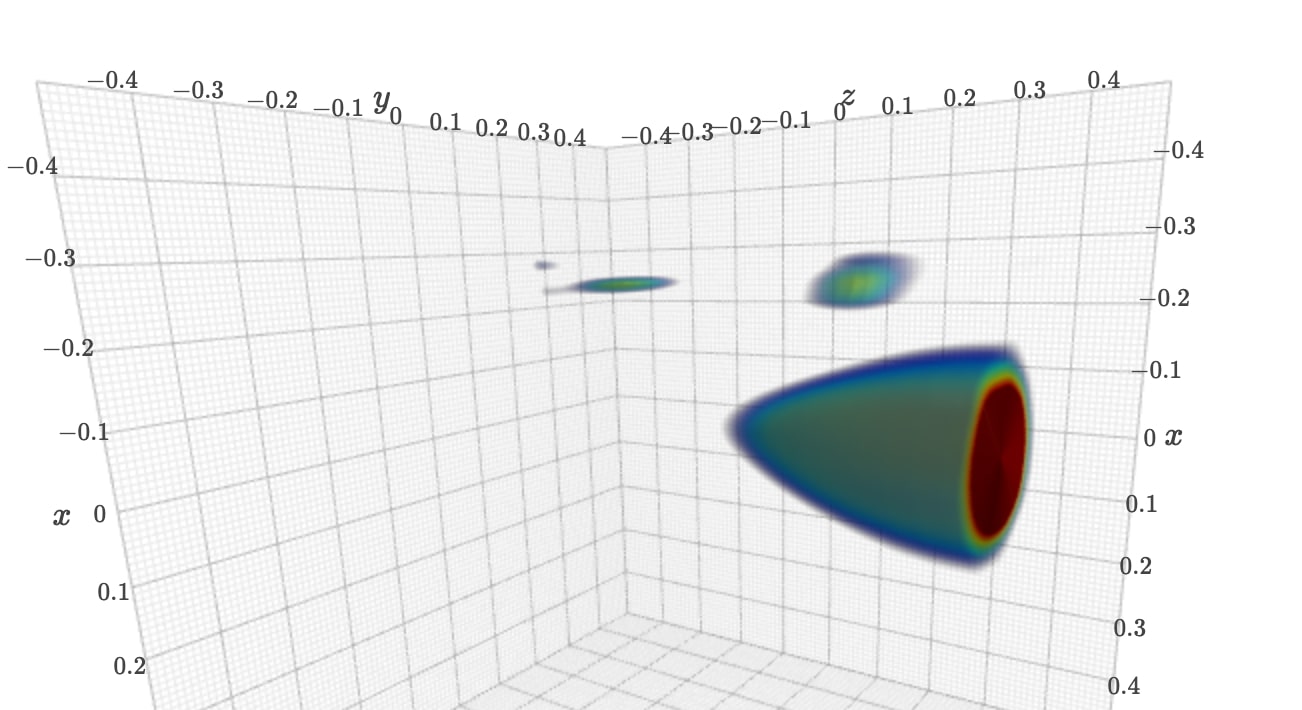
Time-space representation of differences of acquired images.
We might notice that the growth process in this picture looks like a paraboloid. We can easily distinguish if from possible distortion which can take place during the experiment.
Further, we can use Machine Learning to detect those structures, which in turn automatically serve as regions of interest for analysis of the kinetics of the PCR chamber process.
References: