Automated RANO solution for clinical trial
Automated Response Assessment in Neuro-Oncology criteria?
In 2010 the medical community introduced the Response Assessment in Neuro-Oncology criteria (RANO). The goal was to establish trustworthy and widely used response criteria and clinical trial endpoints for CNS tumors. This has created opportunities for clinicians to provide repeatable measurements of “measurable” and “non-measurable” tumors.
Nowadays, most frequently, semi-automated RANO criteria are present in a clinical workflow. Although attempts to fully automate this process have been made, some of the solutions have resulted in overestimated volumes. But is it impossible? As a company specializing in medical algorithm development, we decided to say: check.
The automated RANO solution we needed
In a project for a drug company, we built a system for automated identification and segmentation of different tumor regions. It was supposed to work for glioma patients both before and after surgery. A part of that challenge involved developing a trustworthy automated RANO algorithm.
Our solution accurately segmented brain scans from glioma patients (before and after surgery) much faster (up to 20x) than manual methods. The algorithm performed over 760 pre-operative and 504 post-operative adult patients with glioma. We obtained data from the public database (acquired at 19 sites) and from a clinical treatment trial.
However, there were some challenges we had to overcome.
Ground truth preparation might be a challenge
We developed and followed a strict manual annotation procedure to guarantee the high quality of manual ground-truth delineations. Later, we used them to build a deep learning segmentation model. Moreover, we had to face the inter-rater variability among human readers for the bidimensional RANO calculation in the post-surgery images. Reader disagreements limits the ability to detect a true patient response. Not to mention, to capture early patient response or progression in clinical practice.
We managed to lessen this variability. Our automated pipeline reduced inconsistencies between doctors in measuring tumor response after surgery.
Automated RANO: what we did
We developed two automated RANO solutions:
- The first one computes the product of the perpendicular diameters discovered through the analysis over all slices while adhering to strict RANO criteria.
- The second one thoroughly optimizes the diameters’ product rather than their maximum length. This method, according to our research, is less sensitive to subtle changes and alterations in the contour of the lesions.
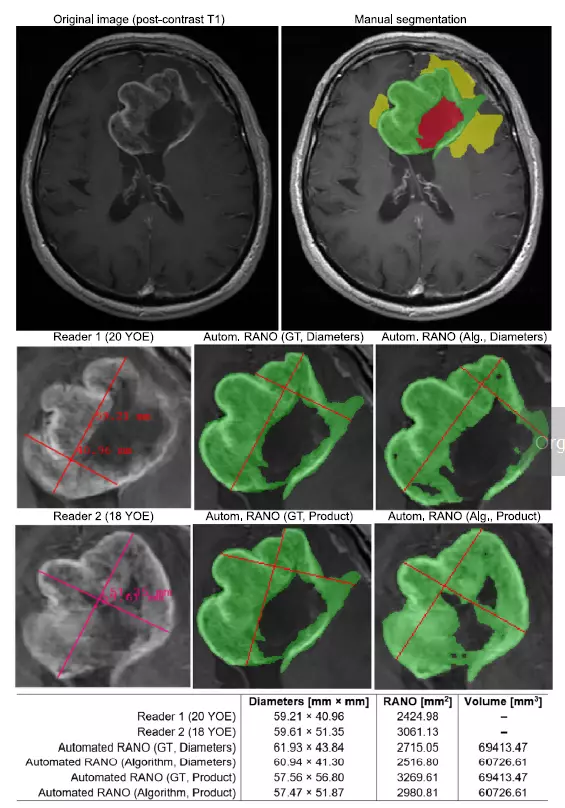
Qualitative and quantitative analysis can reveal important aspects concerning the behavior and abilities of the suggested pipeline—an example axial post-contrast T1 image and manual segmentation (green—ET, yellow—ED, red—surgical cavity). Below, the RANO bidimensional measurements for the two most experienced readers compared with Automated RANO (Diameters) and Automated RANO (Product) obtained from either the ground-truth or the algorithm’s segmentation. Manual calculation of RANO is subjective, difficult to reproduce and may easily lead to high inter-rater disagreement—the proposed algorithm offers full reproducibility and a more accurate optimization of the product diameters leading to a stronger correlation with the ET volume.
Image comes from: Jakub Nalepa, Krzysztof Kotowski, Bartosz Machura, Szymon Adamski, Oskar Bozek, Bartosz Eksner, Bartosz Kokoszka, Tomasz Pekala, Mateusz Radom, Marek Strzelczak, Lukasz Zarudzki, Agata Krason, Filippo Arcadu, Jean Tessier, Deep learning automates bidimensional and volumetric tumor burden measurement from MRI in pre- and post-operative glioblastoma patients, Computers in Biology and Medicine, Volume 154, 2023, https://doi.org/10.1016/j.compbiomed.2023.106603
Let’s talk about algorithm’s performance
Our automated RANO algorithm performed admirably when compared to the most experienced expert readers (ICC: 0.681 and 0.866). We also demonstrated that tumor burden cannot always be determined solely by RANO measurements. Investigating the automatically-extracted volumetric tumor characteristics may easily give more accurate insights into the disease progression.
We believe that due to the automated tumor burden measurement’s high performance, we may add value to patient response assessment. Our automated system measures tumor burden very precisely, improving evaluations for glioblastoma in clinical trials. We also created a new way to calculate RANO (tumor response) that works well even with slightly inaccurate segmentation, making it more reliable.
You may find our processing pipeline, alongside the experimental results in the following paper:
Jakub Nalepa, Krzysztof Kotowski, Bartosz Machura, Szymon Adamski, Oskar Bozek, Bartosz Eksner, Bartosz Kokoszka, Tomasz Pekala, Mateusz Radom, Marek Strzelczak, Lukasz Zarudzki, Agata Krason, Filippo Arcadu, Jean Tessier, Deep learning automates bidimensional and volumetric tumor burden measurement from MRI in pre- and post-operative glioblastoma patients, Computers in Biology and Medicine, Volume 154, 2023, https://doi.org/10.1016/j.compbiomed.2023.106603