Transparency in medical software: a blueprint for trust and safety
Fostering trust and transparency in medical software is crucial in an era marked by significant advancements in artificial intelligence (AI) and machine learning (ML). These innovations have led to a remarkable transformation in the field.
AI has emerged as a powerful tool in clinical applications, enhancing both diagnostic and therapeutic decision-making. Deep neural networks, driven by improvements in data availability and computational resources, have demonstrated performance that matches or even surpasses that of human clinicians in various tasks. [1]
These technologies hold great promise for enhancing healthcare by providing faster diagnoses and potentially better treatment outcomes. However, with this progress comes an essential need for transparency. [2]
United for transparency in medical software
The U.S. Food and Drug Administration (FDA), in collaboration with Health Canada and the United Kingdom’s Medicines and Healthcare Products Regulatory Agency (MHRA), has released a document “Transparency for Machine Learning-Enabled Medical Devices: Guiding Principles.” These organizations have collaborated to create guiding principles for Machine Learning-Enabled Medical Devices (MLMDs) and, thus medical software development.
This document is based on recognized best practices for using machine learning techniques in medical devices and software as a medical device also. By building on established principles for effective machine learning practices, these guidelines ensure that patients, healthcare professionals, and other stakeholders are well-informed about the functioning of these devices. [3]
We should add that the document is the aftermath of the definition by the institutions, already in 2021, of 10 good machine learning practices for medical device development. [4]
What does transparency mean?
Transparency involves clear communication of a device’s intended use, development process, and performance metrics.
Central to this is “explainability” or the ability to understand how the device arrives at its conclusions. Transparency empowers users to identify risks, comprehend the device’s logic, and make informed decisions, ultimately benefiting both clinicians and patients. For example, a clinician using an AI diagnostic tool needs to understand whether it is meant to augment their expertise or provide definitive answers.
Effective transparency:
- Prioritizes the communication of critical information that could affect patient safety.
- Tailors information delivery to the specific needs and context of the intended user or audience.
- Optimizes communication strategies for maximum impact.
- Leverages a deep understanding of user needs, environmental factors, and workflow considerations. [5]
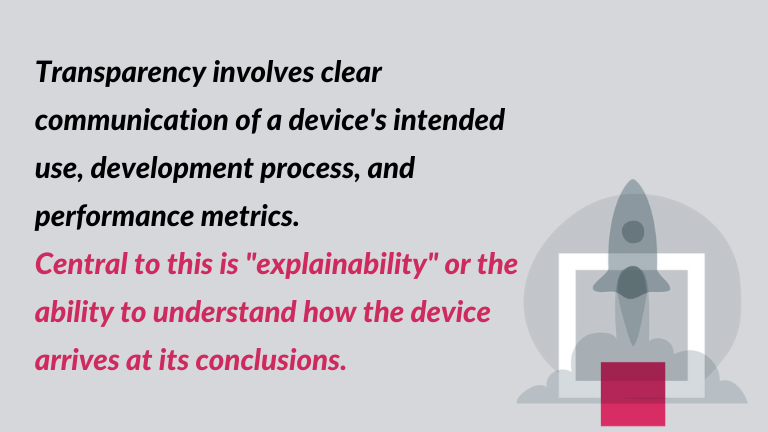
Human-centred design: a path to transparency
Human-centred design is a cornerstone of transparency in medical software and other medical device, focusing on the user experience throughout a medical device’s lifecycle.
This approach can be employed to facilitate the development of medical devices with a high degree of transparency, validate the transparency of such devices, and ensure that users possess all necessary device-related information.
By incorporating feedback from healthcare professionals, patients, and administrators, we can ensure that medical devices based on machine learning are designed to meet real-world needs and improve patient outcomes.
For instance, user interfaces might include visual aids, alerts, or plain-language descriptions tailored to specific audiences. These approaches ensure that healthcare professionals can integrate AI-powered medical devices into their workflows effectively. [6]
Information, timing and communication
Device information should be easily accessible through the user interface, which includes all elements that users can interact with. By optimizing the software interface, we can ensure that the information provided is relevant, timely, and effective. This optimization can be achieved through personalization, adaptability, and interactive features such as audio, video, text, alerts, diagrams, and document libraries.
By communicating effectively throughout a device’s lifecycle, developers can keep users informed about performance, risks, and modifications. Timely updates, such as notifications about software updates or identified biases, can prevent errors and enhance user confidence. [7]
Mitigating risks and building trust in medical devices
A deep understanding of medical devices, regarding also the transparency of medical imaging software’s intended use and limitations, is essential for minimizing risks. By sharing critical information about biases in training data, summaries of clinical studies, and the underlying logic of the model, we empower users to make informed decisions.
For instance, if the training data for a device lacks diversity, transparency allows users to be aware of potential disparities and take steps to address them. Additionally, transparency facilitates proactive risk management, enabling users to identify and resolve issues like performance degradation or bias in real time.
Transparency is the foundation of trust in AI-powered medical devices. By clearly communicating a device’s benefits, limitations, and gaps in data, developers can foster trust among users. Furthermore, transparent practices promote health equity by uncovering potential biases and ensuring inclusivity.[8]
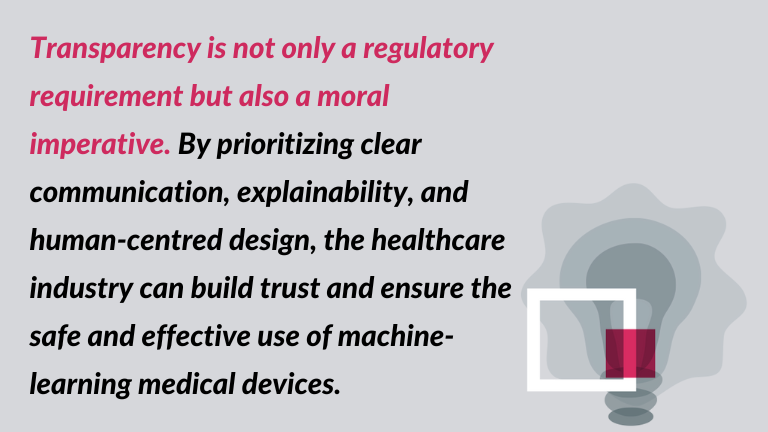
Navigating the path forward
Achieving transparency requires balancing the need to protect proprietary information with the ethical obligation to share critical insights. The dynamic nature of machine learning models necessitates ongoing efforts to keep users informed. Collaboration among developers, regulators, and stakeholders is essential for establishing consistent transparency standards. [9]
A future of trust and innovation
Transparency is not only a regulatory requirement but also a moral imperative. By prioritizing clear communication, explainability, and human-centred design, the healthcare industry can build trust and ensure the safe and effective use of machine-learning medical devices. Continued collaboration will drive the evolution of best practices, supporting the integration of machine learning technologies into patient care and ultimately improving health outcomes for all. [10]
Resources
[1] Zohaib Salahuddin, Henry C. Woodruff, Avishek Chatterjee, Philippe Lambin: Transparency of deep neural networks for medical image analysis: A review of interpretability methods, Computers in Biology and Medicine, January 2022, https://doi.org/10.1016/j.compbiomed.2021.105111.
[2], [3], [5]-[10] Transparency for Machine Learning-Enabled Medical Devices: Guiding Principles, FDA: https://www.fda.gov/medical-devices/software-medical-device-samd/transparency-machine-learning-enabled-medical-devices-guiding-principles.
[4] Good Machine Learning Practice for Medical Device Development: Guiding Principles, FDA: https://www.fda.gov/medical-devices/software-medical-device-samd/good-machine-learning-practice-medical-device-development-guiding-principles.