Manual vs AI-assisted patient response assessment
In recent blog posts, we have been discussing the topic of patient response criteria and measurements. We elaborated on an updated version of the RANO criteria (RANO 2.0). We also asked the question of how well RECIST and RANO are ideal biomarkers of tumor change. Today, we would like to invite you to delve into another, but highly related topic.
We will look at a few clinical trials comparing manual measurements of patient response with those made by AI-powered systems.
iRECIST criteria: manual versus software-assisted
A group of researchers from University Medical Center Hamburg-Eppendorf published in February 2024 a study aimed at a comparison between software-assisted assessments of patient response versus manual approaches [1]. The cohort consisted of people undergoing chemo- or immunotherapy. In these cases, iRECIST criteria applied to all analyzed medical images. Because of the complexity of the assessment researchers evaluated:
- calculated sum of longest diameter (SLD) and the reader agreement,
- error rate,
- and reading time.
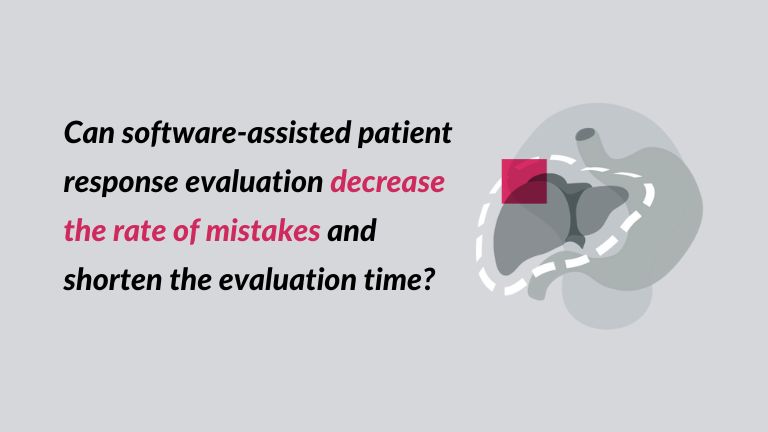
Patient response over time and error rate
When it comes to SLD measurements a quantitative agreement rate was higher in data analyzed by software. When comparing manual analysis to software-assisted ones, the reading time was noticeably greater. Code just works faster than the human eye and hand. How about error rates and reliability? At the first follow-up, some assessments were wrong: 3.3% done by hand, but none with software. This gap widened in the second follow-up: 10% manual errors, but software-assisted stayed low at 1.7%. Can software-assisted patient response evaluation decrease the rate of mistakes and shorten the evaluation time? The study conclusion is clear. Clinical trials can get more accurate results by using software to assess how patients respond to cancer treatment.
Exploring the interobserver variability and agreement
Another study we’d like to present was conducted by researchers from the Department of Medical Oncology at Zhejiang University School of Medicine [2]. The study aimed at the investigation of the reproducibility of a computer-aided contouring tool measurement. Additionally, they wanted to investigate if the aforementioned tool can improve how we track oncological patient response in clinical trials (RECIST 1.1).
The study finding proved a statistically significant reduction in the coefficient of variance in data analyzed with software assistance. This study revealed a significant interobserver variability for manual tumor measurements (2/3 of cases). Conversely, the computer-aided contouring tool achieved twice the better performance.
The software’s key strength lies in its reproducible and consistent segmentation, which substantially reduces interobserver variability in tumor measurement. This, in turn, ensures the quality and repeatability of tumor response evaluation across different radiologists and institutions.
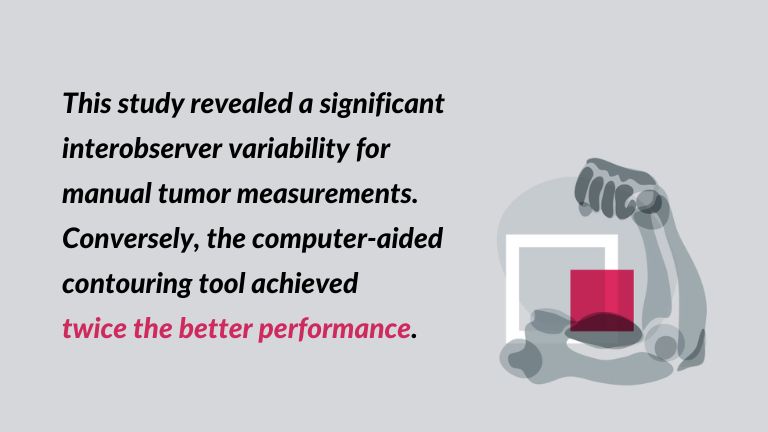
Artificial Intelligence vs radiologists in cancer detection
A separate study in Turkey examined 211 mammograms with AI software [3]. Radiologists identified cancer in 67.3% of cases, while AI achieved 72.7%. 83.6% rate was achieved when both the software and the radiologist interpreted the image data. This might suggest that AI can significantly improve cancer diagnosis in clinical practice and trials.
Just a few days ago, Dutch scientists published a paper summarizing an experiment [4]. They organized a challenge to correctly detect prostate cancer in MRIs, comparing the assessments of radiologists (using PI-RADS 2.1 criteria) with an algorithm.
The AI system not only met the pre-defined standards for performance (AUROC [5] of 0.91), but it also outperformed a group of 62 radiologists in diagnosing cancer at the individual case level. They achieved an AUROC of 0.86.
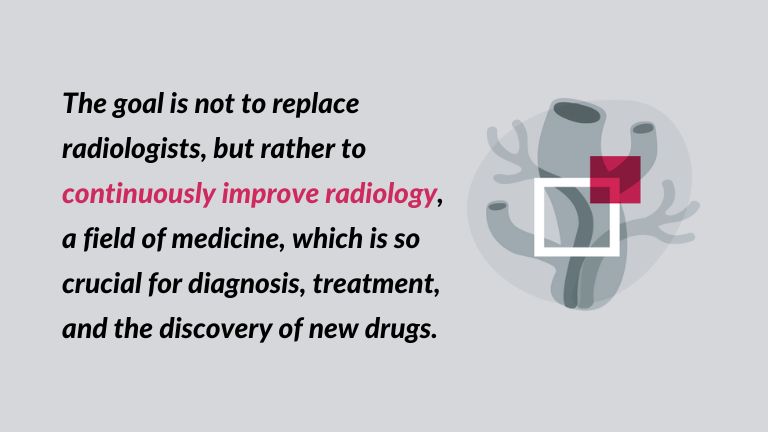
AI as continual improvement in radiology
Many similar studies and publications could be cited here. We are constantly monitoring scientific publications related to this topic. We will definitely describe some of them in the future as well.
However, it seems obvious that the introduction of AI-powered software to the process of analysis and measurement of medical imaging data increases the sensitivity of the measurement. Moreover, in the case of comparing patient response and tests over time, it allows for higher objectivity and repeatability of the measurement.
The goal is not to replace radiologists, but rather to continuously improve radiology, a field of medicine, which is so crucial for diagnosis, treatment, and the discovery of new drugs.
References:
[1] Ristow I. et al., Tumor Response Evaluation Using iRECIST: Feasibility and Reliability of Manual Versus Software-Assisted Assessments. Cancers (Basel). 2024 Feb 29;16(5):993. doi: 10.3390/cancers16050993. PMID: 38473353; PMCID: PMC10931003.
[2] Li H. et al., Exploring the Interobserver Agreement in Computer-Aided Radiologic Tumor Measurement and Evaluation of Tumor Response. Front Oncol. 2022 Jan 31;11:691638. doi: 10.3389/fonc.2021.691638. PMID: 35174064; PMCID: PMC8841678.
[3] Kizildag Yirgin I. et al., Diagnostic Performance of AI for Cancers Registered in A Mammography Screening Program: A Retrospective Analysis. Technol Cancer Res Treat. 2022 Jan-Dec;21:15330338221075172. doi: 10.1177/15330338221075172. PMID: 35060413; PMCID: PMC8796113.
[4] Anindo Saha et al., Artificial intelligence and radiologists in prostate cancer detection on MRI (PI-CAI): an international, paired, non-inferiority, confirmatory study, The Lancet Oncology, 2024, ISSN 1470-2045, https://doi.org/10.1016/S1470-2045(24)00220-1.
[5] Authors defined the test statistic as the difference in the area under the receiver operating characteristic curve (AUROC) metric. It was one of the primary endpoints of this clinical trial, registered as Artificial Intelligence and Radiologists at Prostate Cancer Detection in MRI: The PI-CAI Challenge https://clinicaltrials.gov/study/NCT05489341