Lung cancer prediction using machine learning
Early detection of lung cancer and diagnosis by a physician is a key aspect for improving the prognosis of patients. Modern medicine often uses artificial intelligence to make a diagnosis faster and more efficiently. Researchers are using machine learning (ML) to develop predictive algorithms that can help identify people at high risk of lung cancer and predict the course of the disease in patients who have already been diagnosed.
Predictive algorithms in lung cancer
A world in which diseases are detected before symptoms appear is a vision that is becoming more and more real thanks to predictive algorithms. These powerful tools can predict future events, including cancer risk. The healthcare industry is undergoing a revolution with artificial intelligence (AI) and machine learning (ML). One of the most promising applications of this technology is cancer prediction.
Predictive algorithms analyze vast amounts of patient data, including medical history, genetic information, and imaging scans, to assess an individual’s risk of developing the disease. The potential benefits of cancer predictive algorithms are enormous. Early detection is crucial for successful treatment, and these algorithms can identify individuals who may benefit from more frequent screening or prevention. This can lead to earlier diagnoses, better treatment outcomes, and saving lives.
In this article, we’ll take a closer look at lung cancer predictive algorithms and their potential impact on healthcare.
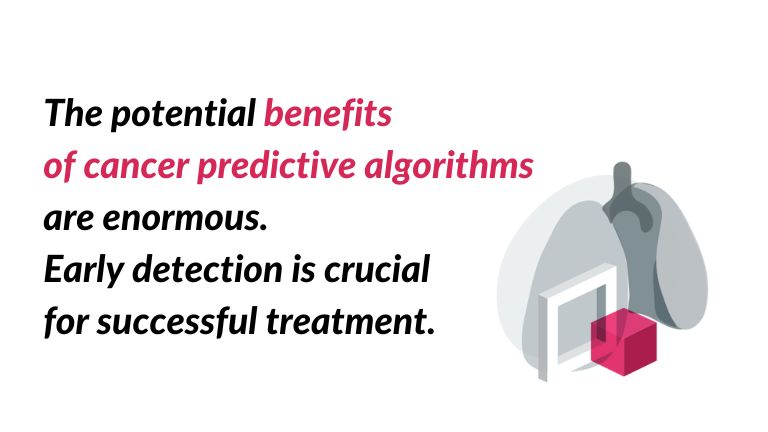
Custom algorithms in lung cancer prediction
Cancer has a high incidence and fatality rate, making it a major global public health concern. Roughly 19.3 million new cases and 10 million fatalities have been reported annually, according to the GLOBOCAN 2020 database. With an estimated 1.8 million deaths, lung cancer continues to be the most common cause of cancer-related mortality.
Despite progress in cancer diagnosis and modern therapies, survival rates are less than five years, which is very low. The main reason for this is primarily late-stage diagnosis and the lack of surgical intervention. However, stable progress in diagnostic and therapeutic methods, particularly those connected with utilizing molecular biology techniques, brings hope for improved prognoses for lung cancer patients.
The use of predictive algorithms can help with:
- Increasing the detection rate of lung cancer at an early stage
- Reducing mortality from lung cancer
- Optimize screening and reduce unnecessary screening
- Allowing for earlier treatment initiation, which may increase the chances of a cure.
Predictive algorithms use a variety of data, such as medical history, risk factors, and biomarkers, to calculate an individual’s risk of developing lung cancer. Based on this data, doctors can develop a personalized screening plan. This plan may include more frequent chest X-rays, computed tomography (CT) scans, or other imaging tests.
Artificial intelligence in lung cancer staging
Medical professionals rely on the TNM (tumour, node, metastasis) classification system to detect lung cancer. This system is primarily based on the results of clinical, radiological and biopsy tests. In many cases, additional medical imaging tests are also needed i.e. computed tomography and PET.
Artificial intelligence has proven repeatedly that it is perfect for analysing large amounts of image data. For years, it has been an invaluable support for radiologists and others performing tests for the diagnosis of cancer. AI algorithms speed up the lung cancer detection process, relieving radiologists of the often time-consuming analysis of medical imaging images. The accuracy of staging depends largely on the radiologist’s experience, and interpreting CT scans of lung nodules can be difficult. This leads to incorrect or overlooked diagnoses, making it difficult to detect the disease early.
Importantly, AI can help eliminate misinterpretations. Studies using neural networks to analyze images have shown improvements in precision in determining the stage of cancer. Not only can AI in image processing lead to earlier and more accurate diagnoses, but it can also increase the efficiency of doctors’ work.
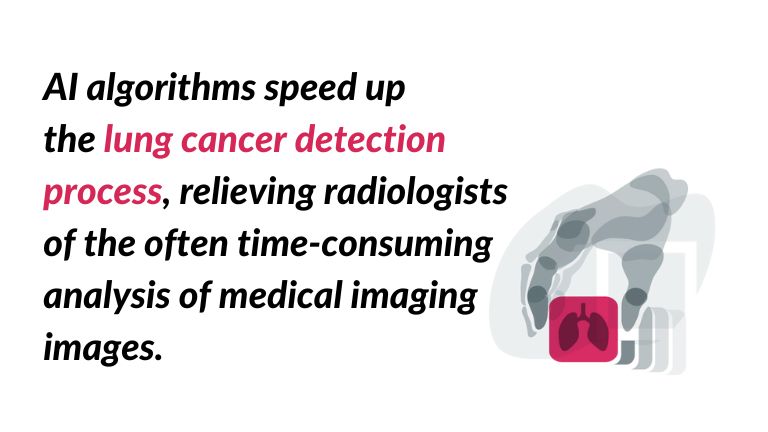
Factors influencing AI accuracy in lung cancer prediction
The accuracy of artificial intelligence (AI) algorithms used to predict lung cancer depends on many factors. Here are some of the highlights:
- Quality and quantity of training data: AI algorithms learn from data. Training data should include not only medical images (e.g., chest X-rays, CT scans) but also clinical data of patients.
- Neural Network Algorithm and Architecture: Choosing the right neural network algorithm and architecture has a significant impact on the accuracy of prediction.
- Training data bias: Bias, or hidden trends in training data, can negatively affect the accuracy of AI algorithms.
- Interpretability of the algorithm: Understanding how the AI algorithm makes decisions is crucial to ensuring its reliability.
- Medical expert collaboration is crucial: This ensures the software aligns with current medical knowledge and that doctors can properly interpret its results.
Improving the accuracy of AI algorithms in lung cancer prediction requires continuous improvement of machine learning methods, the collection of high-quality training data, and collaboration between algorithm developers and physicians.
Regulations for AI in Lung Cancer detection
It’s important to note that NICE’s diagnostic guidance, published on 5 July 2023, highlights that AI-derived computer-aided detection (CAD) software can be used to help clinicians detect and measure lung nodules on CT scans as part of targeted lung cancer screening. That can automatically identify and measure lung nodules on CT images, enabling healthcare professionals to make decisions about further investigation or surveillance.
In fact, the use of artificial intelligence (AI) systems in every industry is becoming increasingly common. However, its use in healthcare presents numerous legal challenges. In each country where it’s used, medical software, including predictive algorithms for detecting lung cancer, needs to comply with a number of restrictive regulations. These requirements include, ensuring the safety and efficacy of medical devices and their compliance with relevant standards.
Medical Device Regulation (MDR): This regulation replaced the MDD in 2021. The MDR introduces several new requirements for medical devices. These include conducting clinical trials and evaluations and ensuring ongoing safety supervision aftermarket placement. Despite these challenges, innovative AI-based solutions are constantly being developed to diagnose lung cancer faster and more accurately. Continuous improvement of predictive algorithms and their wider application in medical practice can significantly contribute to the decrease in mortality due to this disease