Do we have a gold standard for clinical predictive analytics?
The answer should sound like it is not yet. The more variables there are, the harder it is to predict what will happen. This can be easily compared to creating weather forecasts. We have specific variables that create certain patterns when a specific situation occurs. In the field of health, this is not so obvious. We can assume, for example, that people who smoke cigarettes and are over 50 are more likely to get throat cancer. But if we were to look at other diseases, the answers wouldn’t be so obvious.
Simplifying and looking for shortcuts doesn’t work in medicine. However, there is a study that indicate at what stage of clinical predictive analytics, and thus also personalized medicine, we are.
Clinical predictive analytics in Aizheimer’s screening
The Alzheimer’s Association offered tests to people who did not have any symptoms indicating this disease. The study was to be based on the determination of specific biomarkers in the blood. They indicate the accumulation of β-amyloid in the brain and the classification of “stage 1 Alzheimer’s disease”.
Is it reliable? Unfortunately, probably not, because it is only one of many layers of data. Risk can be more accurately assessed, for example, using orthogonal genomic data. In summary, these types of screening tests generate false positive results. In addition, they are too simplistic and can lead to social unrest.
Data as key to early detection of Alzheimer’s: the role of predictive algorithms
Let’s talk about data because it is the most important thing here. If you want to try to detect the disease early, you need to take into account many factors. And not just two blood markers, as I described in the previous paragraph.
Some time ago, there was a machine learning-based study that was able to predict Alzheimer’s disease. Interestingly, it took as many as 7 years before the patient was diagnosed. And all this is thanks to the integration of data from electronic medical records.
These data included information such as;
- blood cholesterol levels,
- blood pressure,
- the amount of vitamin D,
- gender-specific features; Osteoporosis in women and erectile dysfunction and prostate enlargement in men.
Of course, there are several other dimensions of data that could have been integrated to increase the effectiveness of the algorithm. However, such a subset made it possible to detect the disease long before its first symptoms.
Breast and prostate cancer risk: a step towards personalized prevention?
Preventing cancer before the first symptoms appear? It sounds like an ideal and unreal world at the same time. However, researchers are slowly finding pieces of the golden recipe to bring humanity closer to personalized cancer prevention.
Recently, the National Human Genome Research Institute–funded Electronic Medical Records and Genomics (eMERGE) Network published a validation of polygenic risk with readiness for clinical implementation. Two types of cancer – breast and prostate – were assessed based on diverse and large cohorts of data. Interestingly, The Mass General Brigham health system has taken care of implementing this solution for its patients. And so, in the diagram below, we can see the results of these studies:
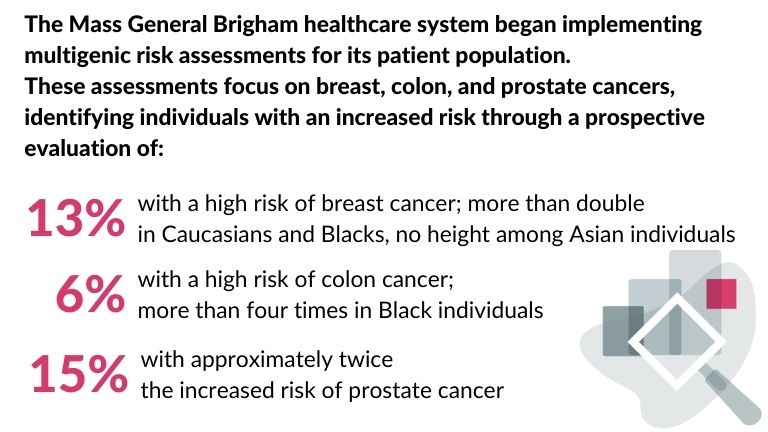
Let’s appreciate the role of electronic medical records
I have already mentioned that patient data from the EHR system is extremely important from the point of view of training predictive medical algorithms. Unfortunately, they are still not used very often.
I will illustrate this with the example of pancreatic cancer which is one of the most difficult to diagnose. Usually, it is detected at an advanced stage, when there is practically no chance for effective therapy. However, there’s promising research. Scientists analysed data from a national registry in Denmark and electronic health records from US veterans. This analysis allowed them to identify people with a significantly increased risk (30 to 60 times higher) of developing pancreatic cancer within the next year.
The AI model integrated more than 80 functions from EHR. The patterns he detected are usually not perceptible to doctors. Although a CT scan of the chest is performed for other reasons, it can reveal abnormalities in the pancreas. However, this is often overlooked by radiologists, who focus on other important aspects when describing such an examination.
An interesting fact is that studies indicate greater sensitivity in the detection of abnormalities by AI, that AI can detect such abnormalities with much greater sensitivity (in one study, 34% sensitivity by radiologists, 93% by artificial intelligence).
Are we one step away from personalized medicine?
Detecting diseases before any symptoms appear is the key to effective prevention and treatment. Being able to identify people at high risk of developing the disease years before symptoms appear can give them time to make lifestyle changes and take advantage of new treatments. Combining genetic, environmental and lifestyle data can enable us to more accurately predict an individual’s risk of dementia.
As the research indicates, there is a huge amount of data that can be used in clinical predictive analytics. Unfortunately, this data is often scattered. Leveraging computationally intensive resources and conducting prospective validation studies on a large scale can help us realize their full potential.
References:
Medical forecasting: https://www.science.org/doi/10.1126/science.adp7977