Assessment of tumor volume in oncology drug development process
It’s no secret that probability of success (POS) for drug development is quite low and, as you could read in one of our previous blog posts, the number of new substances approved by the FDA has been on a consistent decline year after year. Additionally, when looking at different therapeutic areas, oncology maintains the lowest POS rate1.
Medical imaging, also thanks to the technological advances that have been taking place in radiology, is becoming one of the key methods of evaluation. Thanks to AI algorithms, we can monitor the effectiveness of therapy or personalized therapeutic strategies. What’s more, in the early stages of clinical trials – make earlier go/no-go decisions. The popularity of using methods of advanced medical imaging analysis is enhanced by the fact that the FDA is increasingly accepting biomarkers extracted in this way as surrogate endpoints in traditional and fast-track drug approval.
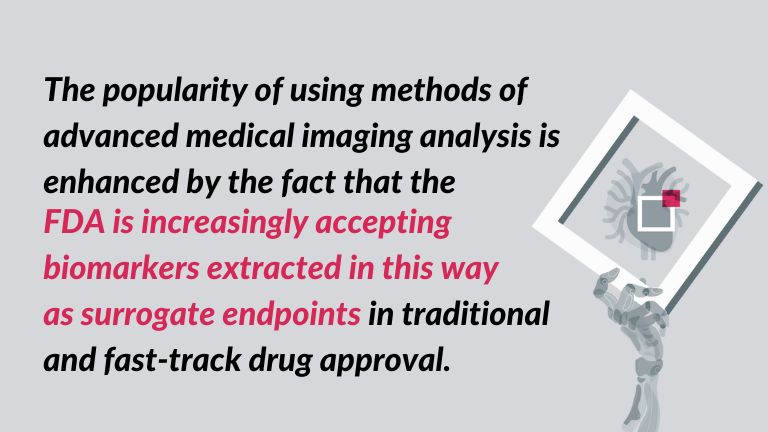
Tumor evaluation using linear measurement methods
Tumor mass evaluations form the basis of response assessments in many clinical trials of anticancer drugs. Traditionally, these evaluations are based on linear measures of tumor size.
Even the choice of assessment criterion influences the clinical trial, as exemplified in a paper by Jack M. Qian et al2. The authors describe a situation in which RECIST (mRECIST), was used during the development of a clinical trial protocol. The minimum size of the target brain lesion was 5 mm in the longest diameter. Researchers evaluated up to 5 brain lesions. They decided to additionally support RANO high-grade glioma (RANO-HGG), and RANO brain metastases (RANO-BM). Although the concordance of results using these different criteria was surprisingly high, “However, compared to RECIST 1.1 or RANO-BM, using a 5 mm threshold for target brain lesions in mRECIST allowed enrollment of 13 additional patients, five of whom had durable responses. Compared to RANO-HGG, 19 additional patients were enrolled using mRECIST, eight of whom had durable responses. Consequently, this resulted in response rates ranging from 12% with RANO-HGG to 28% with mRECIST”3.
Drug development and the limitations of linear measurement
The criterion alone is not the only issue implying limitations. Manual and linear measurements in 2D are affected by variability in technical factors, tumor morphology, or finally, decisions by medical annotators. Automatic or semi-automatic assessment of the total tumor volume could overcome these limitations. Additionally, it might improve our ability to assess even a small patient’s response to an active agent. Gregory Goldmacher and James Conklin, for example, have written about this, proposing that every effort should be made to “qualify tumour volume as a biomarker for the purposes of drug development”4. Since then, technology has advanced so much that this option is now within reach.
Gaining the advantage
The limitations of 2D measurement are reflected in the low POS in drug development, or rather: they are one factor. Of course, many factors influence the success of a clinical trial. On one hand, it may be a fact that human being has a right to react differently than animal model to a given substance. That’s why the translation of conclusions from animal tests sometimes biased. This is just an example – there are many other biological factors.
The list goes on: inappropriate choice of active ingredient dose, non-optimized assessment schedules… The challenges are many and not all are easily answered. However, we can gain an advantage over resisting nature when it comes to data and its analysis. This is where we can make a difference. This is where we can objectivize, automate, and improve the most.
AI-enabled medical images analysis and volume calculation
By applying AI algorithms to medical imaging analysis, we can overcome limitations caused by varying technical factors. These algorithms can function independently of specific equipment manufacturers and significantly improve image quality. And that translates to reduced errors that might occur due to human interpretation. Is it a revolution? Rather an important improvement. RANO has been widely accepted in neuro-oncology trials – and that is great, although we all could use some additional refinements.
The definition of progression adopted in RANO, “≥ 25% increase in the product of perpendicular diameters compared with baseline or best response”5 does not fully capture the finer changes – when calculating total tumor and subregions volume, we are able to more accurately observe the changes occurring within the tumor (ability to detect even small changes). As noted by David Mozley, this is critical, especially when the tumors themselves, which we observe and evaluate are small6.
Can really volumetric assessments reflect drug response better?
A number of studies tries to verify what real clinical significance the calculation of tumor lesion volume can have. Let me here cite a study by Hayes et al7. It was aimed at examining the correlations between uni-dimensional RECIST and volumetric measurements in patients with adenocarcinoma. The goal was to assess their association with overall survival (OS) and progression-free survival (PFS). A retrospective analysis of 42 lung cancer patients participating in an open-label phase 2 study showed that volumetric measurements at first follow-up (4 weeks after treatment initiation) were better able to predict overall survival than RECIST measurements.
Volume measurement isn’t yet a gold standard for drug trials, but many studies are validating its potential, with promising results. Nevertheless, even if today we cannot rely only on this measurement during evaluation, its inclusion provides repeatable, objective data. This increases measurement sensitivity, leading to better “go/no go” calls and higher ROI.
References
[1] https://www.nuventra.com/resources/blog/why-do-clinical-trials-fail/
[2] J. M. Qian et al: Comparing available criteria for measuring brain metastasis response to immunotherapy, Journal of Neuro-Oncology volume 132, pages479–485 (2017), https://link.springer.com/article/10.1007/s11060-017-2398-8
[3] Ibidem
[4] G.V Goldmacher, J. Conklin: The use of tumour volumetrics to assess response to therapy in anticancer clinical trials, British Journal of Clinical Pharmacology 2012 Jun; 73(6): 846–854, https://www.ncbi.nlm.nih.gov/pmc/articles/PMC3391506/
[5] P.Y. Wen et al: Response Assessment in Neuro-Oncology Clinical Trials, Journal of Clinical Oncology 2017 Jul 20; 35(21): 2439–2449, https://www.ncbi.nlm.nih.gov/pmc/articles/PMC5516482/
[6] P. David Mozley et al: Change in lung tumor volume as a biomarker of treatment response: A critical review of the evidence, Annals of Oncology 2010 21(9):1751-5, https://www.annalsofoncology.org/article/S0923-7534(19)40065-3/fulltext
[7] SA Hayes et al: Comparison of CT volumetric measurement with RECIST response in patients with lung cancer, European Journal of Radiology 2016 Mar; 85(3): 524–533, https://www.ejradiology.com/article/S0720-048X(15)30191-1/fulltext
See our case study on automated brain tumor segmentation, volume calculation and patient response