AI in Healthcare – news picked by GLI /03
Presenting the latest iteration of “AI in Healthcare: News picked by GLI”, a compilation of news and articles delving into the latest advancements and nascent directions at the crossroads of artificial intelligence and the healthcare sector, especially medical image analysis area. This series aims to provide you with a compilation of news updates and perspectives, thoughtfully picked by the Graylight Imaging (GLI) team.
AI in healthcare meets cardiovascular imaging
The Society of Cardiovascular Computed Tomography (SCCT) is an international community of physicians, scientists, and technologists who advocate for cardiovascular computed tomography (CCT) research, education, and clinical excellence. In July SCCT published a methodology for developing scientific documents. It is a must-read practice guideline if you’re doing research or experiments based on cardiovascular imaging. [1]
All this effort and methodology revolves around generating real evidence for the technology’s performance and significance. This very topic sparked an intriguing discussion as well during the Annual SCCT Meeting. [2] The topic of population screening appears to be relatively non-controversial, with many potential opportunities for faster and improved diagnostics and patient outcomes, which might be crucial when it comes to the world’s number one killer: cardiovascular disease. But: is there enough evidence to support broad, population-based screening for atherosclerosis using coronary CT angiography (CCTA) and other methods, rather than the risk factor-based approach advocated by guidelines? For more information on this passionate debate, see the linked article.
Speaking of cardiovascular image analysis involving AI – our GLI experts will participate in the 2023 IEEE International Conference on Image Processing in Kuala Lumpur from October 8 to 11, 2023, with a presentation dedicated to one of our cardiac projects. Stay tuned, more info coming soon.
Does AI in healthcare truly bring big numbers to the table?
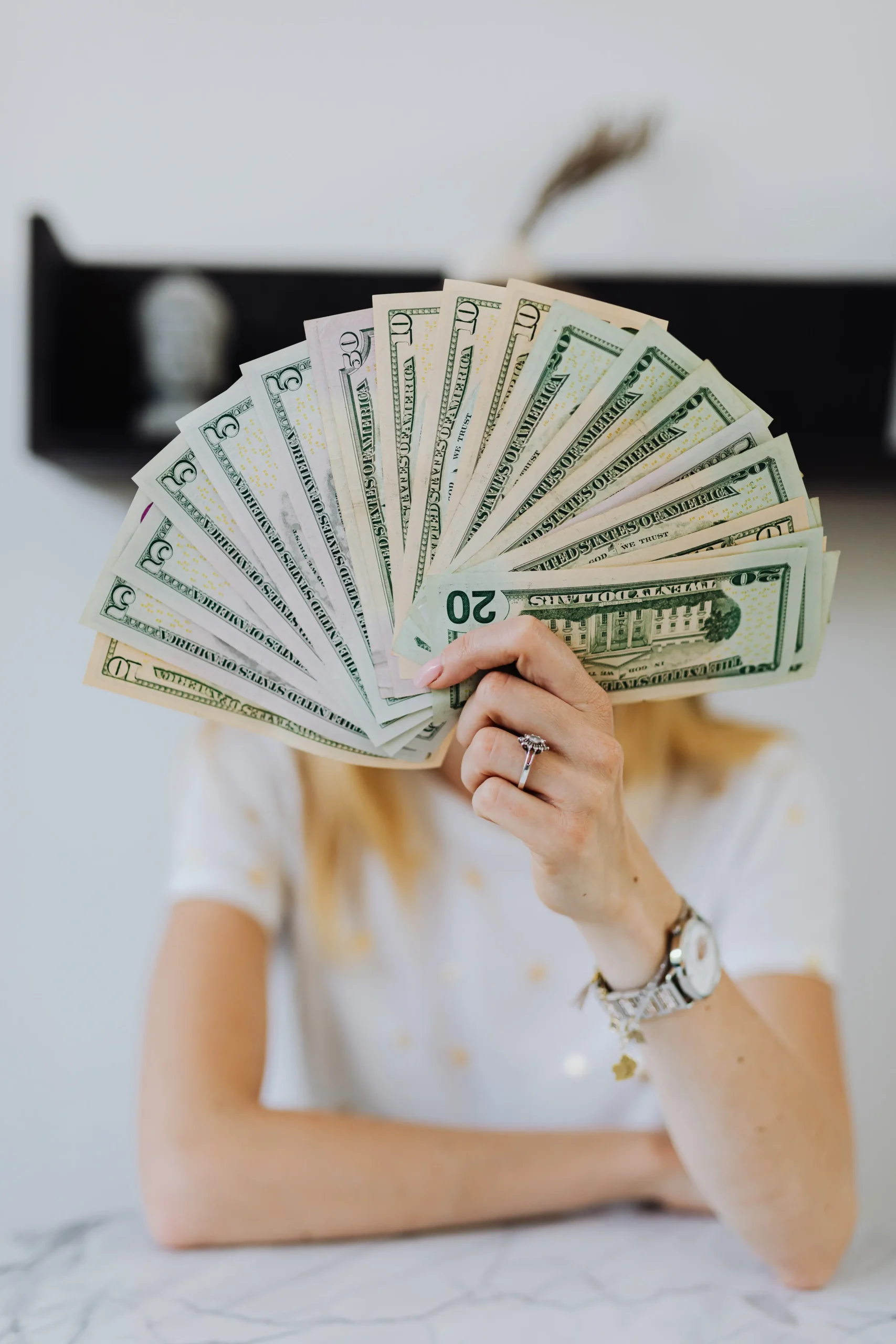
The transition from AI in healthcare research to clinical practice is, of course, closely tied to funding… as much as to cost savings. David M. Cutler from Harvard University estimated that savings from the implementation of AI in the everyday tasks performed by healthcare professionals could range from $200 billion to $360 billion annually, about 35% of which would be administrative savings. [3]
It’s clearly visible that, in addition to mathematical modeling, such data and estimations also find application in the business strategies of market giants. Amazon launched a new generative tool that will allow software developers and healthcare providers to use speech recognition, machine learning and AI to summarize doctors’ visits and manage files [4]. This is a visible direction pursued by the world’s largest healthcare companies, not only Amazon. The question of the actual implementation of this solution remains open, especially as we wrote in the latest edition of this series – over 70% of Americans are concerned about the utilization of AI in healthcare [5]. Nonetheless, this trend is worth noting, and it will almost certainly appear on our blog and “AI in healthcare – news picked by GLI” series more than once.
Segment Anything Model’s impact on medical image analysis
And the last but not least – a must-read recommended by our ML Team. As we all know, because of the scarcity of available data annotations, training segmentation models for medical images remains a challenge. The Segment Anything Model (SAM), which was originally trained on a massive dataset with over a billion annotations for natural images, is a foundational model designed to segment user-defined objects of interest through interactive means. Despite its outstanding performance on natural images, the domain of medical imaging adds new complexities.
In this context, Maciej A. Mazurowski et al. conducted a thorough evaluation of SAM’s efficacy in segmenting medical images across a collection of 19 diverse medical imaging datasets spanning various modalities and anatomical regions. Authors used standard techniques to generate point and box prompts for SAM in our experiment, simulating interactive segmentation. Their observations lead to the very interesting conclusions presented in an article Segment anything model for medical image analysis: An experimental study, published online 2 August 2023. [6]
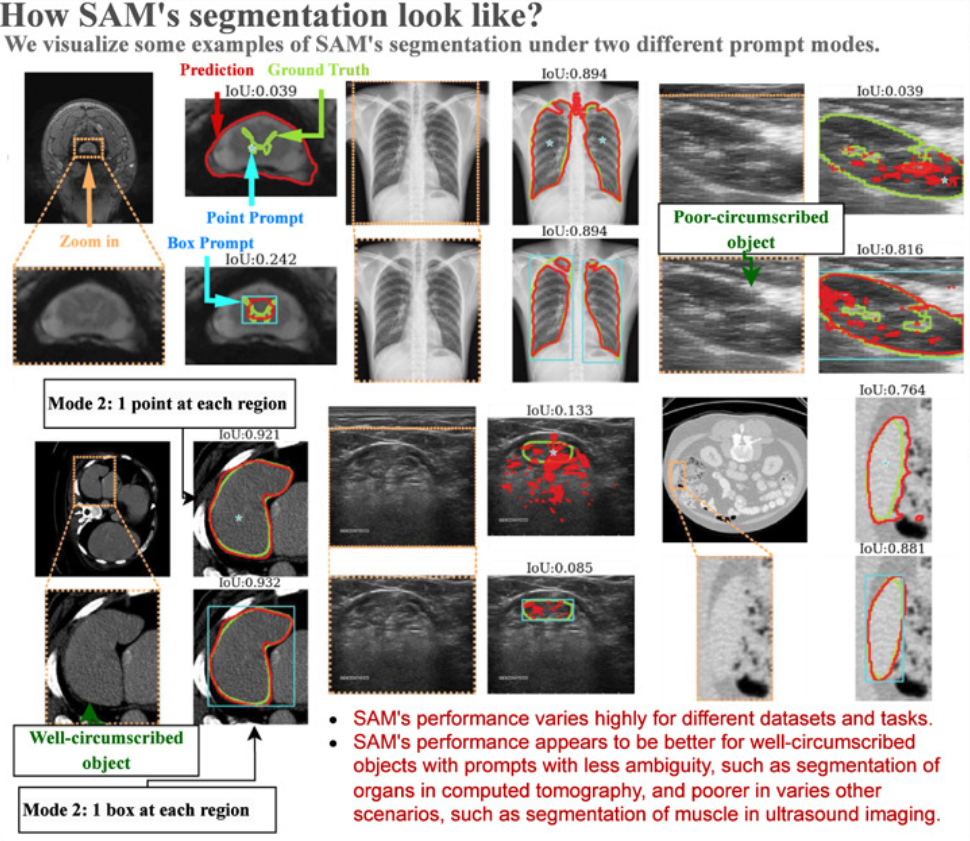
Image source: Maciej A. Mazurowski, Haoyu Dong, Hanxue Gu, Jichen Yang, Nicholas Konz, Yixin Zhang, Segment anything model for medical image analysis: An experimental study, Medical Image Analysis, 2023, 102918, ISSN 1361-8415, https://doi.org/10.1016/j.media.2023.102918
References:
[1] Scientific document development standards for the society of cardiovascular computed tomography (SCCT): A statement from the SCCT Guidelines Committee, https://doi.org/10.1016/j.jcct.2023.06.006
[2] https://www.tctmd.com/news/population-screening-coronary-plaque-evidence-enough
[3] Cutler DM. What Artificial Intelligence Means for Health Care. JAMA Health Forum. 2023;4(7):e232652. https://jamanetwork.com/journals/jama-health-forum/fullarticle/2807176
[5] https://graylight-imaging.com/ai-in-healthcare-news-picked-by-gli-02/
[6] Maciej A. Mazurowski, Haoyu Dong, Hanxue Gu, Jichen Yang, Nicholas Konz, Yixin Zhang, Segment anything model for medical image analysis: An experimental study, Medical Image Analysis, 2023, 102918, ISSN 1361-8415, https://doi.org/10.1016/j.media.2023.102918