AI for clinical prediction in a nutshell
In recent years, AI has greatly advanced clinical prediction with its ability to process complex data sets. It’s a type of process that uses historical patient data to predict future health conditions. This makes it possible to detect diseases such as cancer or heart disease early, which significantly improves the chances of successful treatment. Artificial intelligence is used in many fields of medicine, from oncology, through cardiology, to epidemiology.
However, in today’s post, I will not devote my attention to them, but to a general look at how artificial intelligence is currently used in medicine.
The AI eight domains for clinical prediction
The eight areas where AI supports clinical predictions are:
- Diagnosis – diagnostic predictions are essential for early detection and timely treatment.
- Prognosis – help in understanding the disease’s progression, potential complications, and the likely response to treatment.
- Risk Assessment– predicting future health risks based on various factors
- Treatment Response – this is especially important in personalised medicine, where the goal is to tailor treatments to individual patients for maximum effectiveness.
- Disease Progression – it helps in planning long-term treatment strategies and anticipating future care needs.
- Readmission Risks – this helps in providing targeted interventions to reduce readmission rates
- Complication Risks – is vital for informed consent and for planning to mitigate those risks.
- Mortality Prediction – is important for decision-making regarding treatment intensity and end-of-life care planning.
AI provides valuable insights that can significantly improve the quality of healthcare. Thanks to its ability to analyze large amounts of data, it allows you to identify even very subtle patterns. Its potential in medicine is enormous, but there are some challenges faced by algorithm developers. It is mainly about the quality of the data on which the AI is trained, the interpretability of language models and ethical aspects. However, we feel that with the development of this field, we will be able to find an appropriate vademecum for these issues.
Which medical specialties are leading the way in using predictive algorithms?
The author of the publication, based on the analysis of 74 studies, created a graph presenting the use of artificial intelligence in clinical prediction in various fields of medicine. As we can see, oncology, along with radiology and neurology, occupies the first three positions on the list. They are followed by surgery, cardiology, pulmonology, orthopaedics and critical care medicine, with a share of over 5%. Other fields of medicine, although they also use the potential of AI, have a smaller share in predictive applications (less than 5%). However, it is worth noting that the development of technology and the increasing availability of data may lead to changes in this area in the future.
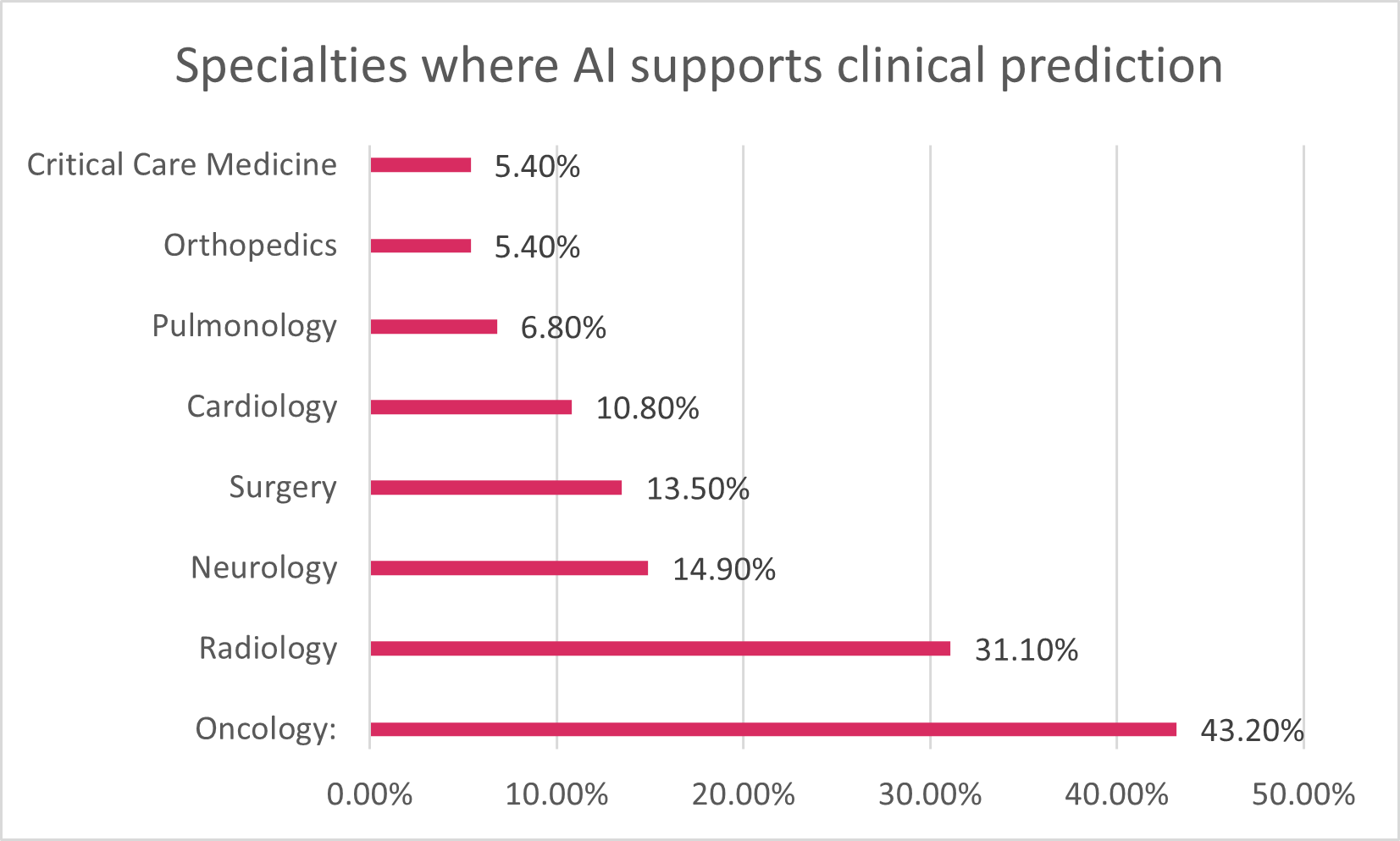
Fig. 1 Specialties where AI supports clinical prediction. Own graph based on https://www.sciencedirect.com/science/article/pii/S2666990024000156
In medical image analysis, AI can detect tiny and complex patterns in X-rays, MRIs, CT and other modalities. This ability is indeed important in identifying the early stages of disease, such as cancer or neurodegenerative diseases. In recent years, artificial intelligence has developed significantly as a tool to support the work of radiologists. Therefore, these results are not a surprise to us, it’s only a confirmation that the predictive algorithm development in the radiology field is significant.
A real examples of predictive algorithms
AI is very useful in assessing the risk of disease, which allows for early implementation of preventive actions. In addition, as a tool to support physicians in their daily practice, it helps to predict: the risk of complications after procedures, the risk of rehospitalization, and the risk of mortality in critically ill patients. This allows doctors to make better treatment decisions, thereby providing patients with better healthcare.
If you are interested in specific examples of predictive algorithms. Then I would like to recommend you our two blog posts. The first one concerns a neurodegenerative disease, namely Alzheimer’s. A team of researchers wanted to create prediction algorithms in alzheimer’s to predict the transition from mild cognitive impairment to Alzheimer’s disease. The second example concerns an oncology field and it’s described example of predict pancreatic cancer.
Of course, the fact is that how the algorithm is supposed to help both doctors and patients depends on many factors such as the medical field or the complexity of the medical case. The development of artificial intelligence over the past few years has made it play an increasingly important role in medicine, enabling more accurate diagnosis and prediction of diseases. This helps doctors create more personalized treatment plans and minimize side effects.
The future of AI in clinical prediction
Artificial intelligence, especially machine learning and deep learning methods, are revolutionizing clinical prognosis. AI algorithms are excellent at analyzing large amounts of complex medical data, such as images or texts, enabling the detection of diseases at an earlier stage. This allows clinicians to diagnose patients faster and more accurately and create more personalized treatment plans.
However, the development of this technology also comes with some challenges. One of the most important is the need to ensure high quality data, which is the basis for creating effective AI models. The interpretability of models is a significant challenge, as it can sometimes be difficult to understand exactly why an algorithm made a particular decision. The key to success, in our opinion, is a balanced approach, where there is synergy between human intelligence and artificial intelligence, which will lead to the development of effective and personalized therapies.
Conclusion: Informatics and ethics must cooperate
The subject of predictive algorithms in medicine is not only multithreaded, but also dynamically evolving. Continuous technological progress in the field of development of machine learning and deep learning methods allows us to believe that in the coming years we can expect a significant development in this field.
We also face some challenges. It is necessary to provide high-quality data for training algorithms, as well as to develop methods that will enable understanding their operation and building confidence in their results. In addition, it is important to remember about the ethical aspects related to the use of algorithms in medicine, such as the privacy of patient data or liability for potential errors.
Despite these challenges, the prospects for the development of predictive algorithms in medicine are extremely promising. Thanks to them, we can expect improved diagnostics, personalization of therapy, increased treatment efficiency and reduction of health care costs. To fully exploit the potential of computer science, ethics and medicine.
References:
Mohamed Khalifa, Mona Albadawy. Artificial Intelligence for Clinical Prediction: Exploring Key Domains and Essential Functions. Available online 7 March 2024, Version of Record 21 March 2024.
https://www.sciencedirect.com/science/article/pii/S2666990024000156