Predictive models to support the future diagnosis of Alzheimer’s disease
If prediction algorithms for Alzheimer’s disease are well-developed, they could help identify individuals at risk and guide early intervention strategies. AD leads to a gradual loss of memory, and the ability to understand, think, and control behaviour. It mostly affects older people ages 65 and above, but it is not a rule. Young people also suffer from it. There are several therapies used to slow the progression of this disease, however, there is no single effective cure for it.
The causes of this disease are believed to be the abnormal accumulation of proteins in and around brain cells. Researchers have found that tissue outside the brain that contains the misfolded amyloid beta-amyloid protein can move around and “infect” the brain. Pathological protein deposits have been closely linked to the genesis of a neurodegenerative disorder called Alzheimer’s disease, AD in shortcut.
I want to draw your attention to a scientific publication on prediction algorithms in Alzheimer disease. A team of researchers embarked on a project to develop predictive models to forecast this disease’s progression from different stages. More specifically, they wanted to predict the transition from mild cognitive impairment to Alzheimer’s disease using radiomic models based on positron emission tomography (PET) images.
ADNI databases to develop a predictive model for MCI progression
This study utilized data from the Alzheimer’s Disease Neuroimaging Initiative (ADNI) project, specifically ADNI2 cohorts, which include 3 years of follow-up records. The study used information on 278 patients with mild cognitive impairment. Researchers divided patients’ data into two sets. The first was the training which contained data on 222 people. The second was the validation group, which had a collection of 56 people.
Researchers employed voxel-based analysis of 18F-FDG PET images to identify brain regions that exhibit significant differences between the pMCI and sMCI groups. Here, pMCI refers to mild cognitive impairment progressing to Alzheimer’s disease. While sMCI indicates mild cognitive impairment that does not progress to Alzheimer’s disease.
Radiological features were extracted from these regions, key features were selected and predictive models were developed for individual and connected brain regions. The effectiveness of the prediction algorithms in Alzheimer’s disease was assessed using indicators such as AUC (the area under the curve). Moreover these indicators were used to determine the most accurate predictive model for the progression of mild cognitive impairment.
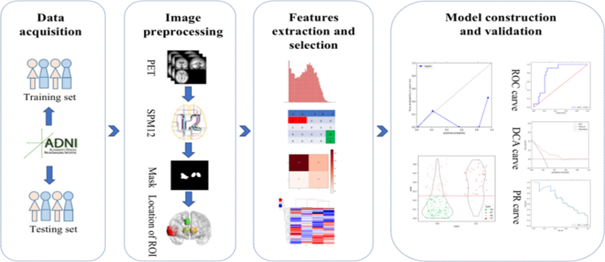
Fig. 1. Research flow chart. Source [1].
Localization of brain regions with glucose metabolic differences related to MCI to AD progression
In this paragraph medical imaging analysis plays a key role in this stage of research. Figure 2 illustrates the results of voxel-based two-sample t-tests performed in a training group that consisted of 218 subjects with MCI not progressing to AD and 60 subjects with MCI progressing to AD.
Indeed four regions showed significantly reduced glucose metabolism, indicating their involvement in disease progression. In particular, ROI1 is located in the temporal lobe, suggesting its key role. Both ROI2 and ROI3 are located within the thalamus, while ROI4 is located in the limbic region, highlighting these areas as key sites of metabolism reduction associated with the progression of MCI toward AD.
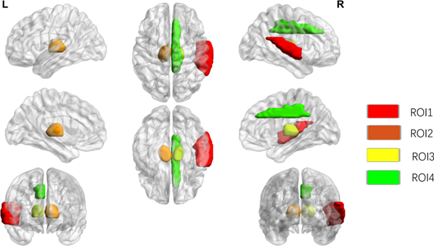
Fig. 2. Results of t-test for two samples undergoing brain 18F-FDG positron emission CT to assess metabolic differences in brain regions between sMCI patients and pMCI. Source [1].
Predicting MCI progression by multiregional radiomic analysis
Evidently, this study showcases an advanced radiomic workflow that utilizes 18F-FDG PET imaging to predict the progression from mild cognitive impairment to Alzheimer’s disease. They’ve focused on analysing 18F-FDG images to pinpoint the areas of the brain where glucose metabolism differs significantly during the MCI transition. This analysis facilitated the extraction of a wide range of radiomic features from these critical areas, leading to the identification of key imaging biomarkers.
Feature fusion integrated features from all identified regions, which significantly improved the predictive accuracy of the comprehensive multi-region radiomic model compared to the single-region approach.
These findings highlight the potential of radiomic analysis, particularly targeting regions of altered glucose metabolism, to predict the development of mild cognitive impairment in the long term. This approach offers promising opportunities for non-invasive monitoring and clinical assessment of mild cognitive impairment, paving the way for early and targeted intervention.
High accuracy of the predictive model in predicting the progression of MCI to AD
Voxel-based analysis revealed four brain regions associated with progression from mild cognitive impairment to AD.It is worth noting that the comprehensive model, which included all identified brain regions, outperformed the results of the below data. Achieved an AUC of 0.884 in the training set and an AUC of 0.816 in the validation set.
The predictive models developed for specific regions in the brain were as follows:
- ROI1 in the temporal lobe,
- ROI2 in the thalamus,
- ROI3 in the thalamus – lowest efficiency AUC 0.75,
- ROI4 in the limbic system – highest predictive accuracy:
- Training Set AUC 0.803
- Validation kit AUC 0.733
Brain PET radiomics: Promising results and further challenges in the diagnosis of Alzheimer’s disease
In brief the study indicated that the limbic system is the region of the brain most associated with the progression from mild cognitive impairment to Aizhaimer’s disease. A multi-brain PET model of brain radiomics is far superior to models based on single brain regions. This comprehensive approach more accurately identifies patients with MCI at high risk of progression to AD. It offers valuable insights for noninvasive diagnosis and facilitating early and timely interventions in the clinical setting.
Unfortunately, there are some limitations to this approach. One of them is PET image processing. When you use too much smoothing, the results may not be reliable. By obscuring some texture details that could potentially affect the reliability of radiomic extraction.
Predictive models for the detection of Alzheimer’s disease, including various prediction algorithms, still require multiple approaches and methodologies. Additionally, thorough validation of radiomic analyses is essential. These efforts aim to enhance the accuracy and reliability of these models.
References:
[1] Mu, X., Cui, C., Liao, J. et al. EJNMMI Rep. 8, 19 (2024). https://doi.org/10.1186/s41824-024-00206-8
[2] https://www.alz.org/alzheimers-dementia/what-is-alzheimers