RESEARCH AND DEVELOPMENT
Our R&D team was established in 2016 to contribute to the advancement of automated medical imaging analysis.
Our scientists develop and publish scientific papers. Furthermore they develop specific solution components in areas with high medical benefit, which are extensible to other tissues and organs.
Graylight Imaging’s R&D teams develop also tools and refine methods to accelerate the creation of new analytical solutions and they support achievement medical certification for our organisation and components (where appropriate) to aid efficient certification of client solutions.
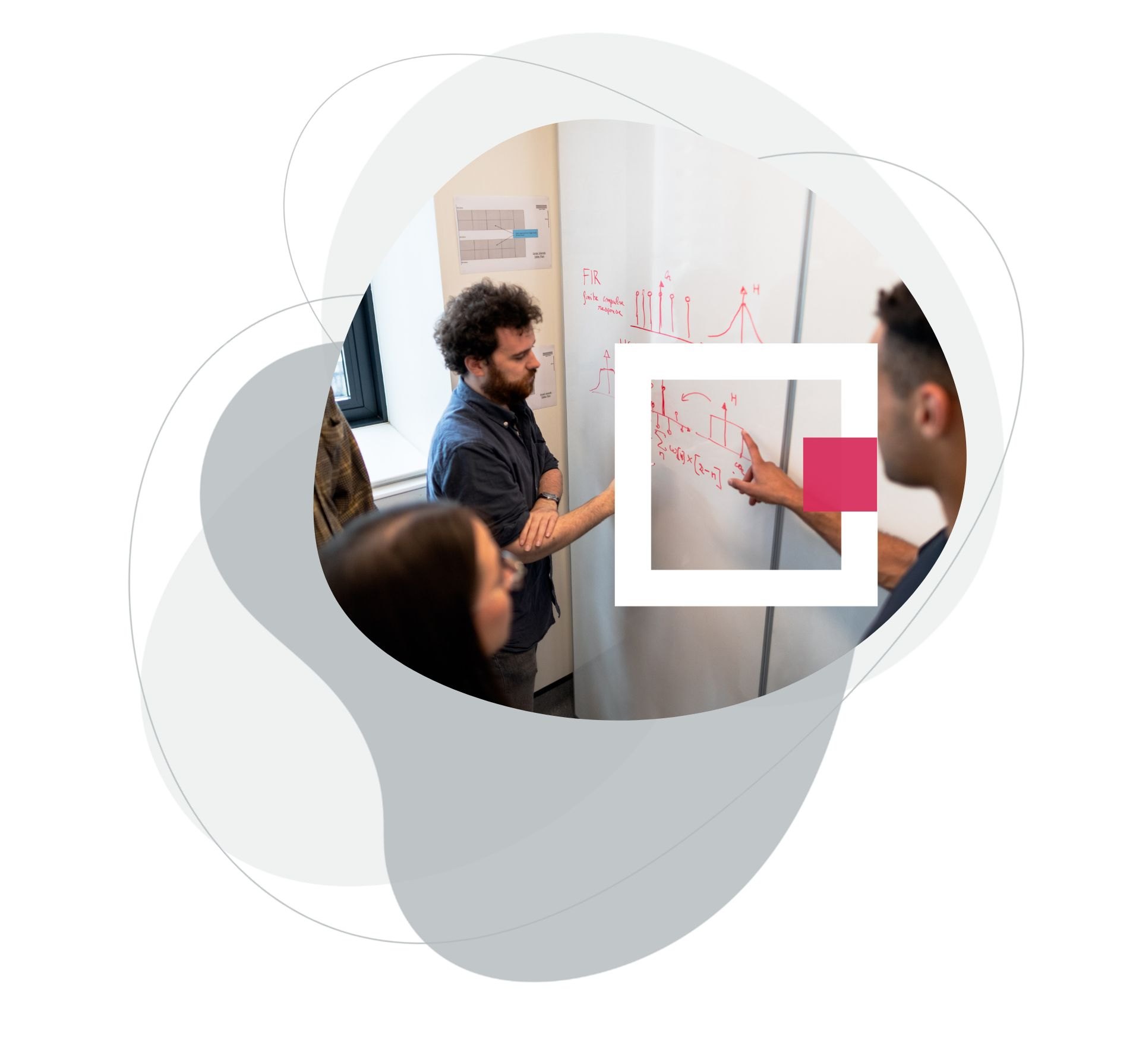
Brain lesions
(segmentation and analysis)
SensAi (version 2.0)
To provide a fully automated analysis of brain lesions for glioma patients using MRI scans, employing AI deep learning techniques. To include: automatically identifying tumors, oedemas and necroses from an image, visualization of the results and calculation of the volume of each of the sub regions.
To provide a standardised view of volume change over time: to support treatment planning. to measure effectiveness of interventions.
To provide an initial impact assessment: to assist with initial intervention triaging, 2nd pair of eyes for a radiologist.
We can easily extend the existing solution for clients needing additional metrics (e.g. RANO criteria). Based on this, and similar segmentations, we can reliably develop segmentations of other tissues and organs.
The approach for version 1 is described in this scientific paper: Version 2 expanded the scope and used a similar approach.
Version 1: the solution achieved a DICE score of 0.882 and has been certified as a class IIa medical device: results of a scan were delivered in less than one minute, creates DICOM files, which can be uploaded to a PACS database, provides consistent results irrespective of the hardware used for scanning.
Version 2: achieved 6th place (in worldwide competition of 2,600 entrants, including the world’sleading medical centres) in the MultimodalBrain Tumor Segmentation (BraTS) Challenge 2021 (http://braintumorsegmentation.org).
Coronary arteries
segmentation and visualisation
Cardio4D / Segmentation
To provide a fully automated analysis of coronary arteries, using AI deep learning. To include: identifying coronary arteries from CT images in 2D, build 3D model from 2D slices, blending voxels for smoothing and resolution enhancement, 3D high contrast visualisation with 360o panning.
Our goal is to provide clinicians with advanced tools enabling for better clinical decision making and risk prediction.
Based on precise 3D CT reconstruction of coronary arteries our solution allows for improved non-invasive assessment of coronary artery disease.
The existing solution (including voxel blending) can easily be applied to other blood vessels. As an initial step in any procedure relating to blood vessels (e.g. aneurisms).
The hypothesis and approach were supported by the award of a 50% EU grant. We used an approach based on Crisp DM, refined based on our experiencea nd developed our own high-quality ground-truth. We have a team dedicated to creation of annotated images, directed by a leading cardiologist. Graylight Imaging want to have developed our own deep learning architectures.
Deep learning based algorithms were selected and trained using our ground-truth data. The algorithm has been validated by leading medical centres in cardiology from France and Poland.
- DICE over 0.9.
- The work is still in progress, we are adding another 250 cases over the next months to validate and refine our algorithm further.
Single scan calcium
score evaluation
Calcium score
In this project, we propose to make use of latest achievements in machine learning technologies to build products enabling for: automatic calculation of coronary artery calcium scoring (CAC) using coronary angio-CT scans only – leading to change of current medical practise by opting out of non-contrast CT scans, with consequential impacts on time, cost, and the patient’s radiation exposure.
Complete automation and standardization of current method of coronary artery calcium scoring (CAC) assessment on non-contrast CT scans smf omprove risk prediction based on complex plaque analysis.
Our goal is to provide clinicians with advanced tools enabling for better clinical decision making and risk prediction. Based on precise 3D CT reconstruction of coronary arteries our solution allows for improved non-invasive assessment of coronary artery disease.
It can be used on chest CT to evaluate calcifications on ECG non-gated scans.
The hypothesis and approach were supported by the award of a 50% EU grant. We used an approach based on Crisp DM, refined based on our experience. We developed our own high-quality ground-truth. We have a team dedicated to creation of annotated images, directed by a leading cardiologist.
We have developed our own deep learning architectures. Deep learning based algorithms were selected and trained using our ground-truth data. The algorithm has been validated by leading medical centres in cardiology from France and Poland.
Initial training in 100 cases has demonstrated the feasibility of the approach. Plans for the next year are to increase to 1000 cases with broader heterogeneity of patient scenarios.