Automatic, precise segmentation of the coronary arteries
Automatic assessment of the CAC in CT
The system for the automatic assessment of the Coronary Artery Calcium score (i.e. calcification index) in computed tomography images of the heart has become the starting point for creating technology for automated, precise segmentation of coronary arteries. We deal with segmenting anatomical structures in cardiology and based of our experience, segmentation of the coronary arteries is the most labor-intensive.
These difficulties arise from: the complicated shape of coronary arteries; variability of coronary arteries in patients – especially when anomalies are present; small vessel diameter considering a spatial resolution of the scan; blurring and noise of the images resulting mainly from heart movements during cardiac cycle or image acquisition and reconstruction parameters used by different vendors.
Despite the challenge of segmentation of coronary arteries, Graylight Imaging specialists have created machine learning algorithms whose work is similar to that of human experts.
Coronary Artery Calcium Score Project
The need for a very precise segmentation of coronary arteries arose as a result of the project on determining the Coronary Artery Calcium score (co-financed by the European Union Program – Operational Program Intelligent Development, Sub-measure 1.1.1). The assumptions of this project include the creation of two machine learning-based models.
The first model is based on a non-contrast computed tomography series of the heart acquired with ECG-gating (i.e. calcium score series). In this case, all voxels in the pericardial sac with a radiological density above 130 Hounsfield units (HU) are automatically marked. Then, the operator manually assigns the calcifications to the specific part of the coronary arteries tree: main trunk of left coronary artery (LM), left anterior descending artery (LAD), left circumflex artery (LCX), and right coronary artery (RCA). That is the stage where there is a risk of human error. An operator might incorrectly mark some areas: calcifications within aorta, aortic valve, mitral valve, pericardium, metal implants or image artifacts. Some areas within the coronary arteries are mistakenly omitted. These errors result, among other things, from the high workload of doctors and too little time to assess this parameter.
Determining the location of the heart
Automating this procedure with machine learning algorithms requires determining the location of the heart (pericardial sac) and coronary arteries, both on non-contrast and contrast-enhanced computed tomography scans
The second model calculates the Coronary Artery Calcium score on a series of contrast enhanced computed tomography images (coronary angio-CT). The examination is performed after the intravenous administration of a contrast agent, which facilitates the reconstruction and analysis of coronary arteries. A natural obstacle in extracting the Coronary Artery Calcium score from contrast enhanced images is that the original Agatston’s procedure requires the determination of voxels with a radiological density above specific threshold of 130 HU. At the same time, the values of the contrasted lumen of the coronary arteries are many times greater. In the contrast enhanced series, however, the vessels themselves are clearly visible, and it is possible to assess their patency, and to determine the degree of stenoses based on precise geometry. It is possible to determine the location of individual calcified and non-calcified plaques on the segments of the vascular tree.
In both problems, the availability of an accurate segmentation model of the coronary arteries plays a key role. With such a model, you can precisely assign calcifications to specific coronary artery.
Coronary artery segmentation procedure
Creating a ground-truth set to achieve desired results involves creating a set of at least several hundred segmentations. Apart from the quantity, the major challenge is the quality and accuracy of created segmentations. From our experience in developing various 3D models, it has been the segmentation of the coronary arteries that we have found the most challenging and labor-intensive.
Thanks to the work of an interdisciplinary team combining competencies in various fields: biomedical engineering and software engineers, researchers, physicists, radiologists and cardiologists, Graylight Imaging has created a procedure for making artificial intelligence algorithms for precise, automatic segmentation of coronary arteries and calcified plaques.
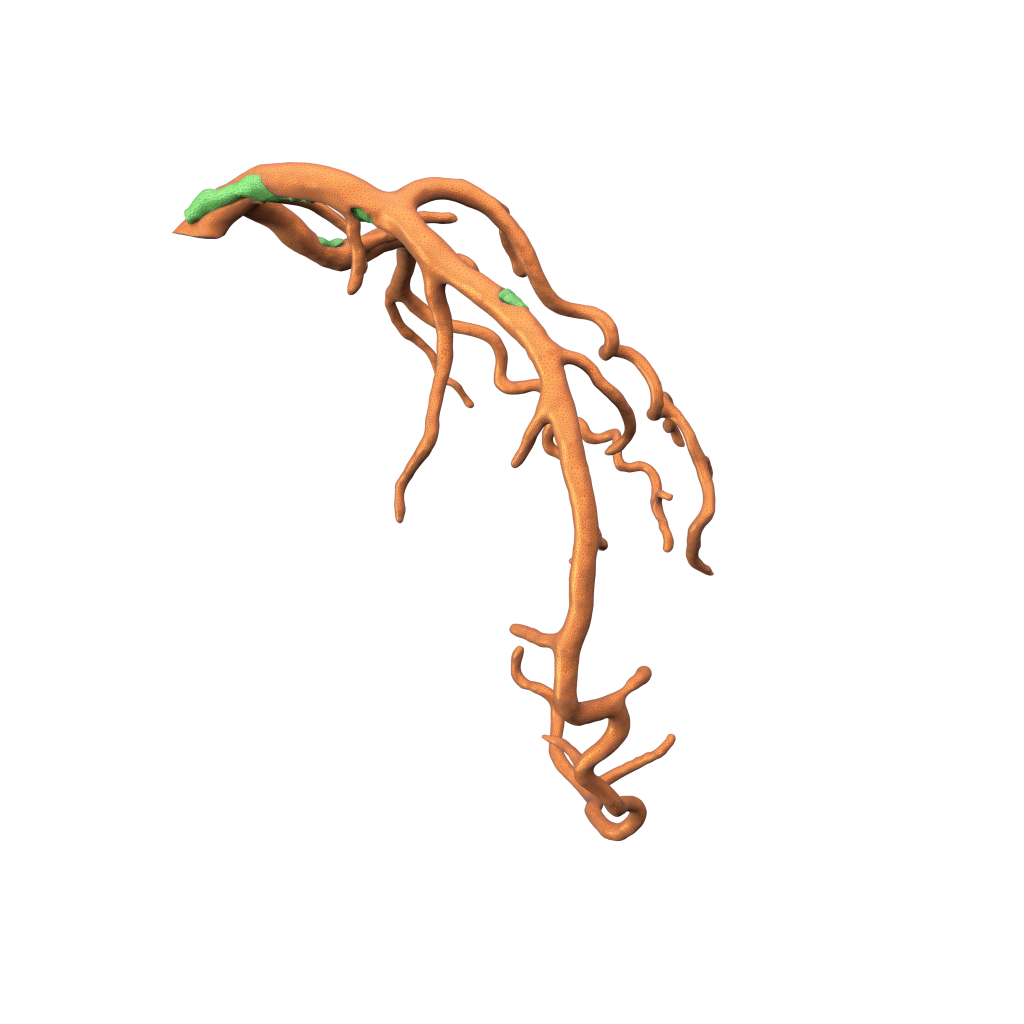
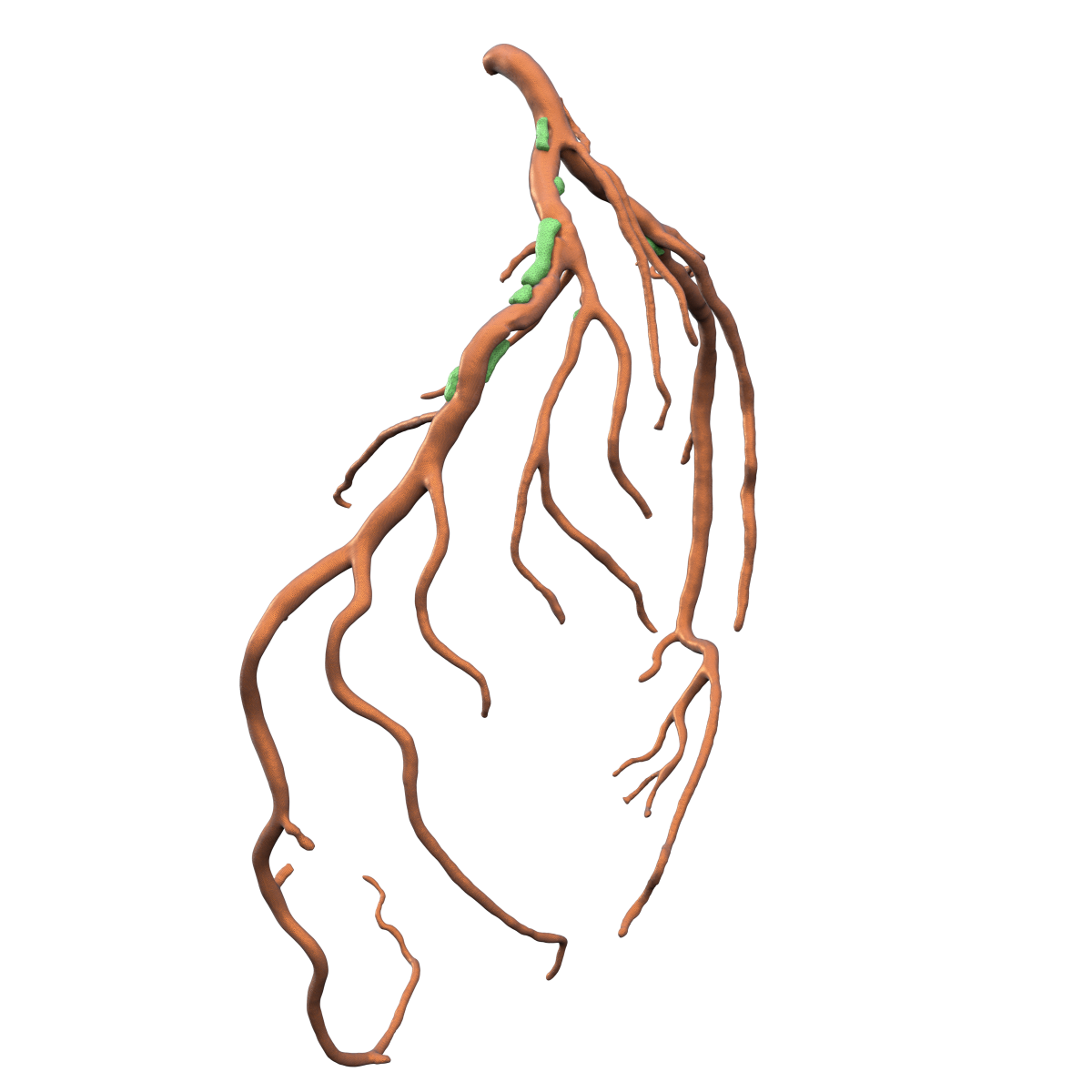
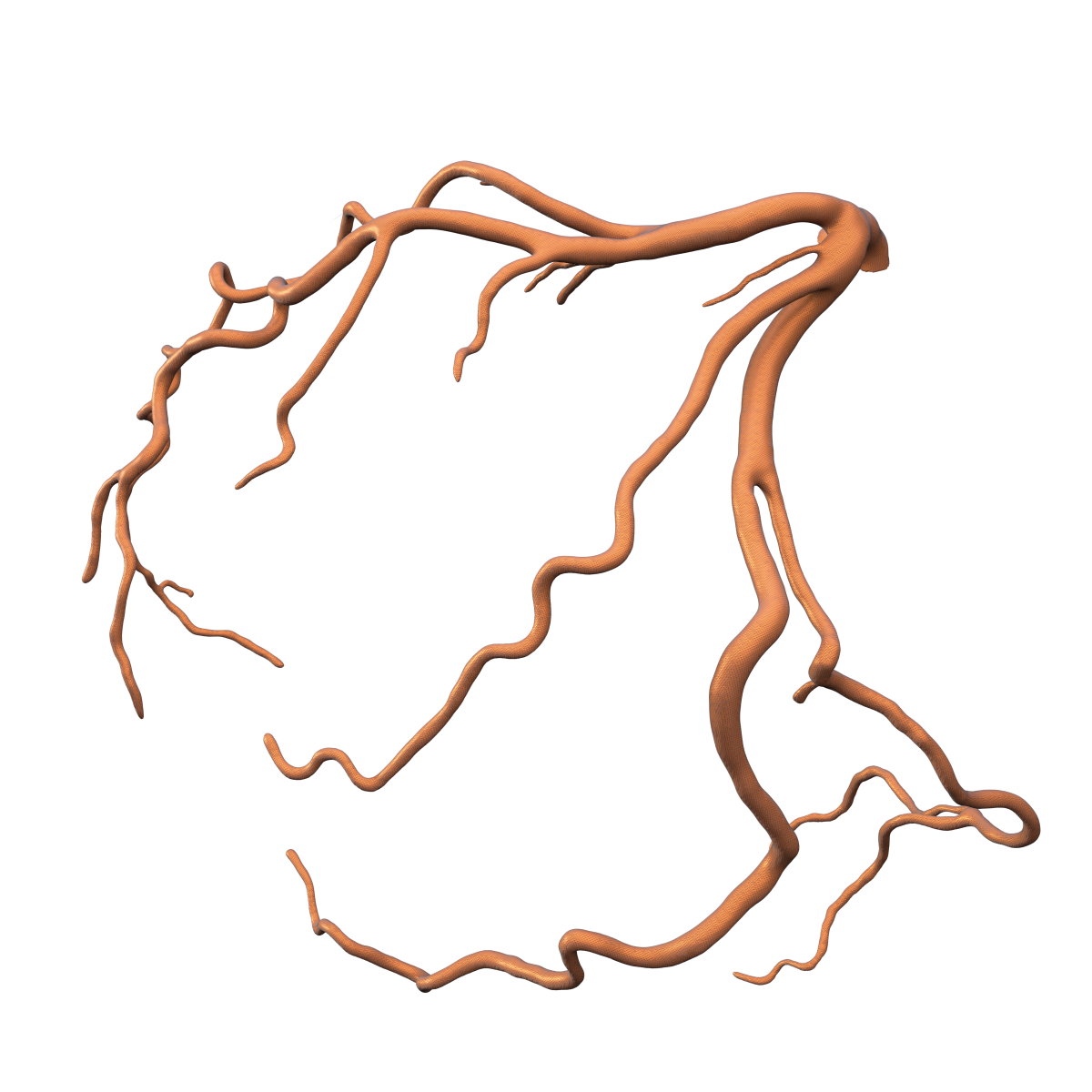