Medical image segmentation as an advancement in medical imaging
Indeed, it is hard to believe that we have been developing solutions related to medical image analysis for over 15 years. Rapid technological development has resulted in an increase in the quality of medical imaging methods and, consequently, in the diagnostic value of commonly performed imaging studies. Its natural consequence is the analysis and image segmentation in medical imaging.
Medical imaging – rapid development
It should be noted, that the first multidetector computed tomography (MDCT) scanners were introduced in the early 1990s.[1]. Close to the emergence of the DICOM 3.0 standard, still used. Let us emphasize, that the past 20 years have been a time of intense development of this medical imaging modality.
Below there are the examples of coronary artery images obtained with MDCT in 2000 and 2020, respectively:
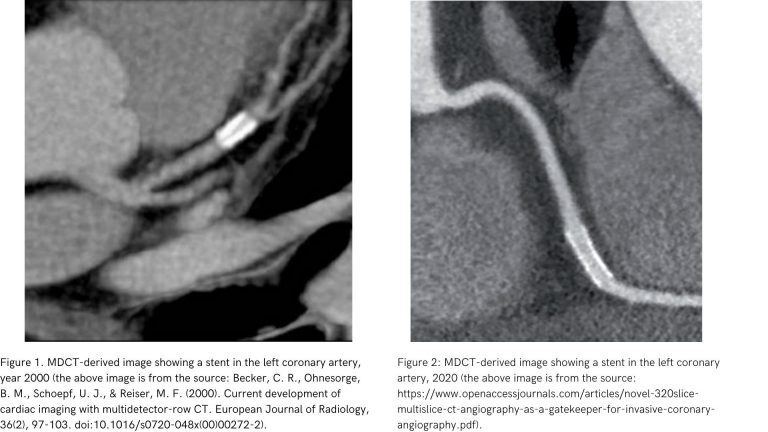
Medical image segmentation – a timely issue
A natural consequence of advances in medical imaging technology is the development of issues related to digital medical image analysis.
An issue closely related to medical image analysis, whose popularity has grown over the past 20 years, is that of medical image segmentation.
The increase in popularity of the topic of medical segmentation is well illustrated by the number of scientific papers on this subject appearing annually in the Science Direct database. This growth is due in part to the development of artificial intelligence (AI) methods.
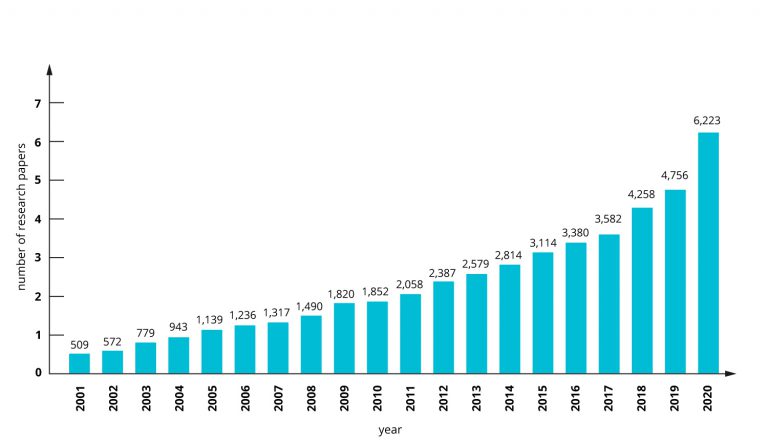
The source of the data used to create the chart comes from: https://www.sciencedirect.com. Data of March 2021.
What is medical image segmentation?
Segmentation of medical images is a way to mark/label anatomical structures: lesions or other relevant objects on medical images. The markings of relevant structures are called masks or labels.
Medical imaging techniques that allow spatial imaging enable 3D reconstruction and 3D object. This allows previously created organ segmentations to be presented in 3D.
An example segmentation of the aorta and coronary arteries in 2D and 3D
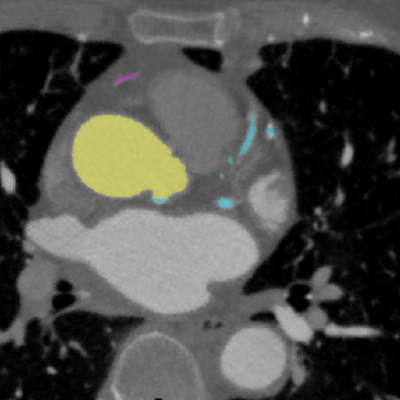
Figure 3: Masks of aorta and coronary arteries on CTA image. [source: image is from the collection of Rotterdam Coronary Artery Algorithm Evaluation Framework, http://coronary.bigr.nl; H.A. Kirişli, M. Schaap, C. Metz, A.S. Dharampal, W.B. Meijboom, S.L. Papadopoulou, A. Dedic, K. Nieman, M.A. de Graaf, M.F.L. Meijs, M.J. Cramer, A. Broersen, S. Cetin, A. Eslami, L. Flórez-Valencia, K.L. Lor, B. Matuszewski, I. Melki, B. Mohr, I. Öksüz, R. Shahzad, C. Wang, P.H. Kitslaar, G. Unal, A. Katouzian, M. Orkisz, C.M. Chen, F. Precioso, L. Najman, S. Masood, D. Ünay, L. van Vliet, R. Moreno, R. Goldenberg, E. Vuçini, G.P. Krestin, W.J. Niessen, T. van Walsum,”Standardized evaluation framework for evaluating coronary artery stenosis detection, stenosis quantification and lumen segmentation algorithms in computed tomography angiography”, Medical Image Analysis, 17(8), 859–876 (2013)].
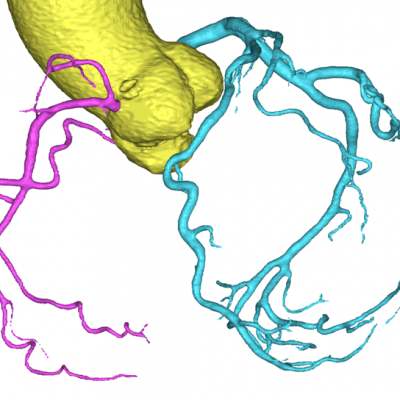
Figure 4: Three-dimensional segmentation reconstruction of the aorta and coronary arteries. [source: image is from the collection of Rotterdam Coronary Artery Algorithm Evaluation Framework, http://coronary.bigr.nl; H.A. Kirişli, M. Schaap, C. Metz, A.S. Dharampal, W.B. Meijboom, S.L. Papadopoulou, A. Dedic, K. Nieman, M.A. de Graaf, M.F.L. Meijs, M.J. Cramer, A. Broersen, S. Cetin, A. Eslami, L. Flórez-Valencia, K.L. Lor, B. Matuszewski, I. Melki, B. Mohr, I. Öksüz, R. Shahzad, C. Wang, P.H. Kitslaar, G. Unal, A. Katouzian, M. Orkisz, C.M. Chen, F. Precioso, L. Najman, S. Masood, D. Ünay, L. van Vliet, R. Moreno, R. Goldenberg, E. Vuçini, G.P. Krestin, W.J. Niessen, T. van Walsum, “Standardized evaluation framework for evaluating coronary artery stenosis detection, stenosis quantification and lumen segmentation algorithms in computed tomography angiography”, Medical Image Analysis, 17(8), 859–876 (2013)].
Summary
The segmentations we create form the essential building blocks for machine learning applications in healthcare. Segmentation is a crucial step in generating training and testing datasets. This elaborate process allows machine learning algorithms to learn and refine their capabilities. Within supervised learning methods.
The topic of segmentation is associated with the issue of preparing input data for segmentation. It will be the theme of the next article. The post will focus on the preparation of image data for segmentation. We will write about the segmentation process itself, and the methods we have developed.
Contact us if you have any questions!
See the previous post: Ground-truth in a medical machine learning project