Med-Team – the foundation of any GLI ML project
Diagnostic imaging is an invaluable source of information to doctors. It is safe to say that for many diseases diagnostics and treatment would be impossible without medical imaging.
With the advancement of technology, the number of medical imaging scans has been increasing steeply and currently medical images make up around 90% of all healthcare data [1].
Medical images are exploding in volume. This strains radiologists. However, they’re not alone. Technology can help. Data visualization tools and software that quantify changes (like volume) can support their daily work. For over 15 years, it has been our mission to contribute to solving the big problems that afflict people on a global scale. We have been developing solutions related to the analysis of medical images. One of the most essential processes of medical image analysis in ML projects is image segmentation. We have gained significant expertise in the application of deep learning for this purpose.
Methods of segmentation in ML project
There are three types of segmentation methods: manual, semi-automatic, and automatic.
Manual segmentation, a clinician’s job, involves outlining the region of interest (ROI) slice-by-slice. Alternatively, they can label individual 3D voxels of interest.
Semi-automatic methods use algorithms, thanks to which marking of a region of interest is propagated on successive slices.
When it comes to automatic segmentation, we can name two methods: learning and non-learning based. At Graylight Imaging we specialize in the first approach. We build models of deep neural networks for hands-free medical image segmentation. Deep learning as a learning-based technique uses complex neural networks to create models focused on resolving a specific imaging problem. The neural network is first trained with labelled examples using for example the U-Net architecture. Once the model has been developed it helps to significantly reduce the length of the segmentation process and does not require interaction from the medical imaging professional. Firstly, we train a neural network (like U-Net) using labelled examples. This trained model then speeds up segmentation dramatically, with no doctor needed for each step. Obviously, at this point, we must highlight one thing. Training an accurate model requires a lot of labelled data and segmentations called ground-truth. The ground-truth preparation requires a manual or semi-automatic method.
Enter Graylight Imaging Med-Team
At Graylight Imaging we have gained significant experience in preparing data for myriad ML projects. We’ve tackled challenges proving that “garbage in, garbage out” applies to ML. Our secret weapon? Additionally, we combine specialists in physics, machine learning, medicine, electro-radiology, biomedical engineering, software development and more. These unique interdisciplinary R&D teams and collaborative environment allow us to develop top notch solutions.
As I noted before, great ML projects start with great data. Our GLI Med-Team, led by Dr. Karol Miszalski-Jamka, prepares training and test data. These medical imaging specialists need patience, precision, and teamwork to create the 3D models that fuel our accurate anatomical models.
The role of Med-Team in ML projects
- collaborating on the development of tools to optimize the segmentation of anatomical structures,
- creation of 3D segmentation of cardiovascular organs (e.g. coronary arteries, heart, aorta) using available tools on the basis of CT and/or MRI images,
- analysis and verification of DICOM images,
- creation of databases together with their analysis,
- analysis of image segmentation results obtained using artificial intelligence AI (machine learning) methods.
What it takes to be part of Med-Team
Anyone who joins MedTeam must have an appropriate education which provides a basis for further study of medical and technical topics related to their work. It should provide such skills as knowledge of medical software that they use every day or the basics of anatomy without which it is difficult to move in the world of medical images.
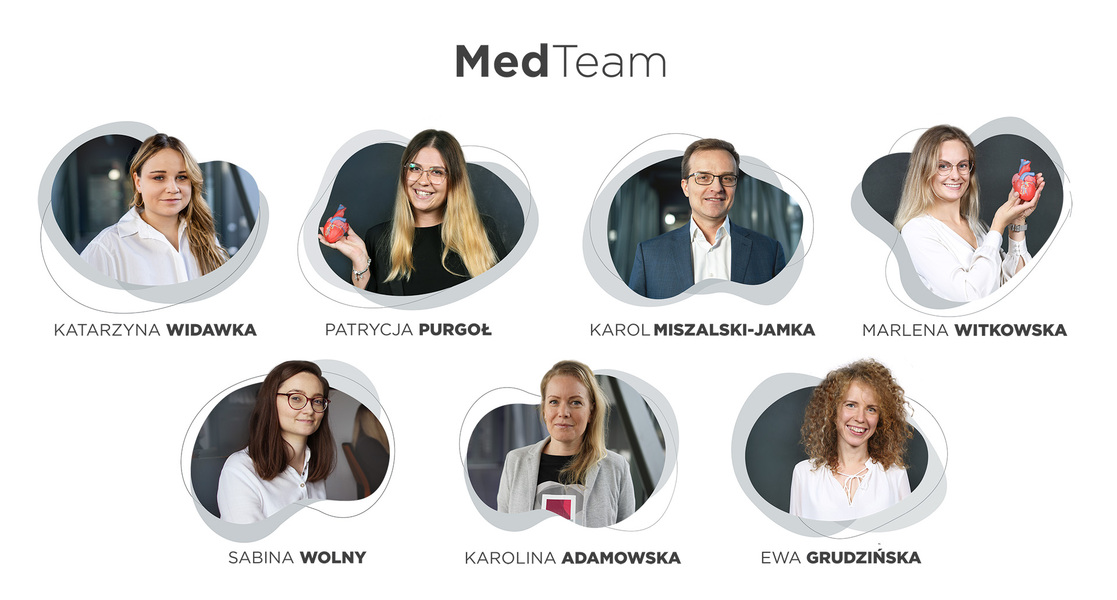
Currently the team led by Karolina Adamowska is comprised of Ewa Grudzińska, Sabina Wolny, Patrycja Purgoł, Katarzyna Widawka, Marlena Witkowska graduates of electro-radiology, biomedical engineering with specialty: biocybernetics, biomechanics holding certificates in CAD design and modeling software. Members of the team hold masters and doctoral degrees and have had previous RnD experience.
Based on medical data Med-Team create 3D models of anatomical structures using advanced, self-developed software. This means that one should have basic knowledge of medical imaging techniques (CT, MRI), be familiar with DICOM standard in medical imaging techniques, have a thorough understanding of anatomy, know 3D modelling software and have a lot of patience. Generated objects must be as accurate as possible because they are then used to train neural networks.
Additionally, their work is based on standards – those resulting from formal requirements for medical devices (operating under QMS), but also requirements resulting from the internal process approach. Working in a diverse GLI team, which is a technical-medical mix poses a challenge of itself.
Working at MedTeam is a pleasure, adventure and challenge all in one, says Marlena Witkowska, Medical Imaging Specialist.
Benefits from Med-Team work
“We work in the field of building extremely advanced technologies. Deep learning is gaining attention and widespread use right now. Everyone knows now that the quality of resulting solution is as good as data used to build it. With that in mind it is very fair to state that work done by our med team, effort and precision they put into it is critical for our overall success – quality of our resulting AI technologies starts with Med Team” Szymon Janota, CEO.
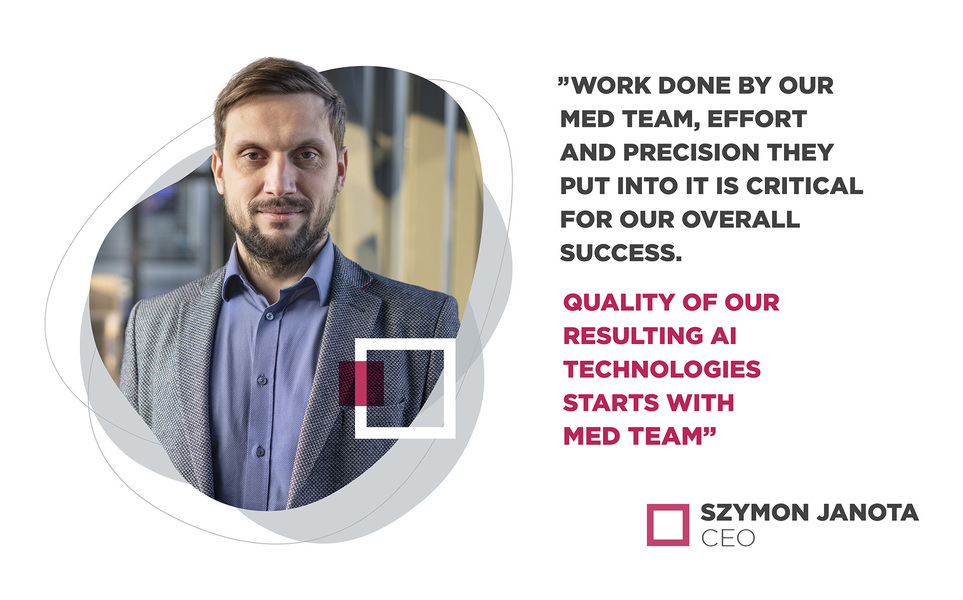
For Graylight Imaging
What needs special emphasis is the fact that without the precise and accurate work of the Med Team it would not be possible for the machine learning teams to work on ML project and for GLI to develop brand-new ground-breaking medical image analysis software.
It is the MedTeam that generates ground-truth labels for supervised learning via the challenging process of segmentation, which is costly and time-consuming but indispensable for building the first ML models.
For partners
Based on MedTeam work state-of-the-art solutions can be developed that will help improve the clinical workflow, support clinicians and the medical staff in the diagnostic process with objective and repeatable results.
For patients
As a result of MedTeam work patients gain the advantage of a faster, non-invasive diagnosis.