Mean Opinion Score as a method for the validation of R&D project results in imaging diagnostics
In one of our medical r&d projects we faced the challenge of establishing an objective measurement to gauge the quality of our results, which was crucial for clinical assessment. The effects of the software performance were extremely challenging to assess objectively. Hence it was difficult to establish an indicator that could be used for their evaluation. In this post I would like to briefly present the solution that we have implemented to deliver the validation of r&d project.
KPIs in r&d project
When we start a project, we should know its purpose and determine how we will measure the achievement of the goal. The selection of appropriate measurements also allows us to monitor the progress of the work. Moreover, it allows establish how close we have approached the designated result.
In the case of creating IT solutions, we can adopt both business indicators, such as the number of users, and technical indicators, such as the speed or efficiency. Similarly, in the case of research projects. Moreover, maybe especially in them, we make hypotheses to be accepted or rejected. On each occasion we determine the criteria with which we will evaluate them.
The criteria should be clear and objective. It means that their fulfillment cannot depend solely on the opinion of the project leaders and be somewhat “discretionary”. They must always be tailored to specific parameters.However, not all of them are easy to determine and obvious. This situation applies to all those cases where the assessor is a single user and his subjective perception is at stake. One such area is imaging diagnostics, where a great deal depends on the opinion of the radiologist. Based on their knowledge and experience, they analyze patient examinations and describe them. They have a very limited set of objective parameters at their disposal. This is reflected in the qualitative nature of their assessments.
Why the Mean Opinion Score?
The key element of our project was the segmentation of the change in the brain tissue caused by glioma. This is the most common brain tumor. The results of the work of the AI algorithm we applied, which involved deep learning, were images with a contour delineating the area identified by our model as the lesion we have been seeking. This area was then subjected to further analysis. Analysis was conducted in the system. It means its correct determination was crucial in order to obtain accurate results for the remaining analyses.
With this in mind, we faced the need to validate the obtained segmentation results. The first, somewhat obvious idea was to rely on ground-truth, specifically that part of it, which we had not used for network training. However, such a method has a fundamental downside. It allows us to evaluate the system’s operation only on tests for which we have ground-truth, and its creation requires time and involvement of internal but also external specialists e.g., radiologists. We had been looking for a solution that would enable us to prepare segmentation for any research, and at the same time we would be able to assess its quality in an objective manner. This solution turned out to be a method borrowed from the telecommunications and marketing industry, called Mean Opinion Score. Its methodology is relatively simple.
Respondents subjectively assess individual results and then their average is calculated. This average now constitutes an objective indicator, facilitating the validation of r&d project results, but not the only one. This method allows for much more.
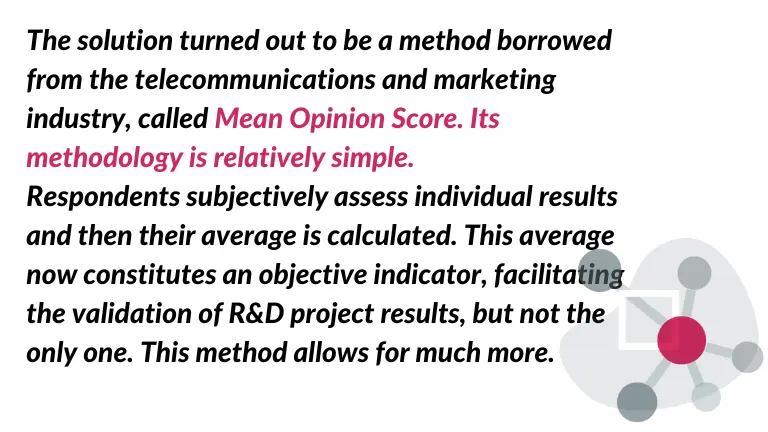
Mean Opinion Score in practice of validation in r&d project
The results were presented in the form of DICOM files of the original study. The outline of the area recognize by the system as a lesion (oedema) caused by a glioma. The participants of the study were radiologists from two different centres. They have at least 1 year of experience in imaging diagnostics. It was one of the criteria for admission. We gathered a group of 12 radiologists with 3 to 11 years of professional experience. Then we asked each of them to evaluate independently 130 segmentations on a scale of 1 to 4, where the individual numbers corresponded to the statements:
- very low-quality segmentation. I would not use the result in the diagnostic process.
- segmentation with considerable shortcomings. I would not use the result in the diagnostic process.
- segmentation with minor shortcomings. I would use the result in the diagnostic process.
- segmentation of very good quality. I would use the result in the diagnostic process.
Results of the survey
The evaluation was based on a single criterion, i.e., the use of the result in the diagnostic process, as it was the applicability and added value in patient diagnostics that was the goal of the entire r&d project. Due to the specific nature of the matter, the evaluations of respondents varied for respective segmentations. In a considerable number of cases there were always respondents who were willing to use a given segmentation, although the majority claimed otherwise. In-depth interviews with some of the respondents, carried out after the survey, revealed a high degree of subjectivity in the assessment, especially in marks 2 and 3. Many of them considered the same segmentation, seeing some deficiencies, to be good enough to be used in diagnostics, while their colleagues stated the opposite. Such subjective evaluation of studies is characteristic of imaging diagnostics, whose passage towards the objectivization of diagnoses is early days yet.
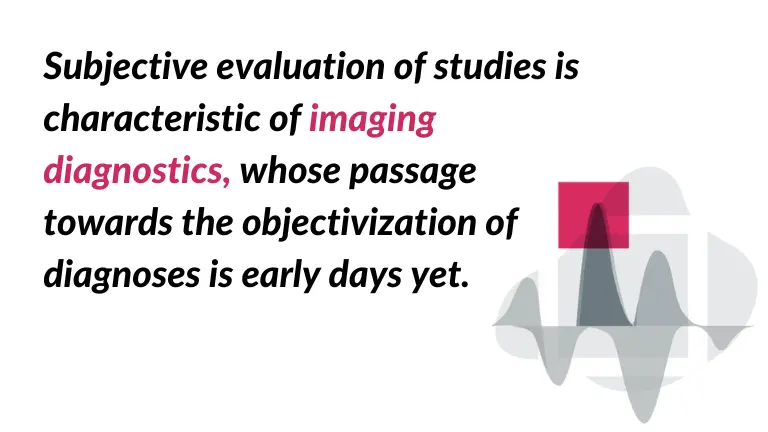
The acceptance criterion for a single segmentation, upon which we considered it to be correct, was that at least half of the respondents acknowledged that they would use it in the diagnostic process. This gave us an objective measure by which we could assess the progress and results of the project.
The Mean Opinion Score method we have used and the way of its implementation have been positively evaluated during the CE certification audit and in the clinical validation we conducted. For more details, see our recent paper [1].
References
- Fully-automated deep learning-powered system for DCE-MRI analysis of brain tumors, Artificial Intelligence in Medicine, Volume 102, January 2020, 101769 (sciencedirect.com).
Contact us if you have any questions!
Check the previous post: 6 reasons why healthcare companies should outsource R&D?