Cancer prognosis and predictive analytics in medicine
Predictive analytics in medicine is concerned with predicting the probability of a phenomenon occurring. With its help, it is possible to predict and determine the degree of risk of a disease. Thanks to this, preventive measures can be started early enough to prevent the appearance of the disease. How? This depends primarily on the type of ailment, but it may refer to lifestyle changes or more frequent medical check-ups.
Artificial intelligence is employed to predict and automate many tasks in medicine. In recent years, predictive medical algorithms used in the field of oncology have developed significantly. To be more precise, it is mainly about advances in the diagnosis, prognosis and treatment of cancer patients. These processes are constantly being improved and updated at various research centres. Thus, the application of machine learning in this field of medicine has many positive aspects. It allows us to look to a future that can protect humanity from the cancers that threaten us today. However, before this happens, algorithms must be trained on a large amount of diversified data. So that their predictions are as accurate as possible.
ML algorithms: diagnose cancer and predictive analytics to reduce cancer risk
In this paragraph, I’m going to discuss the results of research conducted by scientists who are working in research centers around the world. They checked if an ML algorithm could help diagnose cancer and predict the likelihood of cancer detection.
Firstly, let’s start with a study where ML diagnosed prostate cancer. In July 2020, researchers at the University of Pittsburgh developed a machine learning technique. Based on an artificial intelligence system, this technique achieved the highest accuracy in diagnosing prostate cancer, demonstrating an impressive 98% specificity and 98% sensitivity. A recent study employed an improved ViT (Vision Transformer) architecture, which the researchers named ViT-Patch. They validated this architecture on a publicly available dataset. The experiments showed that ViT-Patch is effective in both detecting malignant tumors and locating them.
Secondly, another study used ML to classify data relevant to breast cancer which is a method of prediction. Different classification techniques were tested and applied to certain subsets of traits. Support vector machine classifier models have shown maximum overall accuracy in the diagnosis of breast cancer. It’s worth highlighting classification algorithms use stored historical data to learn and predict new input categories, benign and malignant tumors. According to this study, a random model showed the highest accuracy of 96% in detecting cancer. This study served as the basis for a thorough understanding of the model and the implementation of the suggested prediction breast cancer AI system.
AI detect cancer risk factors
Researchers at the University of South Australia used machine learning algorithms to analyze cancer risk and develop predictive analytics in medicine. They were able to identify more than 80 biomarkers indicative of chronic kidney disease. The main factor in the study was the high level of protein found in the urine. Responsible for the most common kidney diseases. Other factors include high blood cystatin C values, urine creatinine and low serum total protein. All of this is associated with a high risk of cancer.
The results that the researchers have been able to achieve through the use of machine learning are satisfactory. The analysis of a large number of functions and the identification of hidden risk factors would not be possible without the support of artificial intelligence. The conclusion of this analysis is that we need more research to identify people at increased risk of cancer. According to this principle, prevention is better than cure. That’s why predictive analytics in medicine can help us with this.
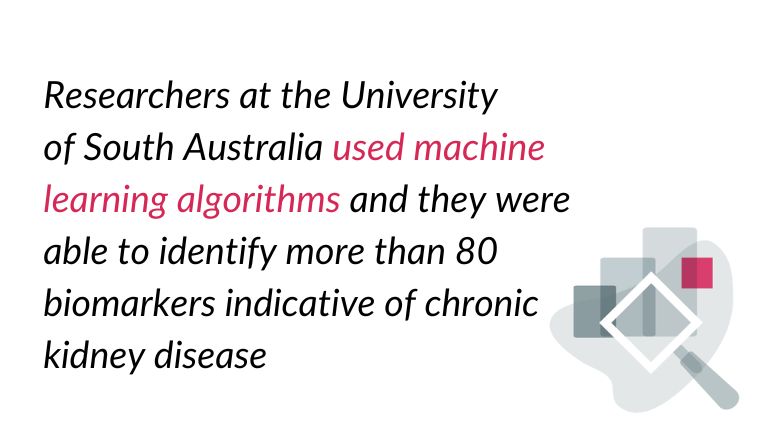
Radiomics as a part of deep learning techniques
Radiomics is one of the deep learning techniques. It is applied to medical images to obtain a huge amount of data invisible to the human eye. Can identify patterns and features related to, for example, cancer. In the medical field, radiomics is the study of these features, and there is growing interest in combining them with clinical genomic data. Radiomic techniques are used to provide information and models that predict the efficacy of treatments or adverse reactions occurring in cancer therapy.
Radiomics is particularly useful in two different types of cancer, i.e.:
- lung cancer; PET/CT in lung cancer analysis assisted with radiomic-based features,
- brain cancer; deep learning using radiomic features of the brain MRI can distinguish gliomas from brain metastases.
Limitation the use of AI in cancer detection
Based on the above examples, we can observe the growing popularity of the use of artificial intelligence for cancer detection purposes. Unfortunately, this is associated with certain risks and even limitations in its use. The biggest problem may be the problem of the so-called “black box”. While AI algorithms are effective at identifying certain patterns in data, it is difficult to understand how they arrive at certain conclusions. The lack of transparency makes it difficult to trust the predictions of artificial intelligence, especially in such a sensitive topic of cancer diagnosis.
Whereas there may be more limitations, it is worth adding another one here, which is, in my opinion, as important as the first one. Well, the potential bias of the data used to train the algorithm. Diversity is very important because different ethnic groups have different genetic predispositions. And this can lead to inaccurate results for particular groups of people.
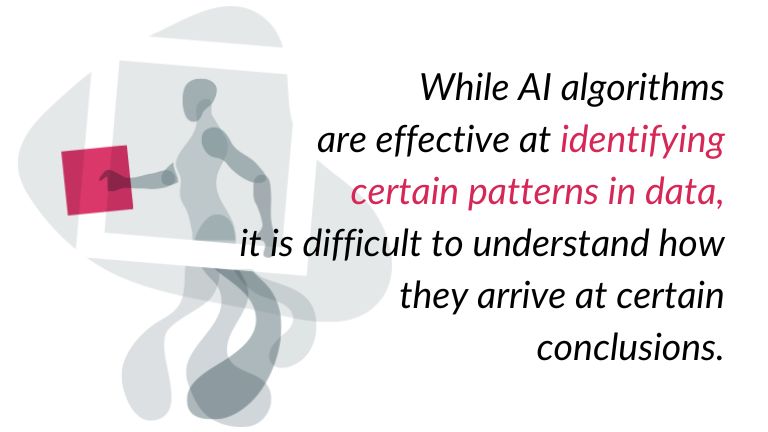
Summarize: What is the impact of AI cancer predictive algorithms in healthcare?
In brief, it is worth noting that artificial intelligence and machine learning have a huge impact on healthcare. They require a lot of research and work on training precise models to predict the topic discussed here in the field of cancer detection. The potential is enormous and has applications in almost every aspect of cancer research, including diagnosis, prognosis, and treatment. If you would like to know more about this topic in general, we recommend you read our latest post about predictive analysis algorithms in cardiovascular diseases.
As we all know cancer prevention is important, which is why the development of early detection AI algorithms, and prognosis is crucial for the oncology field. As I have shown, machine learning (ML), deep machine learning (DL), and artificial intelligence (AI) methods are and will continue to be developed. Their development aims to create models that will result in more effective cancer treatments in the future.